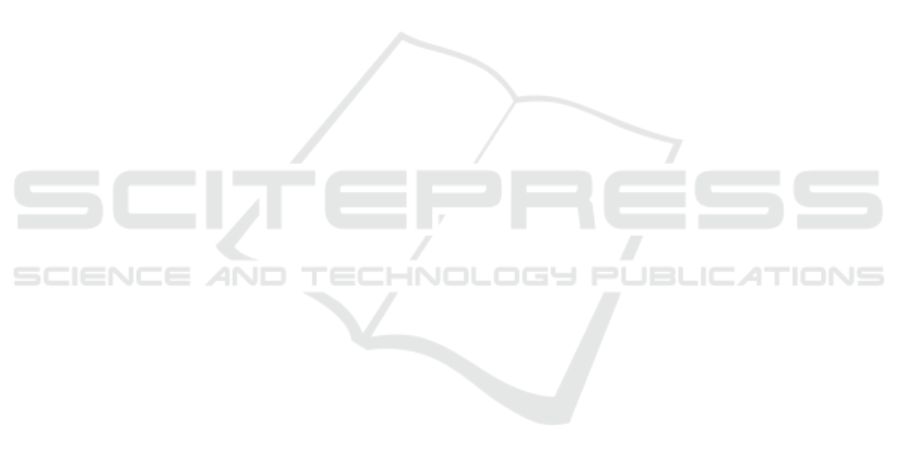
the lightness axis becomes, the more central the color
signal is located. The evolution of the spectral recon-
struction error over the lightness axis is displayed in
Fig. 7a as box plot. The considered error metric is
the mean absolute error (MAE), i.e. the average Eu-
clidean distance of the computed spectral reconstruc-
tion to its ground truth. A direct increase of the error
metric depending on the camera signal position is im-
mediately apparent.
Likewise to the proposed metameric loss, it is also
possible to project all spectral reconstructions back
into camera signal space and compare the result to the
input RGB image. When performing the same anal-
ysis as before but this time inside the camera signal
space, another trend is observed and shown in Fig. 7b.
In contrast to the spectral domain, the reconstruction
error remains rather constant independent of the po-
sition inside the signal space. It is worth highlighting
the average absolute error inside the camera signal do-
main. Since we are assuming an 8bit encoding and
therefore signals ranging from 0 to 255, the average
absolute errors are in fact in the same range as po-
tential quantization noise. One might argue, that the
reconstruction itself is already close to ideal. Any ad-
ditionally introduced reconstruction error within the
spectral domain must thus be along dimensions that
are not observable by the camera system. Therefore,
the limits of spectral reconstruction from RGB image
acquisition appear to be already reached. The map-
ping from one camera signal to many possible spec-
tral signals cannot be easily solved and most likely
only be further optimized in a significant way by em-
ploying multi-spectral imaging. The interesting re-
sult though is, that the neural network appears to
be already capable of implicitly learning the realm
of metameric blacks itself. Made reconstruction er-
rors are mostly introduced in a meaningful way along
spectral dimensions no information is available on.
For further research, it would be highly interesting to
understand how and in what form the network actu-
ally represents the information.
6 CONCLUSION
Within this work, a modification to neural networks
which perform the task of spectral reconstruction
from camera images was proposed. The modification
is based upon the idea to mathematically enforce the
reconstruction to be at least within the metameric sub-
set of spectral stimuli to the true stimulus. The poten-
tial positive impact of the modification was demon-
strated by applying it to a state-of-the-art model and
using it to reconstruct spectral images from differ-
ent simulated RGB cameras. Since the enforced
metameric constraint directly corresponds to a better
initialization, the training process also converges sig-
nificantly faster. However, above findings only hold
true in an ideal world. The metameric based recon-
struction was found to be highly sensitive to noise,
probably preventing an application in the real world.
It was further demonstrated, that a consideration of
metamerism within the loss function does not yield
any positive effects at all. The reason is that knowl-
edge of a cameras’ sensitivity can already be success-
fully learned by directly training a neural network to
learn an end-to-end mapping from the camera signal
space to the spectral domain. As shown within this
work, such self-learned knowledge must be contained
somewhere within a fully trained network. However,
it is unclear in what form, leaving the potential extrac-
tion of a learned camera sensitivity from the network
as an interesting topic for further research.
REFERENCES
Aeschbacher, J., Wu, J., and Timofte, R. (2017). In de-
fense of shallow learned spectral reconstruction from
rgb images. 2017 IEEE International Conference on
Computer Vision Workshops (ICCVW), pages 471–
479.
Arad, B. and Ben-Shahar, O. (2016). Sparse recovery of
hyperspectral signal from natural rgb images. In Eu-
ropean Conference on Computer Vision, pages 19–34.
Springer.
Arad, B., Ben-Shahar, O., Timofte, R., Van Gool, L., Zhang,
L., Yang, M.-H., et al. (2018). Ntire 2018 challenge on
spectral reconstruction from rgb images. In The IEEE
Conference on Computer Vision and Pattern Recogni-
tion (CVPR) Workshops.
Bianco, S. (2010). Reflectance spectra recovery from tris-
timulus values by adaptive estimation with metameric
shape correction. J. Opt. Soc. Am. A, 27(8):1868–
1877.
Finlayson, G. D. and Morovic, P. (2005). Metamer sets. J.
Opt. Soc. Am. A, 22(5):810–819.
Gijsenij, A., Gevers, T., and van de Weijer, J. (2011). Com-
putational color constancy: Survey and experiments.
IEEE Transactions on Image Processing, 20:2475–
2489.
Hardeberg, J. Y., Schmitt, F. J. M., and Brettel, H. (1999).
Multispectral image capture using a tunable filter. In
Proc.SPIE, volume 3963, pages 3963 – 3963 – 12.
Hill, B. (2002). Optimization of total multispectral imag-
ing systems: best spectral match versus least observer
metamerism. In 9th Congress of the International
Colour Association, volume 4421, pages 481–486.
Imai, F. H., Rosen, M. R., and Berns, R. S. (2002). Com-
parative study of metrics for spectral match quality.
In Conference on Colour in Graphics, Imaging, and
Vision (CGIV), pages 492–496.
Enhancing Deep Spectral Super-resolution from RGB Images by Enforcing the Metameric Constraint
65