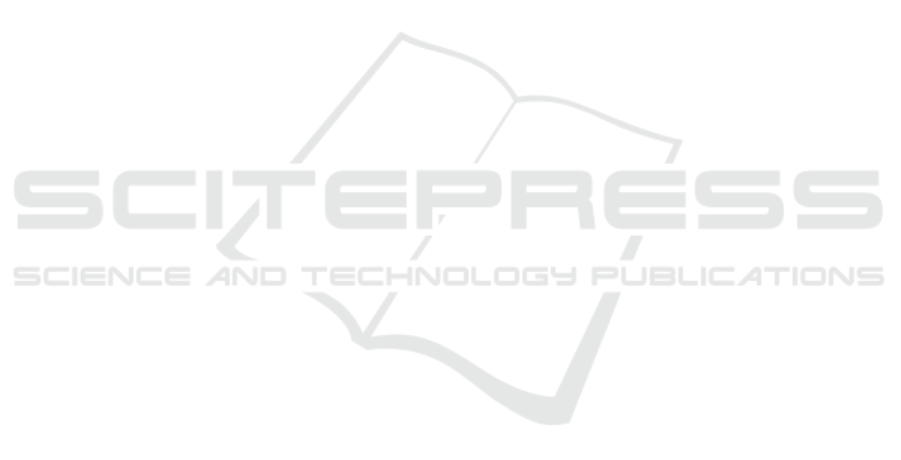
821966. This publication reflects the views only of
the authors, and the European Commission cannot be
held responsible for any use which may be made of
the information contained therein.
REFERENCES
Agustsson, E., Timofte, R., Escalera, S., Bar
´
o, X., Guyon,
I., and Rothe, R. (2017). Apparent and real age esti-
mation in still images with deep residual regressors on
APPA-REAL database. In FG 2017 - 12th IEEE Inter-
national Conference on Automatic Face and Gesture
Recognition, pages 1–12.
Al-Nabki, M. W., Fidalgo, E., Alegre, E., and Fern
´
andez-
Robles, L. (2019). Torank: Identifying the most influ-
ential suspicious domains in the tor network. Expert
Systems with Applications, 123:212 – 226.
Alrjebi, M., Pathirage, N., Liu, W., and Li, L. (2017). Face
recognition against occlusions via colour fusion using
2d-mcf model and src. Pattern Recognition Letters,
95:1339–1351.
Anda, F., Lillis, D., Kanta, A., Becker, B. A., Bou-Harb,
E., Le-Khac, N.-A., and Scanlon, M. (2019). Improv-
ing borderline adulthood facial age estimation through
ensemble learning. In 14th International Conference
on Availability, Reliability and Security (ARES ’19),
pages 1–8.
Angulu, R., Tapamo, J. R., and Adewumi, A. O. (2018).
Age estimation via face images: a survey. EURASIP
Journal on Image and Video Processing, 2018(1):42.
Antipov, G., Baccouche, M., Berrani, S., and Duge-
lay, J. (2016). Apparent age estimation from face
images combining general and children-specialized
deep learning models. In 2016 IEEE Conference on
Computer Vision and Pattern Recognition Workshops
(CVPRW), pages 801–809.
Biswas, R., Gonz
´
alez-Castro, V., Fidalgo, E., and Chaves,
D. (2019). Boosting child abuse victim identification
in forensic tools with hashing techniques. In V Jor-
nadas Nacionales de Investigaci
´
on en Ciberseguridad
(JNIC), volume 1, pages 344–345.
Carcagn
`
ı, P., Coco, M. D., Cazzato, D., Leo, M., and Dis-
tante, C. (2015). A study on different experimental
configurations for age, race, and gender estimation
problems. EURASIP Journal on Image and Video Pro-
cessing, 2015:1–22.
Cen, F. and Wang, G. (2019). Dictionary representation
of deep features for occlusion-robust face recognition.
IEEE Access, 7:26595 – 26605.
Chaves, D., Fidalgo, E., Alegre, E., and Blanco, P. (2019).
Improving speed-accuracy trade-off in face detectors
for forensic tools by image resizing. In V Jor-
nadas Nacionales de Investigaci
´
on en Ciberseguridad
(JNIC), pages 1–2.
Chen, S., Zhang, C., Dong, M., Le, J., and Rao, M. (2017).
Using ranking-cnn for age estimation. In 2017 IEEE
Conference on Computer Vision and Pattern Recogni-
tion (CVPR), pages 742–751.
Galusha, A., Dale, J., Keller, J. M., and Zare, A. (2019).
Deep convolutional neural network target classifica-
tion for underwater synthetic aperture sonar imagery.
In Bishop, S. S. and Isaacs, J. C., editors, Detection
and Sensing of Mines, Explosive Objects, and Ob-
scured Targets XXIV, volume 11012, pages 18 – 28.
Gangwar, A., Fidalgo, E., Alegre, E., and Gonz
´
alez-Castro,
V. (2017). Pornography and child sexual abuse detec-
tion in image and video: A comparative evaluation. In
8th International Conference on Imaging for Crime
Detection and Prevention (ICDP), pages 37–42.
Grd, P. and Ba
ˇ
ca, M. (2016). Creating a face database for
age estimation and classification. In 2016 39th Inter-
national Convention on Information and Communi-
cation Technology, Electronics and Microelectronics
(MIPRO), pages 1371–1374.
Hase, N., Ito, S., Kaneko, N., and Sumi, K. (2019). Data
augmentation for intra-class imbalance with genera-
tive adversarial network. In Fourteenth International
Conference on Quality Control by Artificial Vision,
volume 11172, pages 34 – 41.
Min, R., Hadid, A., and Dugelay, J.-L. (2011). Improv-
ing the recognition of faces occluded by facial acces-
sories. In 2011 IEEE International Conference on
Automatic Face and Gesture Recognition and Work-
shops, FG 2011, pages 442 – 447.
Moschoglou, S., Papaioannou, A., Sagonas, C., Deng, J.,
Kotsia, I., and Zafeiriou, S. (2017). Agedb: The first
manually collected, in-the-wild age database. 2017
IEEE Conference on Computer Vision and Pattern
Recognition Workshops (CVPRW), pages 1997–2005.
Ricanek, K. and Tesafaye, T. (2006). Morph: A longitudi-
nal image database of normal adult age-progression.
FGR 2006: Proceedings of the 7th International Con-
ference on Automatic Face and Gesture Recognition,
2006:341 – 345.
Rothe, R., Timofte, R., and Gool, L. V. (2015). Dex: Deep
expectation of apparent age from a single image. In
IEEE International Conference on Computer Vision
Workshops (ICCVW), pages 10–15.
Yadav, D., Singh, R., Vatsa, M., and Noore, A. (2014). Rec-
ognizing age-separated face images: Humans and ma-
chines. PLoS ONE, 9(12):1–22.
Yang, T.-Y., Huang, Y.-H., Lin, Y.-Y., Hsiu, P.-C., and
Chuang, Y.-Y. (2018). Ssr-net: A compact soft
stagewise regression network for age estimation.
In Proceedings of the Twenty-Seventh International
Joint Conference on Artificial Intelligence (IJCAI-18),
pages 1–7.
Ye, L., Li, B., Mohammed, N., Wang, Y., and Liang, J.
(2018). Privacy-preserving age estimation for content
rating. In 2018 IEEE 20th International Workshop on
Multimedia Signal Processing (MMSP), pages 1–6.
Yi, D., Lei, Z., and Li, S. (2015). Age estimation by multi-
scale convolutional network. In Conference: Asian
Conference on Computer Vision, volume 9005, pages
144–158.
Zhang, C., Liu, S., Xu, X., and Zhu, C. (2019). C3AE:
exploring the limits of compact model for age estima-
tion. CoRR, abs/1904.05059:1–10.
VISAPP 2020 - 15th International Conference on Computer Vision Theory and Applications
728