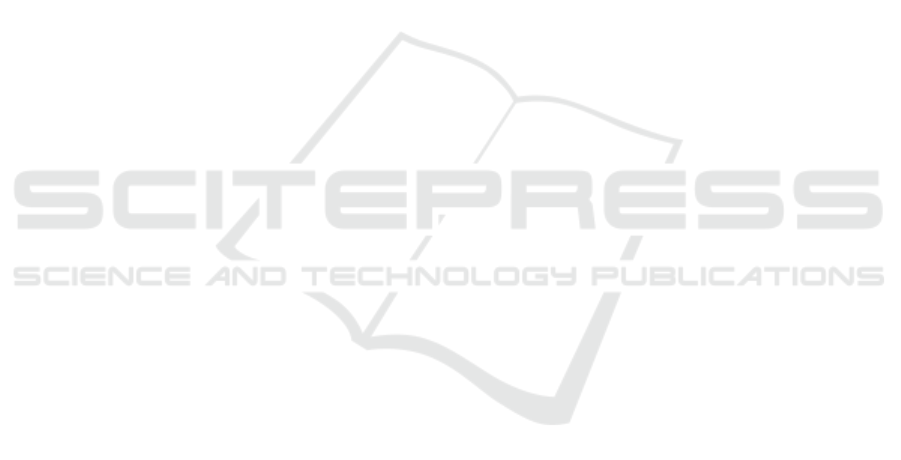
REFERENCES
Bendale, A. and Boult, T. E. (2015). Towards open world
recognition. Proceedings of the IEEE Conference
on Computer Vision and Pattern Recognition, pages
1893–1902.
Bendale, A. and Boult, T. E. (2016). Towards open set deep
networks. Proceedings of the IEEE conference on
computer vision and pattern recognition, pages 1563–
1572.
Ester, M., Kriegel, H.-P., Sander, J., and Xu, X. (1996).
A density-based algorithm for discovering clusters a
density-based algorithm for discovering clusters in
large spatial databases with noise. In Proceedings of
the Second International Conference on Knowledge
Discovery and Data Mining, KDD’96, pages 226–
231. AAAI Press.
Everingham, M., Gool, L., Williams, C. K., Winn, J., and
Zisserman, A. (2010). The pascal visual object classes
(voc) challenge. Int. J. Comput. Vision, 88(2):303–
338.
Hammami., N., Mhalla., A., and Landrault., A. (2019). Do-
main adaptation for pedestrian dcnn detector toward
a specific scene and an embedded platform. In Pro-
ceedings of the 14th International Joint Conference
on Computer Vision, Imaging and Computer Graphics
Theory and Applications - Volume 5: VISAPP,, pages
321–327. INSTICC, SciTePress.
Lin, T., Goyal, P., Girshick, R. B., He, K., and Doll
´
ar, P.
(2017). Focal loss for dense object detection. CoRR,
abs/1708.02002.
Lin, T.-Y., Maire, M., Belongie, S., Hays, J., Perona, P., Ra-
manan, D., Doll
´
ar, P., and Zitnick, C. L. (2014). Mi-
crosoft coco: Common objects in context. In Fleet,
D., Pajdla, T., Schiele, B., and Tuytelaars, T., edi-
tors, Computer Vision – ECCV 2014, pages 740–755,
Cham. Springer International Publishing.
Maamatou, H., Chateau, T., Gazzah, S., Goyat, Y., and
ESSOUKRI BEN AMARA, N. (2016). Transductive
transfer learning to specialize a generic classifier to-
wards a specific scene. pages 411–422.
Mhalla, A., Chateau, T., Mamatou, H., Gazzah, S., and
Amara, N. E. B. (2017). Smc faster r-cnn: Toward
a scene-specialized multi-object detector. Computer
Vision and Image Understanding, 164:3 – 15. Deep
Learning for Computer Vision.
Namatevs, I., Sudars, K., and Polaka, I. (2019). Auto-
matic data labeling by neural networks for the count-
ing of objects in videos. Procedia Computer Science,
149:151 – 158. ICTE in Transportation and Logistics
2018 (ICTE 2018).
Osep, A., Voigtlaender, P., Luiten, J., Breuers, S., and
Leibe, B. (2019). Large-scale object mining for ob-
ject discovery from unlabeled video. arXiv preprint
arXiv:1903.00362.
Redmon, J. and Farhadi, A. (2018). Yolov3: An incremental
improvement. arXiv.
Ren, S., He, K., Girshick, R., and Sun, J. (2015). Faster
r-cnn: Towards real-time object detection with region
proposal networks.
Rosenberg, C., Hebert, M., and Schneiderman, H. (2005).
Semi-supervised self-training of object detection
models. WACV/MOTION, 2.
Scheirer, W. J., de Rezende Rocha, A., Sapkota, A., and
Boult, T. E. (2012). Toward open set recognition.
IEEE transactions on pattern analysis and machine
intelligence, 35(7):1757–1772.
Sobral, A. and Bouwmans, T. (2014). Bgs library:
A library framework for algorithms evaluation in
foreground/background segmentation. In Back-
ground Modeling and Foreground Detection for Video
Surveillance. CRC Press, Taylor and Francis Group.
St-Charles, P., Bilodeau, G., and Bergevin, R. (2015). Sub-
sense: A universal change detection method with lo-
cal adaptive sensitivity. IEEE Transactions on Image
Processing, 24(1):359–373.
St-Charles, P., Bilodeau, G., and Bergevin, R. (2016). Uni-
versal background subtraction using word consensus
models. IEEE Transactions on Image Processing,
25(10):4768–4781.
Uehara, K., Tejero-De-Pablos, A., Ushiku, Y., and Harada,
T. (2018). Visual question generation for class acqui-
sition of unknown objects. Proceedings of the Euro-
pean Conference on Computer Vision (ECCV), pages
481–496.
Wang, Y., Jodoin, P.-M., Porikli, F., Konrad, J., Benezeth,
Y., and Ishwar, P. (2014). Cdnet 2014: An expanded
change detection benchmark dataset. In Proceedings
of the 2014 IEEE Conference on Computer Vision and
Pattern Recognition Workshops, CVPRW ’14, pages
393–400, Washington, DC, USA. IEEE Computer So-
ciety.
Wei, H. and Kehtarnavaz, N. (2019). Semi-supervised faster
rcnn-based person detection and load classification for
far field video surveillance. Machine Learning and
Knowledge Extraction, 1(3):756767.
Yousif, H., Yuan, J., Kays, R., and He, Z. (2018). Object
detection from dynamic scene using joint background
modeling and fast deep learning classification. Jour-
nal of Visual Communication and Image Representa-
tion, 55:802 – 815.
Yu, T., Yang, J., and Lu, W. (2019a). Combining back-
ground subtraction and convolutional neural network
for anomaly detection in pumping-unit surveillance.
12:115.
Yu, T., Yang, J., and Lu, W. (2019b). Refinement of
background-subtraction methods based on convolu-
tional neural network features for dynamic back-
ground. Algorithms, 12:128.
VISAPP 2020 - 15th International Conference on Computer Vision Theory and Applications
106