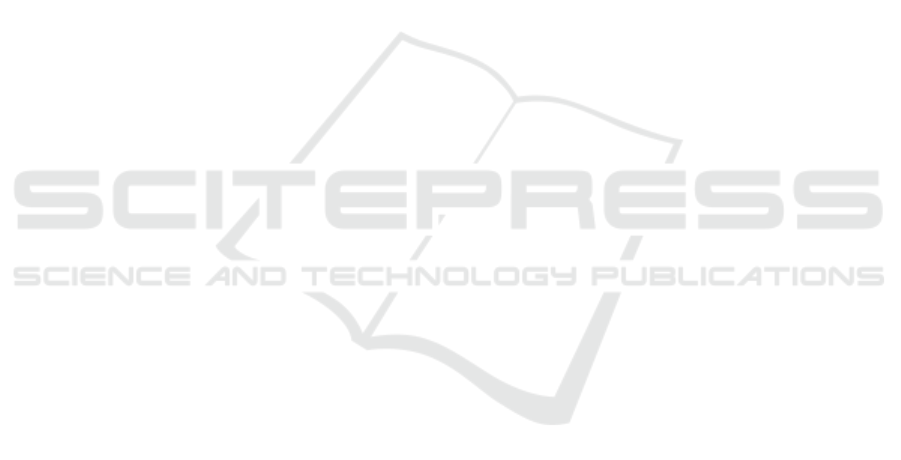
5 CONCLUSIONS AND FUTURE
WORK
In this paper, we proposed a novel model for multi-
texture synthesis. We showed it ensures full dataset
coverage and can detect textures on images in the un-
supervised setting. We provided a way to learn a man-
ifold of training textures even from a collection of raw
high-resolution photos. We also demonstrated that the
proposed model applies to the real-world 3D texture
synthesis problem: porous media generation. Our
model outperforms the baseline by better reproduc-
ing physical properties of real data. In future work,
we want to study the texture detection ability of our
model for improving segmentation in an unsupervised
way and seek for its new applications.
ACKNOWLEDGEMENTS
Aibek Alanov, Max Kochurov, Dmitry Vetrov were
supported by Samsung Research, Samsung Electron-
ics. The work of Dmitry Vetrov was supported by the
Russian Science Foundation grant no.17-71-20072.
The work of E. Burnaev and D. Volkhonskiy was sup-
ported by the MES of RF, grant No. 14.615.21.0004,
grant code: RFMEFI61518X0004. The authors E.
Burnaev and D. Volkhonskiy acknowledge the usage
of the Skoltech CDISE HPC cluster Zhores for ob-
taining some results presented in this paper.
REFERENCES
Bergmann, U., Jetchev, N., and Vollgraf, R. (2017). Learn-
ing texture manifolds with the periodic spatial gan.
ICML.
Cimpoi, M., Maji, S., Kokkinos, I., Mohamed, S., and
Vedaldi, A. (2014). Describing textures in the wild.
In Proc. IEEE CVPR, pages 3606–3613, Washington,
DC, USA. IEEE Computer Society.
Frigo, O., Sabater, N., Delon, J., and Hellier, P. (2016). Split
and match: Example-based adaptive patch sampling
for unsupervised style transfer. In Proc. IEEE CVPR,
pages 553–561.
Gatys, L., Ecker, A. S., and Bethge, M. (2015). Texture syn-
thesis using convolutional neural networks. In NIPS,
pages 262–270.
Gatys, L. A., Bethge, M., Hertzmann, A., and Shechtman,
E. (2016a). Preserving color in neural artistic style
transfer. arXiv preprint arXiv:1606.05897.
Gatys, L. A., Ecker, A. S., and Bethge, M. (2016b). Image
style transfer using convolutional neural networks. In
Proc. IEEE CVPR, pages 2414–2423.
Goodfellow, I., Pouget-Abadie, J., Mirza, M., Xu, B.,
Warde-Farley, D., Ozair, S., Courville, A., and Ben-
gio, Y. (2014). Generative adversarial nets. In NIPS,
pages 2672–2680.
Jetchev, N., Bergmann, U., and Vollgraf, R. (2016). Texture
synthesis with spatial generative adversarial networks.
arXiv preprint arXiv:1611.08207.
Johnson, J., Alahi, A., and Fei-Fei, L. (2016). Perceptual
losses for real-time style transfer and super-resolution.
In ECCV, pages 694–711. Springer.
Kingma, D. P. and Ba, J. (2015). Adam: A method for
stochastic optimization. In International Conference
on Learning Representations (ICLR).
Li, C. and Wand, M. (2016). Combining markov random
fields and convolutional neural networks for image
synthesis. In Proceedings of the IEEE Conference
on Computer Vision and Pattern Recognition, pages
2479–2486.
Li, Y., Fang, C., Yang, J., Wang, Z., Lu, X., and Yang,
M.-H. (2017). Diversified texture synthesis with feed-
forward networks. In Proc. CVPR.
Miyato, T., Kataoka, T., Koyama, M., and Yoshida, Y.
(2018). Spectral normalization for generative adver-
sarial networks. ICLR.
Mosser, L., Dubrule, O., and Blunt, M. J. (2017). Re-
construction of three-dimensional porous media using
generative adversarial neural networks. Physical Re-
view E, 96(4):043309.
Szegedy, C., Vanhoucke, V., Ioffe, S., Shlens, J., and Wojna,
Z. (2016). Rethinking the inception architecture for
computer vision. In 2016 IEEE Conference on Com-
puter Vision and Pattern Recognition, CVPR 2016,
Las Vegas, NV, USA, June 27-30, 2016, pages 2818–
2826.
Ulyanov, D., Lebedev, V., Vedaldi, A., and Lempitsky, V. S.
(2016). Texture networks: Feed-forward synthesis of
textures and stylized images. In ICML, pages 1349–
1357.
Ulyanov, D., Vedaldi, A., and Lempitsky, V. Instance nor-
malization: the missing ingredient for fast stylization.
corr abs/1607.0 (2016).
Ulyanov, D., Vedaldi, A., and Lempitsky, V. (2017). Im-
proved texture networks: Maximizing quality and di-
versity in feed-forward stylization and texture synthe-
sis. In Proceedings of the IEEE Conference on Com-
puter Vision and Pattern Recognition, pages 6924–
6932.
Volkhonskiy, D., Muravleva, E., Sudakov, O., Orlov, D.,
Belozerov, B., Burnaev, E., and Koroteev, D. (2019).
Reconstruction of 3d porous media from 2d slices.
arXiv preprint arXiv:1901.10233.
Xian, W., Sangkloy, P., Agrawal, V., Raj, A., Lu, J., Fang,
C., Yu, F., and Hays, J. (2018). Texturegan: Con-
trolling deep image synthesis with texture patches. In
Proceedings of the IEEE Conference on Computer Vi-
sion and Pattern Recognition, pages 8456–8465.
Zhou, Y., Zhu, Z., Bai, X., Lischinski, D., Cohen-Or,
D., and Huang, H. (2018). Non-stationary texture
synthesis by adversarial expansion. arXiv preprint
arXiv:1805.04487.
User-controllable Multi-texture Synthesis with Generative Adversarial Networks
221