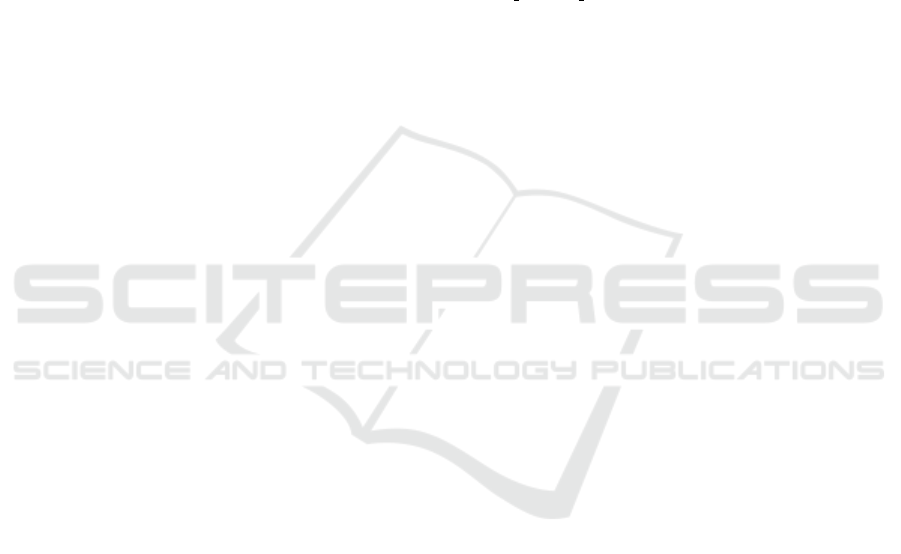
REFERENCES
Adler, N. E. and Stead, W. W. (2015). Patients in context –
EHR capture of social and behavioral determinants of
health. N Engl J Med, 372(8):698–701.
Aronson, A. R. and Lang, F.-M. (2010). An overview of
metamap: historical perspective and recent advances.
J Am Med Inform Assoc, 17(3):229–236.
Bird, S., Klein, E., and Loper, E. (2009). Natural language
processing with Python: analyzing text with the natu-
ral language toolkit. ” O’Reilly Media, Inc.”.
Forouzanfar, M. H., Afshin, A., Alexander, L. T., An-
derson, H. R., Bhutta, Z. A., Biryukov, S., Brauer,
M., Burnett, R., Cercy, K., Charlson, F. J., et al.
(2016). Global, regional, and national compara-
tive risk assessment of 79 behavioural, environmen-
tal and occupational, and metabolic risks or clusters
of risks, 1990–2015: a systematic analysis for the
global burden of disease study 2015. The Lancet,
388(10053):1659–1724.
Gheorghiu, B. and Hagens, S. (2017). Use and maturity of
electronic patient portals. In ITCH, pages 136–141.
Jakovljevic, M., Jakab, M., Gerdtham, U., McDaid, D.,
Ogura, S., Varavikova, E., Merrick, J., Adany, R.,
Okunade, A., and Getzen, T. E. (2019). Comparative
financing analysis and political economy of noncom-
municable diseases. J Med Econ, 0(0):1–6. PMID:
30913928.
Jensen, P. B., Jensen, L. J., and Brunak, S. (2012). Mining
electronic health records: towards better research ap-
plications and clinical care. Nat Rev Genet, 13(6):395.
Kiecolt-Glaser, J. K., Christian, L., Preston, H., Houts,
C. R., Malarkey, W. B., Emery, C. F., and Glaser, R.
(2010). Stress, inflammation, and yoga practice. Psy-
chosom Med, 72(2):113.
Kreimeyer, K., Foster, M., Pandey, A., Arya, N., Hal-
ford, G., Jones, S. F., Forshee, R., Walderhaug, M.,
and Botsis, T. (2017). Natural language processing
systems for capturing and standardizing unstructured
clinical information: a systematic review. J Biomed
Inform, 73:14–29.
Mafi, J. N., Gerard, M., Chimowitz, H., Anselmo, M., Del-
banco, T., and Walker, J. (2018). Patients contribut-
ing to their doctors’ notes: insights from expert inter-
views. Annals Intern Med, 168(4):302–305.
Pascoe, M. C. and Bauer, I. E. (2015). A systematic review
of randomised control trials on the effects of yoga on
stress measures and mood. J Psychiatr Res, 68:270–
282.
Pedregosa, F., Varoquaux, G., Gramfort, A., Michel, V.,
Thirion, B., Grisel, O., Blondel, M., Prettenhofer, P.,
Weiss, R., Dubourg, V., et al. (2011). Scikit-learn:
Machine learning in python. J Mach Learn Res,
12(Oct):2825–2830.
Penrod, N. M., Lynch, S., Thomas, S., Seshadri, N., and
Moore, J. H. (2019). Prevalence and characterization
of yoga mentions in the electronic health record. The
Journal of the American Board of Family Medicine,
32(6):790–800.
Savova, G. K., Masanz, J. J., Ogren, P. V., Zheng, J., Sohn,
S., Kipper-Schuler, K. C., and Chute, C. G. (2010).
Mayo clinical text analysis and knowledge extraction
system (ctakes): architecture, component evaluation
and applications. J Am Med Inform Assoc, 17(5):507–
513.
Soysal, E., Wang, J., Jiang, M., Wu, Y., Pakhomov, S., Liu,
H., and Xu, H. (2017). Clamp–a toolkit for efficiently
building customized clinical natural language process-
ing pipelines. J Am Med Inform Assoc, 25(3):331–
336.
Waters, H. and Graf, M. (2018). The cost of chronic dis-
eases in the U.S. Technical report, Milken Institute.
World Health Organization (2019). Global Health Observa-
tory (GHO) data. https://www.who.int/gho/mortality\
burden\ disease/en/. Accessed May 10, 2019.
HEALTHINF 2020 - 13th International Conference on Health Informatics
82