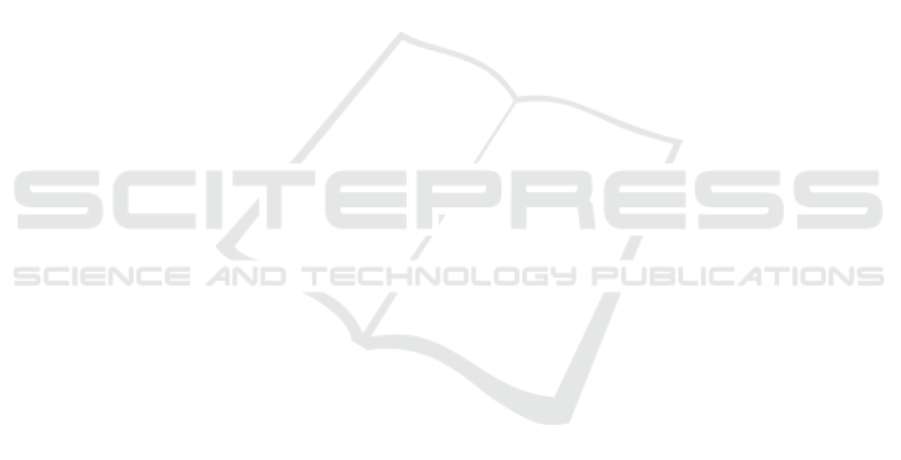
quires only 2 trials per class of training data to model
the AR models, hence only a few seconds of training
are actually required for a new BCI user. From a pre-
liminary analysis we have carried out, we identified
that increasing the number of training trials for the
AR-MM framework did not show statistical improve-
ments in performance. The transferability of train-
ing data in the AR-MM probabilistic approach from
one subject to another will also be addressed in future
work.
5 CONCLUSIONS
A novel autoregressive multiple model (AR-MM)
probabilistic framework for the detection of SSVEPs
for BCIs was presented in this paper. Through this
work we have shown that the univariate AR-MM
probabilistic approach can yield a significant im-
provement in performance over PSDA, a standard
single-channel SSVEP detection method and is only
2.29 % and 3.73 % lower in classification perfor-
mance compared to CCA and FBCCA, respectively,
two standard multichannel SSVEP detection meth-
ods. The proposed framework also provides a mea-
sure of probability for each SSVEP class, which can
be used as a measure of certainty in the decision mak-
ing process.
ACKNOWLEDGEMENTS
This work was partially supported by the project
BrainApp, financed by the Malta Council for Science
& Technology through FUSION: The R&I Technol-
ogy Development Programme 2016.
REFERENCES
Babiloni, F., Cincotti, F., Babiloni, C., Carducci, F., Mat-
tia, D., Astolfi, L., Basilisco, A., Rossini, P. M., Ding,
L., Ni, Y., Cheng, J., Christine, K., Sweeney, J., and
He, B. (2005). Estimation of the cortical functional
connectivity with the multimodal integration of high-
resolution EEG and fMRI data by directed transfer
function. Neuroimage, 24(1):118–131.
Bin, G., Gao, X., Yan, Z., Hong, B., and Gao, S.
(2009). An online multi-channel SSVEP-based brain-
computer interface using a canonical correlation anal-
ysis method. J. Neural Eng., 6(4):046002.
Cerutti, S., Chiarenza, G., Liberati, D., Mascellani, P., and
Pavesi, G. (1988). A Parametric Method of Identifica-
tion. IEEE Trans. Biomed. Eng., 35(9):701–711.
Chen, L. L., Madhavan, R., Rapoport, B. I., and Ander-
son, W. S. (2013). Real-time brain oscillation detec-
tion and phase-locked stimulation using autoregres-
sive spectral estimation and time-series forward pre-
diction. IEEE Trans. Biomed. Eng., 60(3):753–762.
Chen, X., Wang, Y., Gao, S., Jung, T.-P., and Gao,
X. (2015). Filter bank canonical correlation
analysis for implementing a high-speed SSVEP-
based brain–computer interface. J. Neural Eng.,
12(4):46008.
Fabri, S., Camilleri, K., and Cassar, T. (2011). Paramet-
ric Modelling of EEG Data for the Identification of
Mental Tasks. In Biomed. Enigeering Trends Electron.
Commun. Softw., pages 367–386.
Fabri, S. G. and Kadirkamanathan, V. (2001). Functional
Adaptive Control : an Intelligent Systems Approach.
Springer London.
Friman, O., Volosyak, I., and Gr
¨
aser, A. (2007). Mul-
tiple channel detection of steady-state visual evoked
potentials for brain-computer interfaces. IEEE Trans.
Biomed. Eng., 54(4):742–750.
Galka, A., Wong, K. K. F., and Ozaki, T. (2010). Modeling
Phase Transitions in the Brain. Model. Phase Transi-
tions Brain, pages 27–53.
Ghahramani, Z. (2001). An Introduction to Hidden Markov
Models and Bayesian Networks. J. Pattern Recognit.
Artif. Intell., 15(1):9–42.
Ghahramani, Z. and Hinton, G. E. (1998). Switching State-
Space Models. Dep. Comput. Sci. Univ. Toronto,
pages 1–28.
Guger, C., Allison, B. Z., Großwindhager, B., Pr
¨
uckl, R.,
Hinterm
¨
uller, C., Kapeller, C., Bruckner, M., Krausz,
G., and Edlinger, G. (2012). How many people could
use an SSVEP BCI? Front. Neurosci., 6(169):1–6.
Herrmann, C. S. (2001). Human EEG responses to 1-100
Hz flicker: Resonance phenomena in visual cortex
and their potential correlation to cognitive phenom-
ena. Exp. Brain Res., 137(3-4):346–353.
Krusienski, D. J., McFarland, D. J., and Wolpaw, J. R.
(2006). An evaluation of autoregressive spectral es-
timation model order for brain-computer interface ap-
plications. Conf. Proc. IEEE Eng. Med. Biol. Soc.,
1:1323–6.
Liehr, S., Pawelzik, K., Kohlmorgen, J., and M
¨
uller, K. R.
(1999). Hidden Markov mixtures of experts with an
application to EEG recordings from sleep. Theory
Biosci., 118(3-4):246–260.
Lin, Z., Zhang, C., Wu, W., and Gao, X. (2007). Frequency
recognition based on canonical correlation analysis
for SSVEP-Based BCIs. IEEE Trans. Biomed. Eng.,
54(6):1172–1176.
Miran, S., Akram, S., Sheikhattar, A., Simon, J. Z., Zhang,
T., and Babadi, B. (2018). Real-time tracking of se-
lective auditory attention from M/EEG: A Bayesian
filtering approach. Front. Neurosci., 12(MAY):1–20.
M
¨
uller-Putz, G. R., Eder, E., Wriessnegger, S. C., and
Pfurtscheller, G. (2008). Comparison of DFT and
lock-in amplifier features and search for optimal elec-
trode positions in SSVEP-based BCI. J. Neurosci.
Methods, 168(1):174–181.
An Autoregressive Multiple Model Probabilistic Framework for the Detection of SSVEPs in Brain-Computer Interfaces
77