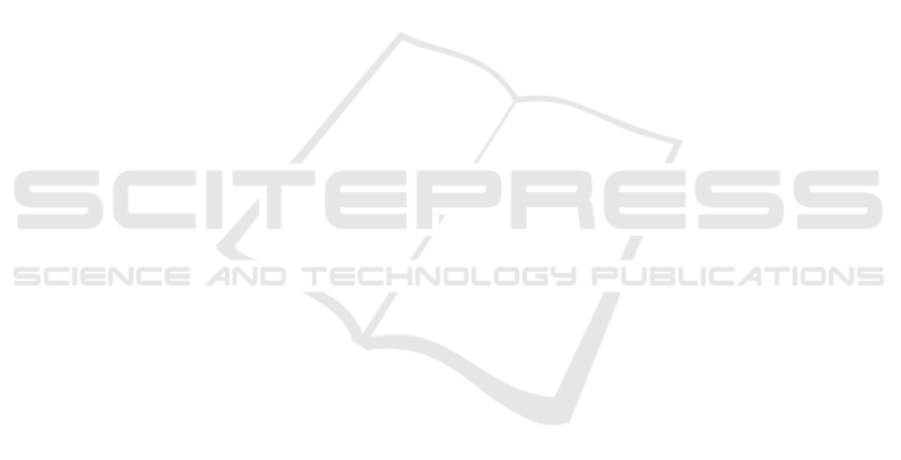
ACKNOWLEDGMENTS
The support of this work in part by the Ministry
of Science and Technology of Taiwan under Grant
MOST 106-2221-E-194-004 and the Advanced In-
stitute of Manufacturing with High-tech Innovations
(AIM-HI) from The Featured Areas Research Cen-
ter Program within the framework of the Higher Ed-
ucation Sprout Project by the Ministry of Education
(MOE) in Taiwan is gratefully acknowledged.
REFERENCES
Benjdira, B., Khursheed, T., Koubaa, A., Ammar, A., and
Ouni, K. (2019). Car Detection using Unmanned
Aerial Vehicles: Comparison between Faster R-CNN
and YOLOv3Benjdira, B., Khursheed, T., Koubaa,
A., Ammar, A., &Ouni, K. (2019). Car Detection
using Unmanned Aerial Vehicles: Comparison be-
tween Faster R-CNN and YOLOv3. 2019 1s. In 2019
1st International Conference on Unmanned Vehicle
Systems-Oman, UVS 2019.
Cheng, G. and Han, J. (2016). A survey on object detection
in optical remote sensing images.
Deng, Z., Sun, H., Zhou, S., Zhao, J., and Zou, H. (2017).
Toward Fast and Accurate Vehicle Detection in Aerial
Images Using Coupled Region-Based Convolutional
Neural Networks. IEEE Journal of Selected Topics in
Applied Earth Observations and Remote Sensing.
Girshick, R. (2015). Fast R-CNN. In Proceedings of the
IEEE International Conference on Computer Vision.
Kembhavi, A., Harwood, D., and Davis, L. S. (2011). Vehi-
cle Detection using Partial Least Squares. IEEE Trans.
Pattern Analysis and Machine Intelligence (PAMI),
33(6):1250–1265.
Lenhart, D., Hinz, S., Leitloff, J., and Stilla, U. (2008). Au-
tomatic traffic monitoring based on aerial image se-
quences. Pattern Recognition and Image Analysis.
Liu, W., Anguelov, D., Erhan, D., Szegedy, C., Reed, S.,
Fu, C. Y., and Berg, A. C. (2016). SSD: Single shot
multibox detector. In Lecture Notes in Computer Sci-
ence (including subseries Lecture Notes in Artificial
Intelligence and Lecture Notes in Bioinformatics).
Lu, J., Ma, C., Li, L., Xing, X., Zhang, Y., Wang, Z., and
Xu, J. (2018). A Vehicle Detection Method for Aerial
Image Based on YOLO. Journal of Computer and
Communications.
Razakarivony, S. and Jurie, F. (2016). Vehicle detection in
aerial imagery: A small target detection benchmark.
Journal of Visual Communication and Image Repre-
sentation.
Redmon, J., Divvala, S., Girshick, R., and Farhadi, A.
(2016). You only look once: Unified, real-time ob-
ject detection. In Proceedings of the IEEE Computer
Society Conference on Computer Vision and Pattern
Recognition.
Redmon, J. and Farhadi, A. (2017). YOLO9000: Better,
faster, stronger. In Proceedings - 30th IEEE Con-
ference on Computer Vision and Pattern Recognition,
CVPR 2017.
Redmon, J. and Farhadi, A. (2018). YOLO v.3. Tech report.
Ren, S., He, K., Girshick, R., and Sun, J. (2017). Faster R-
CNN: Towards Real-Time Object Detection with Re-
gion Proposal Networks. IEEE Transactions on Pat-
tern Analysis and Machine Intelligence.
Ren, Y., Zhu, C., and Xiao, S. (2018). Object Detection
Based on Fast/Faster RCNN Employing Fully Con-
volutional Architectures. Mathematical Problems in
Engineering.
Shao, W., Yang, W., Liu, G., and Liu, J. (2012). Car detec-
tion from high-resolution aerial imagery using multi-
ple features. In International Geoscience and Remote
Sensing Symposium (IGARSS).
Sommer, L. W., Schuchert, T., and Beyerer, J. (2017). Fast
deep vehicle detection in aerial images. In Proceed-
ings - 2017 IEEE Winter Conference on Applications
of Computer Vision, WACV 2017.
Tang, T., Zhou, S., Deng, Z., Lei, L., and Zou, H. (2017).
Arbitrary-oriented vehicle detection in aerial imagery
with single convolutional neural networks. Remote
Sensing.
Xia, G. S., Bai, X., Ding, J., Zhu, Z., Belongie, S., Luo, J.,
Datcu, M., Pelillo, M., and Zhang, L. (2018). DOTA:
A Large-Scale Dataset for Object Detection in Aerial
Images. In Proceedings of the IEEE Computer Society
Conference on Computer Vision and Pattern Recogni-
tion.
Yalcin, H., Hebert, M., Collins, R., and Black, M. J. (2005).
A flow-based approach to vehicle detection and back-
ground mosaicking in airborne video. In Proceedings
- 2005 IEEE Computer Society Conference on Com-
puter Vision and Pattern Recognition, CVPR 2005.
VISAPP 2020 - 15th International Conference on Computer Vision Theory and Applications
782