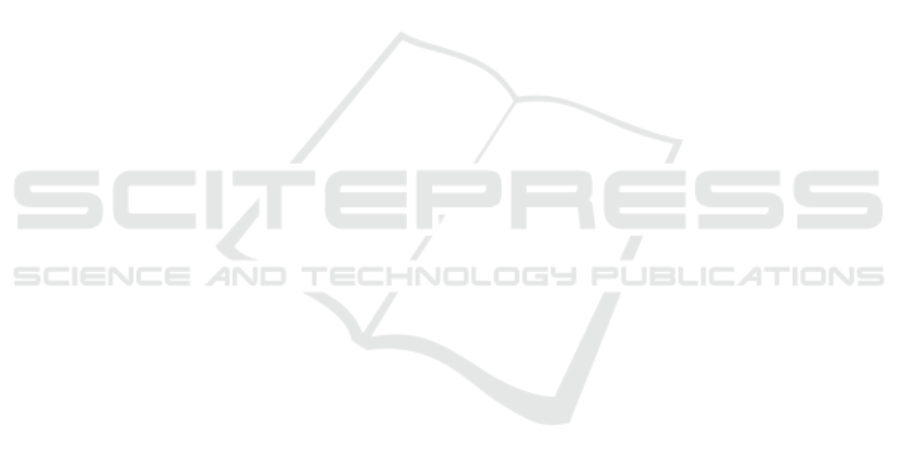
gio, Y. (2014). Generative adversarial nets. In Neural
Information Processing Systems, pages 2672–2680.
Isola, P., Zhu, J.-Y., Zhou, T., and Efros, A. A. (2017).
Image-to-image translation with conditional adversar-
ial networks. In IEEE International Conference on
Computer Vision and Pattern Recognition.
Kim, H., Handa, A., Benosman, R., Ieng, S., and Davison,
A. J. (2014). Simultaneous mosaicing and tracking
with an event camera. In British Machine Vision Con-
ference.
Kingma, D. P. and Ba, J. (2014). Adam: A method for
stochastic optimization. CoRR, abs/1412.6980.
Lagorce, X., Orchard, G., Galluppi, F., Shi, B. E., and
Benosman, R. B. (2017). Hots: a hierarchy of event-
based time-surfaces for pattern recognition. IEEE
Transactions on Pattern Analysis and Machine Intel-
ligence, 39(7):1346–1359.
Lichtsteiner, P., Posch, C., and Delbruck, T. (2006). A 128 x
128 120db 30mw asynchronous vision sensor that re-
sponds to relative intensity change. In Solid-State Cir-
cuits Conference, 2006. ISSCC 2006. Digest of Tech-
nical Papers. IEEE International, pages 2060–2069.
IEEE.
Lin, T.-Y., Maire, M., Belongie, S., Hays, J., Perona, P.,
Ramanan, D., Doll
´
ar, P., and Zitnick, C. L. (2014).
Microsoft COCO: Common objects in context. In Eu-
ropean Conference on Computer Vision. Springer.
Lungu, I.-A., Corradi, F., and Delbr
¨
uck, T. (2017). Live
demonstration: Convolutional neural network driven
by dynamic vision sensor playing roshambo. In IEEE
International Symposium on Circuits and Systems (IS-
CAS), pages 1–1. IEEE.
Maqueda, A. I., Loquercio, A., Gallego, G., Garcıa, N.,
and Scaramuzza, D. (2018). Event-based vision meets
deep learning on steering prediction for self-driving
cars. In IEEE International Conference on Computer
Vision and Pattern Recognition, pages 5419–5427.
Mirza, M. and Osindero, S. (2014). Conditional generative
adversarial nets. arXiv preprint arXiv:1411.1784.
Mitrokhin, A., Fermuller, C., Parameshwara, C., and Aloi-
monos, Y. (2018). Event-based moving object detec-
tion and tracking. arXiv preprint arXiv:1803.04523.
Munda, G., Reinbacher, C., and Pock, T. (2018). Real-time
intensity-image reconstruction for event cameras us-
ing manifold regularisation. International Journal of
Computer Vision, 126(12):1381–1393.
Pini, S., Borghi, G., Vezzani, R., and Cucchiara, R. (2019).
Video synthesis from intensity and event frames. In
International Conference on Image Analysis and Pro-
cessing, pages 313–323. Springer.
Ramesh, B., Zhang, S., Lee, Z. W., Gao, Z., Orchard, G.,
and Xiang, C. (2018). Long-term object tracking with
a moving event camera. In British Machine Vision
Conference.
Rebecq, H., Gallego, G., and Scaramuzza, D. (2016). Emvs:
Event-based multi-view stereo. In British Machine Vi-
sion Conference.
Rebecq, H., Ranftl, R., Koltun, V., and Scaramuzza, D.
(2019). Events-to-video: Bringing modern computer
vision to event cameras. In Proceedings of the IEEE
Conference on Computer Vision and Pattern Recogni-
tion, pages 3857–3866.
Redmon, J. and Farhadi, A. (2018). YOLOv3:
An incremental improvement. arXiv preprint
arXiv:1804.02767.
Reinbacher, C., Graber, G., and Pock, T. (2016). Real-time
intensity-image reconstruction for event cameras us-
ing manifold regularisation. In British Machine Vision
Conference.
Ronneberger, O., Fischer, P., and Brox, T. (2015). U-net:
Convolutional networks for biomedical image seg-
mentation. In International Conference on Medical
image computing and computer-assisted intervention,
pages 234–241. Springer.
Rota Bul
`
o, S., Porzi, L., and Kontschieder, P. (2018).
In-place activated batchnorm for memory-optimized
training of dnns. In Proceedings of the IEEE Confer-
ence on Computer Vision and Pattern Recognition.
Salimans, T., Goodfellow, I., Zaremba, W., Cheung, V.,
Radford, A., and Chen, X. (2016). Improved tech-
niques for training gans. In Neural Information Pro-
cessing Systems, pages 2234–2242.
Scheerlinck, C., Barnes, N., and Mahony, R. (2018).
Continuous-time intensity estimation using event
cameras. Asian Conf. Comput. Vis. (ACCV).
Uhrig, J., Schneider, N., Schneider, L., Franke, U., Brox,
T., and Geiger, A. (2017). Sparsity invariant cnns. In
International Conference on 3D Vision (3DV).
Wang, Z., Bovik, A. C., Sheikh, H. R., and Simoncelli, E. P.
(2004). Image quality assessment: from error visi-
bility to structural similarity. IEEE transactions on
image processing, 13(4):600–612.
Xingjian, S., Chen, Z., Wang, H., Yeung, D.-Y., Wong, W.-
K., and Woo, W.-c. (2015). Convolutional lstm net-
work: A machine learning approach for precipitation
nowcasting. In Neural Information Processing Sys-
tems, pages 802–810.
Zhang, R., Isola, P., Efros, A. A., Shechtman, E., and Wang,
O. (2018). The unreasonable effectiveness of deep
features as a perceptual metric. In CVPR.
Zhou, Y., Gallego, G., Rebecq, H., Kneip, L., Li, H., and
Scaramuzza, D. (2018). Semi-dense 3d reconstruction
with a stereo event camera. In European Conference
on Computer Vision.
Zhu, A. Z., Thakur, D.,
¨
Ozaslan, T., Pfrommer, B., Kumar,
V., and Daniilidis, K. (2018). The multivehicle stereo
event camera dataset: An event camera dataset for 3d
perception. IEEE Robotics and Automation Letters,
3(3):2032–2039.
Learn to See by Events: Color Frame Synthesis from Event and RGB Cameras
47