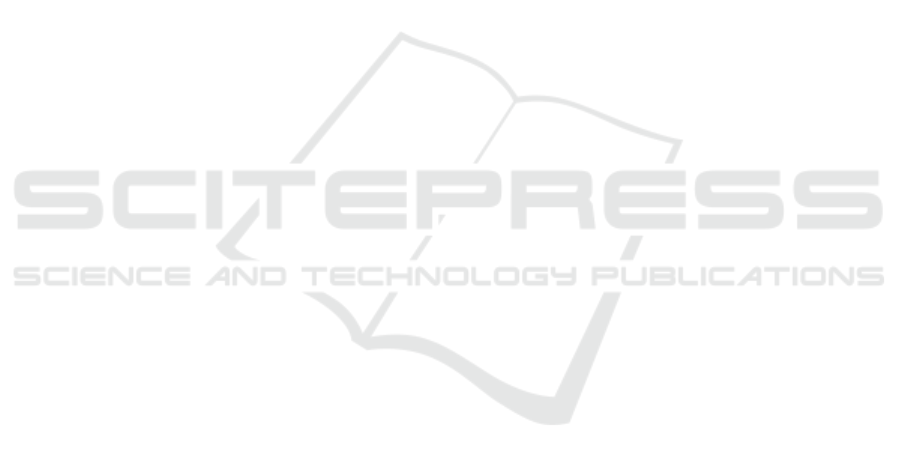
works. In 2017 IEEE Conference on Computer Vision
and Pattern Recognition (CVPR). IEEE.
Iandola, F. N., Han, S., Moskewicz, M. W., Ashraf, K.,
Dally, W. J., and Keutzer, K. (2016). Squeezenet:
Alexnet-level accuracy with 50x fewer parame-
ters and <0.5 MB model size. arXiv preprint
arXiv:1602.07360.
Jia, Y., Shelhamer, E., Donahue, J., Karayev, S., Long, J.,
Girshick, R., Guadarrama, S., and Darrell, T. (2014).
Caffe: Convolutional Architecture for Fast Feature
Embedding. arXiv preprint arXiv:1408.5093.
Kermany, D. S., Goldbaum, M., Cai, W., Valentim, C. C.,
Liang, H., Baxter, S. L., McKeown, A., Yang, G., Wu,
X., Yan, F., Dong, J., Prasadha, M. K., Pei, J., Ting,
M. Y., Zhu, J., Li, C., Hewett, S., Dong, J., Ziyar,
I., Shi, A., Zhang, R., Zheng, L., Hou, R., Shi, W.,
Fu, X., Duan, Y., Huu, V. A., Wen, C., Zhang, E. D.,
Zhang, C. L., Li, O., Wang, X., Singer, M. A., Sun, X.,
Xu, J., Tafreshi, A., Lewis, M. A., Xia, H., and Zhang,
K. (2018). Identifying Medical Diagnoses and Treat-
able Diseases by Image-Based Deep Learning. Cell,
172(5):1122–1131.e9.
Krizhevsky, A., Sutskever, I., and Hinton, G. E. (2012). Im-
ageNet Classification with Deep Convolutional Neu-
ral Networks. In Advances in Neural Information Pro-
cessing Systems 25, pages 1097–1105. Curran Asso-
ciates, Inc.
Liang, G. and Zheng, L. (2019). A transfer learning method
with deep residual network for pediatric pneumo-
nia diagnosis. Computer Methods and Programs in
Biomedicine.
Litjens, G., Kooi, T., Bejnordi, B. E., Setio, A. A. A.,
Ciompi, F., Ghafoorian, M., van der Laak, J. A., van
Ginneken, B., and S
´
anchez, C. I. (2017). A survey
on deep learning in medical image analysis. Medical
Image Analysis, 42:60–88.
Lodha, R., Kabra, S., and Pandey, R. (2013). Antibiotics for
communityacquired pneumonia in children. Cochrane
Database of Systematic Reviews.
Møller, M. F. (1993). A scaled conjugate gradient algo-
rithm for fast supervised learning. Neural Networks,
6(4):525–533.
Organization, W. H. (2001). Standardization of interpreta-
tion of chest radiographs for the diagnosis of pneumo-
nia in children. Last Accessed: 14 May 2019.
Paszke, A., Gross, S., Chintala, S., Chanan, G., Yang, E.,
DeVito, Z., Lin, Z., Desmaison, A., Antiga, L., and
Lerer, A. (2017). Automatic differentiation in Py-
Torch. In NIPS-W.
Pedregosa, F., Varoquaux, G., Gramfort, A., Michel, V.,
Thirion, B., Grisel, O., Blondel, M., Prettenhofer, P.,
Weiss, R., Dubourg, V., Vanderplas, J., Passos, A.,
Cournapeau, D., Brucher, M., Perrot, M., and Duch-
esnay, E. (2011). Scikit-learn: Machine Learning
in Python. Journal of Machine Learning Research,
12:2825–2830.
Powers, D. M. (2011). Evaluation: from precision, recall
and f-measure to roc, informedness, markedness and
correlation. Journal of Machine Learning Technolo-
gies, 2(1):37 – 63.
Qin, C., Yao, D., Shi, Y., and Song, Z. (2018). Computer-
aided detection in chest radiography based on artifi-
cial intelligence: a survey. BioMedical Engineering
OnLine, 17(1).
Rajpurkar, P., Irvin, J., Zhu, K., Yang, B., Mehta, H., Duan,
T., Ding, D., Bagul, A., Langlotz, C., Shpanskaya, K.,
et al. (2017). CheXNet: Radiologist-Level Pneumo-
nia Detection on Chest X-Rays with Deep Learning.
arXiv preprint arXiv:1711.05225.
Razzak, M. I., Naz, S., and Zaib, A. (2017). Deep Learning
for Medical Image Processing: Overview, Challenges
and the Future. In Lecture Notes in Computational Vi-
sion and Biomechanics, pages 323–350. Springer In-
ternational Publishing.
Robbins, H. and Monro, S. (1951). A Stochastic Approx-
imation Method. The Annals of Mathematical Statis-
tics, 22(3):400–407.
Shie, C.-K., Chuang, C.-H., Chou, C.-N., Wu, M.-H., and
Chang, E. Y. (2015). Transfer representation learn-
ing for medical image analysis. In 2015 37th Annual
International Conference of the IEEE Engineering in
Medicine and Biology Society (EMBC). IEEE.
Siddiqi, R. (2019). Automated pneumonia diagnosis using
a customized sequential convolutional neural network.
In Proceedings of the 2019 3rd International Confer-
ence on Deep Learning Technologies - ICDLT 2019.
ACM Press.
Simonyan, K. and Zisserman, A. (2014). Very Deep Con-
volutional Networks for Large-Scale Image Recogni-
tion. arXiv preprint arXiv:1409.1556.
Szegedy, C., Ioffe, S., Vanhoucke, V., and Alemi, A. (2017).
Inception-v4, Inception-ResNet and the Impact of
Residual Connections on Learning. In 2017 31st AAAI
Conference on Artificial Intelligence (AAAI-17).
Szegedy, C., Liu, W., Jia, Y., Sermanet, P., Reed, S.,
Anguelov, D., Erhan, D., Vanhoucke, V., and Rabi-
novich, A. (2015). Going deeper with convolutions. In
2015 IEEE Conference on Computer Vision and Pat-
tern Recognition (CVPR). IEEE.
Szegedy, C., Vanhoucke, V., Ioffe, S., Shlens, J., and Wojna,
Z. (2016). Rethinking the Inception Architecture for
Computer Vision. In 2016 IEEE Conference on Com-
puter Vision and Pattern Recognition (CVPR). IEEE.
Wang, H. and Xia, Y. (2018). ChestNet: A Deep Neural
Network for Classification of Thoracic Diseases on
Chest Radiography. arXiv preprint arXiv:1807.03058.
Wang, X., Peng, Y., Lu, L., Lu, Z., Bagheri, M., and Sum-
mers, R. M. (2017). Chestx-ray8: Hospital-scale chest
x-ray database and benchmarks on weakly-supervised
classification and localization of common thorax dis-
eases. In 2017 IEEE Conference on Computer Vision
and Pattern Recognition (CVPR), pages 3462–3471.
Yosinski, J., Clune, J., Bengio, Y., and Lipson, H. (2014).
How transferable are features in deep neural net-
works? In Advances in Neural Information Process-
ing Systems 27, pages 3320–3328. Curran Associates,
Inc.
A Deep Transfer Learning Framework for Pneumonia Detection from Chest X-ray Images
293