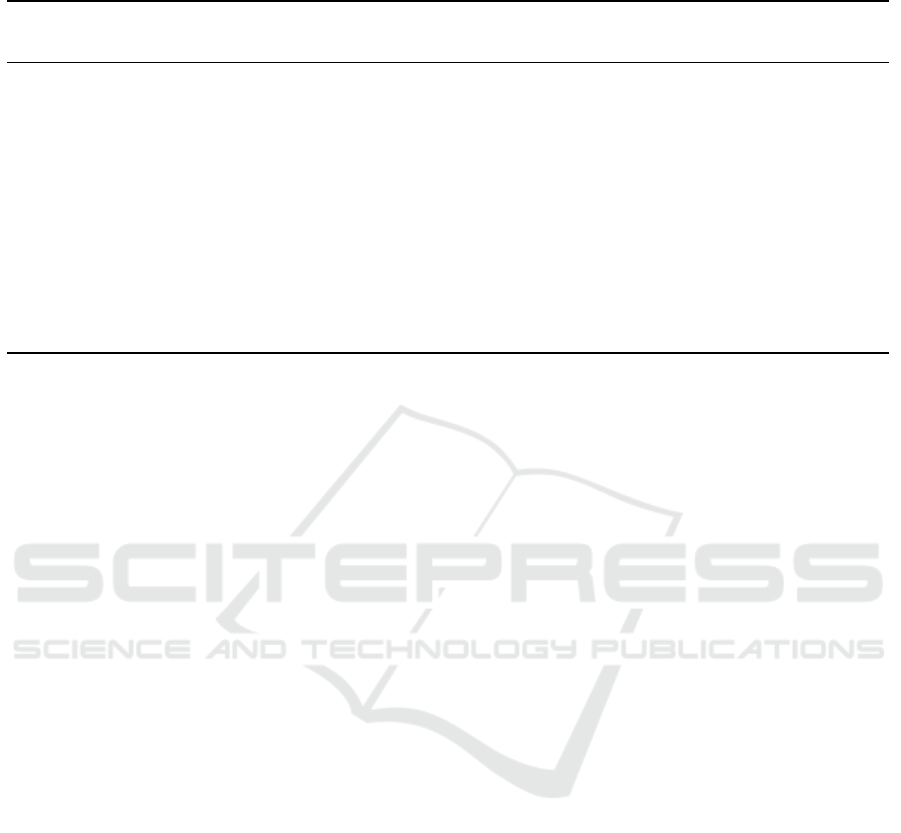
Table 3: Performance of state of the art model (Parmar and Morris, 2017), (Parmar and Morris, 2018) and our Siamese
approach.
Diving Gym Vault Ski Snowboard
Sync Dive
3m
Sync Dive
10m
Average
Single-action
C3D-SVR
(Parmar and Morris, 2017)
0.79 0.68 0.52 0.4 0.59 0.91 0.69
Single-action
C3D-LSTM
(Parmar and Morris, 2018)
0.6 0.56 0.46 0.5 0.79 0.69 0.62
Finetuned All-action
C3D-LSTM
(Parmar and Morris, 2018)
0.74 0.59 0.6 0.44 0.74 0.81 0.65
Finetuned
Siamese Network
trained with MSE (ours)
0.69 0.72 0.65 0.55 0.91 0.86 0.73
REFERENCES
Bertasius, G., Stella, X. Y., Park, H. S., and Shi, J. (2016).
Am i a baller? basketball skill assessment using first-
person cameras. CoRR.
Bromley, J., Guyon, I., LeCun, Y., Säckinger, E., and Shah,
R. (1993). Signature verification using a siamese time
delay neural network. In NIPS.
Burns, A.-M., Kulpa, R., Durny, A., Spanlang, B., Slater,
M., and Multon, F. (2011). Using virtual humans and
computer animations to learn complex motor skills: a
case study in karate. In BIO Web of Conferences.
Chen, W.-Y., Liu, Y.-C., Kira, Z., Wang, Y.-C. F., and
Huang, J.-B. (2019). A closer look at few-shot classi-
fication. ICLR.
Chung, D., Tahboub, K., and Delp, E. J. (2017). A two
stream siamese convolutional neural network for per-
son re-identification. In Proceedings of the IEEE
International Conference on Computer Vision, pages
1983–1991.
Doughty, H., Damen, D., and Mayol-Cuevas, W. W. (2018).
Who’s better? who’s best? pairwise deep ranking for
skill determination. 2018 IEEE/CVF Conference on
Computer Vision and Pattern Recognition.
Doughty, H., Mayol-Cuevas, W. W., and Damen, D. (2019).
The pros and cons: Rank-aware temporal attention for
skill determination in long videos. Computer Vision
and Pattern Recognition.
Fawaz, H., Forestier, G., Weber, J., Idoumghar, L., and
Muller, P.-A. (2018). Evaluating surgical skills from
kinematic data using convolutional neural networks.
Medical Image Computing and Computer-Assisted In-
tervention – MICCAI 2018, 11073.
Funke, I., Mees, S. T., Weitz, J., and Speidel, S.
(2019). Video-based surgical skill assessment using
3d-convolutional neural networks. International Jour-
nal of Computer Assisted Radiology and Surgery.
Gao, Y., Vedula, S. S., Reiley, C. E., Ahmidi, N., Varadara-
jan, B., Lin, H. C., Tao, L., Zappella, L., Béjar, B.,
Yuh, D. D., Chen, C. C. G., Vidal, R., Khudanpur, S.,
and Hager, G. D. (2014). Jhu-isi gesture and skill as-
sessment working set ( jigsaws ) : A surgical activity
dataset for human motion modeling.
Hochreiter, S. and Schmidhuber, J. (1997). Long short-term
memory. Neural Computation.
Karpathy, A., Toderici, G., Shetty, S., Leung, T., Suk-
thankar, R., and Fei-Fei, L. (2014). Large-scale
video classification with convolutional neural net-
works. 2014 IEEE Conference on Computer Vision
and Pattern Recognition.
Kim, Y. (2014). Convolutional neural networks for sentence
classification. In EMNLP.
Kingma, D. P. and Ba, J. (2015). Adam: A method for
stochastic optimization. CoRR.
Komura, T., Lam, B., Lau, R. W., and Leung, H. (2006). e-
learning martial arts. In International Conference on
Web-Based Learning.
Ledig, C., Theis, L., Huszár, F., Caballero, J., Aitken, A. P.,
Tejani, A., Totz, J., Wang, Z., and Shi, W. (2017).
Photo-realistic single image super-resolution using a
generative adversarial network. 2017 IEEE Confer-
ence on Computer Vision and Pattern Recognition
(CVPR).
Lei, Q., Du, J., Zhang, H., Ye, S., and Chen, D.-S. (2019). A
survey of vision-based human action evaluation meth-
ods. In Sensors.
Li, Z., Huang, Y., Cai, M., and Sato, Y. (2019).
Manipulation-skill assessment from videos with spa-
tial attention network. ArXiv.
Morel, M., Achard, C., Kulpa, R., and Dubuisson, S.
(2017). Automatic evaluation of sports motion: A
generic computation of spatial and temporal errors.
Image and Vision Computing.
Morel, M., Achard, C., Kulpa, R., and Dubuisson, S.
VISAPP 2020 - 15th International Conference on Computer Vision Theory and Applications
64