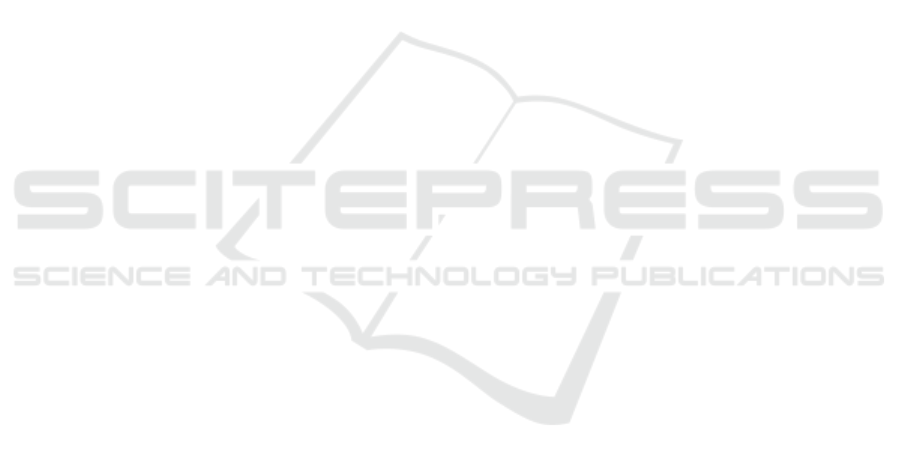
REFERENCES
Ahad, M. A. R., Tan, J. K., Kim, H., and Ishikawa, S.
(2012). Motion history image: its variants and appli-
cations. Machine Vision and Applications, 23(2):255–
281.
Auffarth, B., L
´
opez, M., and Cerquides, J. (2010). Compar-
ison of redundancy and relevance measures for feature
selection in tissue classification of ct images. In In-
dustrial Conference on Data Mining, pages 248–262.
Springer.
Biesiada, J., Duch, W., Kachel, A., Maczka, K., and
Palucha, S. (2005). Feature ranking methods based on
information entropy with parzen windows. In Interna-
tional Conference on Research in Electrotechnology
and Applied Informatics, pages 1–9.
Bobick, A. F. and Davis, J. W. (2001). The recognition
of human movement using temporal templates. IEEE
Transactions on Pattern Analysis & Machine Intelli-
gence, (3):257–267.
Coster, M. and Chermant, J.-L. (1989). Pr
´
ecis d’analyse
d’images. Technical report, Presses du CNRS.
Dalal, N. and Triggs, B. (2005). Histograms of oriented
gradients for human detection. In Computer Vision
and Pattern Recognition (CVPR).
Filippoupolitis, A., Oliff, W., Takand, B., and Loukas, G.
(2017). Location-enhanced activity recognition in
indoor environments using off the shelf smartwatch
technology and ble beacons. Sensors, 17(6):1230.
Gorelick, L., Blank, M., Shechtman, E., Irani, M., and
Basri, R. (2007). Actions as space-time shapes. IEEE
transactions on pattern analysis and machine intelli-
gence, 29(12):2247–2253.
Hall, M. A. (1999). Correlation-based feature selection for
machine learning. PhD thesis, New Zealand, Depart-
ment of Computer Science, Waikato University.
Hofmann, T. (1999). Probabilistic latent semantic analysis.
In Proceedings of the Fifteenth conference on Uncer-
tainty in artificial intelligence, pages 289–296.
Hu, M.-K. (1962). Visual pattern recognition by moment
invariants. IRE transactions on information theory,
8(2):179–187.
Jalal, A., Kim, Y.-H., Kim, Y.-J., Kamal, S., and Kim, D.
(2017). Robust human activity recognition from depth
video using spatiotemporal multi-fused features. Pat-
tern recognition, 61:295–308.
Ji, S., Xu, W., Yang, M., and Yu, K. (2012). 3d convolu-
tional neural networks for human action recognition.
IEEE transactions on pattern analysis and machine
intelligence, 35(1):221–231.
Laptev, I., Marszałek, M., Schmid, C., and Rozenfeld,
B. (2008). Learning realistic human actions from
movies.
Liu, L., Shao, L., and Rockett, P. (2013). Boosted
key-frame selection and correlated pyramidal motion-
feature representation for human action recognition.
Pattern recognition, 46(7):1810–1818.
Lowe, D. G. (2004). Distinctive image features from scale-
invariant keypoints. International journal of computer
vision, 60(2):91–110.
Luo, X., Guan, Q., Tan, H., Gao, L., Wang, Z., and Luo,
X. (2017). Simultaneous indoor tracking and activity
recognition using pyroelectric infrared sensors. Sen-
sors (Basel), 17(8):1738.
Mohibullah, M., Hossain, M. Z., and Hasan, M. (2015).
Comparison of euclidean distance function and man-
hattan distance function using k-mediods. Interna-
tional Journal of Computer Science and Information
Security, 13(10):61.
Peng, H., Long, F., and Ding, C. (2005). Feature selec-
tion based on mutual information: criteria of max-
dependency, max-relevance, and min-redundancy.
IEEE Transactions on Pattern Analysis & Machine In-
telligence, (8):1226–1238.
Polla, F., Boudjelaba, K., Emile, B., and Laurent, H.
(2017). Proposal of segmentation method adapted
to the infrared sensor. In International Conference
on Advanced Concepts for Intelligent Vision Systems
(ACIVS), pages 639–650. Springer.
Polla, F., Laurent, H., and Emile, B. (2019). Action recog-
nition from low-resolution infrared sensor for indoor
use: a comparative study between deep learning and
classical approaches. In 20th IEEE International Con-
ference on Mobile Data Management (MDM), pages
409–414.
Ragb, H. K. and Asari, V. K. (2016). Color and local
phase based descriptor for human detection. In 2016
IEEE National Aerospace and Electronics Conference
(NAECON) and Ohio Innovation Summit (OIS), pages
68–73.
Robertson, N. and Reid, I. (2005). Behaviour understand-
ing in video: a combined method. In Tenth IEEE In-
ternational Conference on Computer Vision (ICCV),
volume 1, pages 808–815.
Roffo, G., Melzi, S., Castellani, U., and Vinciarelli, A.
(2017). Infinite latent feature selection: A probabilis-
tic latent graph-based ranking approach. In Proceed-
ings of the IEEE International Conference on Com-
puter Vision, pages 1398–1406.
Sefen, B., Baumbach, S., Dengel, A., and Abdennadher, S.
(2016). Human activity recognition using sensor data
of smartphones and smartwatches. In Proceedings of
the 8th International Conference on Agents and Artifi-
cial Intelligence (ICAART), Volume 2, pages 488–493.
Wang, H., Kl
¨
aser, A., Schmid, C., and Liu, C.-L. (2013).
Dense trajectories and motion boundary descriptors
for action recognition. International journal of com-
puter vision, 103(1):60–79.
Yu, L. and Liu, H. (2003). Feature selection for high-
dimensional data: A fast correlation-based filter so-
lution. In Proceedings of the 20th international con-
ference on machine learning (ICML), pages 856–863.
VISAPP 2020 - 15th International Conference on Computer Vision Theory and Applications
236