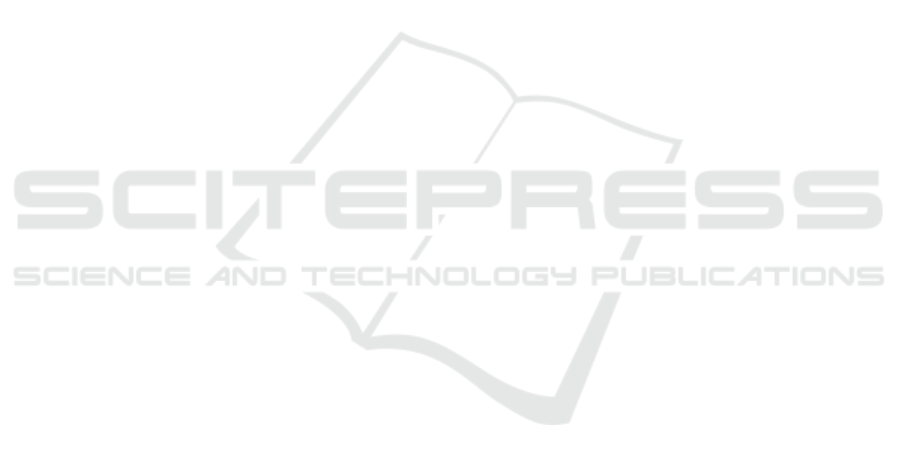
applications in the atmospheric sciences. Atmospheric
Environment, 32(14–15), 2627–2636. https://doi.org/
10.1016/S1352-2310(97)00447-0
Gooch, J. W. (2011). Pearson Product-Moment Correlation
Coefficient. In Encyclopedia of Measurement and
Statistics. https://doi.org/
10.4135/9781412952644.n338
Guyon, I., Steve, G., Masoud, N., & Lotfi, A. Z. (2006).
Feature Extraction: Foundations and Applications (I.
Guyon, M. Nikravesh, S. Gunn, & L. A. Zadeh, Eds.).
https://doi.org/10.1007/978-3-540-35488-8
Hall, M., Frank, E., Holmes, G., Pfahringer, B., Reutemann,
P., & Witten, I. H. (2009). The WEKA data mining
software. ACM SIGKDD Explorations Newsletter.
https://doi.org/10.1145/1656274.1656278
Hosni, M., Idri, A., & Abran, A. (2017). Investigating
heterogeneous ensembles with filter feature selection for
software effort estimation. Proceedings of the 27th
International Workshop on Software Measurement and
12th International Conference on Software Process and
Product Measurement, 207–220.
https://doi.org/10.1145/3143434.3143456
Huan, L., & Lei, Y. (2005). Toward integrating feature
selection algorithms for classification and clustering.
IEEE Transactions on Knowledge and Data
Engineering, 17(4), 491–502. https://doi.org/
10.1109/TKDE.2005.66
Idri, A., Benhar, H., Fernández-Alemán, J. L., & Kadi, I.
(2018). A systematic map of medical data preprocessing
in knowledge discovery. Computer Methods and
Programs in Biomedicine, 162, 69–85.
https://doi.org/10.1016/j.cmpb.2018.05.007
Jabbar, M. A., Deekshatulu, B. L., & Chandra, P. (2013).
Heart disease classification using nearest neighbor
classifier with feature subset selection. ANNALS
.COMPUTER SCIENCE SERIES, Vol. XI fa, 47–54.
Jabbar, M. A., Deekshatulu, B. L., & Chandra, P. (2015).
Computational intelligence technique for early
diagnosis of heart disease. 2015 IEEE International
Conference on Engineering and Technology
(ICETECH), 1–6. https://doi.org/10.1109/
ICETECH.2015.7275001
Jaganathan, P., & Kuppuchamy, R. (2013). A threshold
fuzzy entropy based feature selection for medical
database classification. Computers in Biology and
Medicine, 43(12), 2222–2229. https://doi.org/10.1016/
j.compbiomed.2013.10.016
Jovic, A., Brkic, K., & Bogunovic, N. (2015). A review of
feature selection methods with applications. 2015 38th
International Convention on Information and
Communication Technology, Electronics and
Microelectronics (MIPRO), 1200–1205. https://doi.
org/10.1109/MIPRO.2015.7160458
Kadi, I., Idri, A., & Fernandez-Aleman, J. L. (2017).
Knowledge discovery in cardiology: A systematic
literature review. International Journal of Medical
Informatics, 97, 12–32. https://doi.org/10.1016/j.ijmed
inf.2016.09.005
Kenji, K., & A., R. L. (1992). The Feature Selection
Problem : Traditional Methods and a New Algorithm.
AAAI-92 Proceedings. https://doi.org/10.1016/S0031-
3203(01)00046-2
Kononenko, I., Robnik-Šikonja, M., & Pompe, U. (1996).
ReliefF for estimation and discretization of attributes in
classification, regression, and ILP problems. Artificial
Intelligence: Methodology, Systems, Applications, 31–
40. https://doi.org/DOI 10.1016/
j.chroma.2012.01.030
Mendes, D., Paredes, S., Rocha, T., Carvalho, P.,
Henriques, J., Cabiddu, R., & Morais, J. (2015).
Assessment of cardiovascular risk based on a data-
driven knowledge discovery approach. 2015 37th
Annual International Conference of the IEEE
Engineering in Medicine and Biology Society (EMBC),
6800–6803. https://doi.org/10.1109/EMBC.2015.7319
955
Mustaqeem, A., Anwar, S. M., Majid, M., & Khan, A. R.
(2017). Wrapper method for feature selection to classify
cardiac arrhythmia. Proceedings of the Annual
International Conference of the IEEE Engineering in
Medicine and Biology Society, EMBS, 3656–3659.
https://doi.org/10.1109/EMBC.2017.8037650
Peter, T. J., & Somasundaram, K. (2012). Study and
Development of Novel Feature Selection Framework
for Heart Disease Prediction. International Journal of
Scientific and Research Publications, 2(10), 1–7.
Quinlan, J. R. (1986). Induction of Decision Trees. Machine
Learning, 1(1), 81–106. https://doi.org/
10.1023/A:1022643204877
Sasikala, S., Appavu, A. B. S., & Geetha, S. (2014). RF-
SEA-Based Feature Selection for Data Classification in
Medical Domain. Intelligent Computing, Networking,
and Informatics, 243, 599–608.
https://doi.org/10.1007/978-81-322-1665-0_59
Scott, A. J., & Knott, M. (1974). A Cluster Analysis Method
for Grouping Means in the Analysis of Variance.
Biometrics. https://doi.org/10.2307/2529204
Seijo-Pardo, B., Porto-Díaz, I., Bolón-Canedo, V., &
Alonso-Betanzos, A. (2017). Ensemble feature
selection: Homogeneous and heterogeneous approaches.
Knowledge-Based Systems, 118, 124–139.
https://doi.org/10.1016/j.knosys.2016.11.017
Shouman, M., Turner, T., & Stocker, R. (2012). Using data
mining techniques in heart disease diagnosis and
treatment. 2012 Japan-Egypt Conference on
Electronics, Communications and Computers, 173–177.
https://doi.org/10.1109/JEC-ECC.2012.6186978
Ting, S. L., Shum, C. C., Kwok, S. K., Tsang, A. H. C., &
Lee, W. B. (2009). Data Mining in Biomedicine:
Current Applications and Further Directions for
Research. Journal of Software Engineering and
Applications, 02(03), 150–159. https://doi.org/10.
4236/jsea.2009.23022
Vapnik, V. N. (2000). The Nature of Statistical Learning
Theory. https://doi.org/10.1007/978-1-4757-3264-1
Vivekanandan, T., & Sriman, N. I. N. C. (2017). Optimal
feature selection using a modified differential evolution
algorithm and its effectiveness for prediction of heart
disease. Computers in Biology and Medicine, 90, 125–
136. https://doi.org/10.1016/j.compbiomed.2017.09.011
HEALTHINF 2020 - 13th International Conference on Health Informatics
398