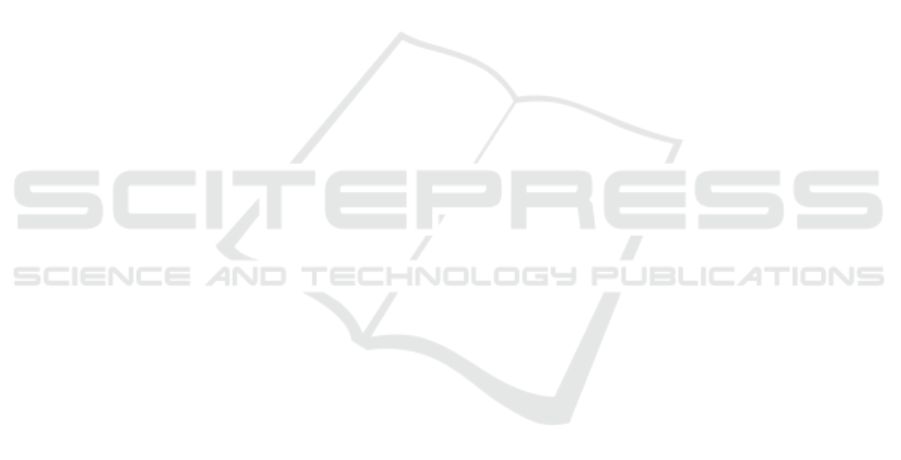
In summary, these results indicate that 2D pose es-
timation in depth data is possible at an accuracy simi-
lar to 2D pose estimation in color images, suggesting
that depth data are suitable for this purpose in terms
of the achievable accuracy. However, given the di-
verging results and the limited number of keypoints
that are consistent across detectors, we aim to carry
out more studies in the future to confirm this.
6 CONCLUSIONS
We have presented a case study on how utilizing syn-
thetic depth data for solving a practical problem via
deep learning, namely 3D human pose estimation
for health care applications, compares to alternative
means for acquiring training data. The results show
that synthetic training data are a promising alterna-
tive particularly to acquiring own realistic data if this
results in a dataset that is small by deep learning stan-
dards, despite using transfer learning. We presume
that this applies for related problems such as face and
person detection in depth data as well as these tasks
are similar in terms of data characteristics. For the
future we plan to verify this empirically and to inves-
tigate why the sensor noise simulation method em-
ployed did not lead to conclusive results. On this basis
we hope to be able to develop an improved noise sim-
ulation method that helps to further reduce the gener-
alization gap from synthetic to real data.
ACKNOWLEDGEMENTS
This work was supported by the Austrian Research
Promotion Agency (FFG-855696).
REFERENCES
Andriluka, M., Pishchulin, L., Gehler, P., and Schiele, B.
(2014). 2D Human Pose Estimation: New Benchmark
and State of the Art Analysis. In IEEE Conference
on Computer Vision and Pattern Recognition, pages
3686–3693.
Cao, Z., Hidalgo, G., Simon, T., Wei, S.-E., and Sheikh, Y.
(2018). OpenPose: Realtime Multi-Person 2D Pose
Estimation using Part Affinity Fields. arXiv preprint
arXiv:1812.08008.
Chen, C.-H. and Ramanan, D. (2017). 3D Human Pose Es-
timation = 2D Pose Estimation + Matching. In IEEE
Conference on Computer Vision and Pattern Recogni-
tion, pages 7035–7043.
Fang, H.-S., Xie, S., Tai, Y.-W., and Lu, C. (2017). RMPE:
Regional Multi-Person Pose Estimation. In Interna-
tional Conference on Computer Vision.
Guo, H., Wang, G., Chen, X., and Zhang, C. (2017). To-
wards Good Practices for Deep 3D Hand Pose Esti-
mation. arXiv preprint arXiv:1707.07248.
Haque, A., Peng, B., Luo, Z., Alahi, A., Yeung, S., and
Fei-Fei, L. (2016). Towards Viewpoint Invariant 3D
Human Pose Estimation. In European Conference on
Computer Vision, pages 160–177.
He, K., Zhang, X., Ren, S., and Sun, J. (2016). Deep Resid-
ual Learning for Image Recognition. In IEEE Con-
ference on Computer Vision and Pattern Recognition,
pages 770–778.
Howard, J. and Ruder, S. (2018). Universal Language
Model Fine-Tuning for Text Classification. arXiv
preprint arXiv:1801.06146.
Huber, P. J. (1992). Robust Estimation of a Location Param-
eter. In Breakthroughs in statistics, pages 492–518.
Springer.
Loshchilov, I. and Hutter, F. (2017). Fixing Weight Decay
Regularization in Adam. CoRR, abs/1711.05101.
Moon, G., Yong Chang, J., and Mu Lee, K. (2018). V2V-
PoseNet: Voxel-to-Voxel Prediction Network for Ac-
curate 3D Hand and Human Pose Estimation from a
Single Depth Map. In IEEE Conference on Computer
Vision and Pattern Recognition, pages 5079–5088.
Pramerdorfer, C., Kampel, M., and Heering, J. (2019). 3D
Upper-Body Pose Estimation and Classification for
Detecting Unhealthy Sitting Postures at the Work-
place. In International Conference on Informatics and
Assistive Technologies for Health-Care, Medical Sup-
port and Wellbeing.
Russakovsky, O., Deng, J., Su, H., Krause, J., Satheesh, S.,
Ma, S., Huang, Z., Karpathy, A., Khosla, A., Bern-
stein, M., Berg, A. C., and Fei-Fei, L. (2015). Ima-
geNet Large Scale Visual Recognition Challenge. In-
ternational Journal of Computer Vision, 115(3):211–
252.
Shotton, J., Fitzgibbon, A., Cook, M., Sharp, T., Finocchio,
M., Moore, R., Kipman, A., and Blake, A. (2011).
Real-Time Human Pose Recognition in Parts from
Single Depth Images. In IEEE Conference on Com-
puter Vision and Pattern Recognition, pages 1297–
1304. Ieee.
Sun, X., Xiao, B., Wei, F., Liang, S., and Wei, Y. (2018).
Integral Human Pose Regression. In European Con-
ference on Computer Vision, pages 529–545.
Toshev, A. and Szegedy, C. (2014). DeepPose: Human Pose
Estimation via Deep Neural Networks. In IEEE Con-
ference on Computer Vision and Pattern Recognition,
pages 1653–1660.
Varol, G., Romero, J., Martin, X., Mahmood, N., Black,
M. J., Laptev, I., and Schmid, C. (2017). Learning
from Synthetic Humans. In IEEE Conference on Com-
puter Vision and Pattern Recognition, pages 109–117.
Xu, C. and Cheng, L. (2013). Efficient Hand Pose Esti-
mation from a Single Depth Image. In International
Conference on Computer Vision, pages 3456–3462.
Deep Body-pose Estimation via Synthetic Depth Data: A Case Study
325