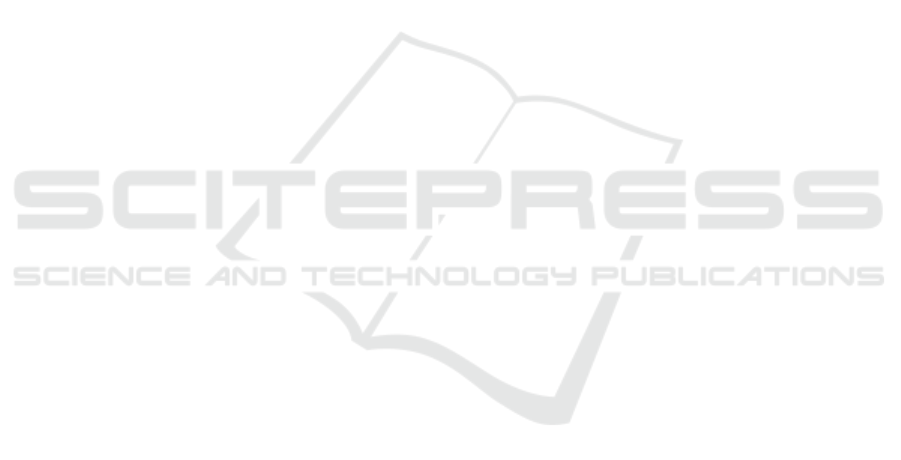
bone to reduce execution time and the prediction
blocks to enrich the source feature maps. By com-
bining them, FSSSD runs at 16.7 FPS, which is faster
than any two-stage detectors and achieves 63.7% AP,
which is the highest one among all one-stage detec-
tors.
ACKNOWLEDGEMENTS
This work was partly supported by Institute of Infor-
mation & Communications Technology Planning &
Evaluation(IITP) grant funded by the Korea govern-
ment(MSIT) (No.2014-3-00077, AI National Strat-
egy Project) and the National Research Foundation
of Korea (NRF) grant funded by the Korea govern-
ment(MSIT) (No. 2019R1A2C2087489).
REFERENCES
Dai, J., Li, Y., He, K., and Sun, J. (2016). R-FCN: object de-
tection via region-based fully convolutional networks.
CoRR, abs/1605.06409.
Girshick, R. (2015). Fast r-cnn. In Proceedings of the 2015
IEEE International Conference on Computer Vision
(ICCV), ICCV ’15, pages 1440–1448, Washington,
DC, USA. IEEE Computer Society.
Girshick, R., Donahue, J., Darrell, T., and Malik, J. (2014).
Rich feature hierarchies for accurate object detection
and semantic segmentation. In Proceedings of the
2014 IEEE Conference on Computer Vision and Pat-
tern Recognition, CVPR ’14, pages 580–587, Wash-
ington, DC, USA. IEEE Computer Society.
He, K., Gkioxari, G., Doll
´
ar, P., and Girshick, R. (2017).
Mask r-cnn. In Proceedings of the IEEE international
conference on computer vision, pages 2961–2969.
Hu, P. and Ramanan, D. (2017). Finding tiny faces. In Pro-
ceedings of the IEEE conference on computer vision
and pattern recognition, pages 951–959.
Lee, K., Choi, J., Jeong, J., and Kwak, N. (2017). Resid-
ual features and unified prediction network for single
stage detection. CoRR, abs/1707.05031.
Lin, T.-Y., Doll
´
ar, P., Girshick, R., He, K., Hariharan, B.,
and Belongie, S. (2017). Feature pyramid networks
for object detection. In Proceedings of the IEEE con-
ference on computer vision and pattern recognition,
pages 2117–2125.
Liu, W., Anguelov, D., Erhan, D., Szegedy, C., Reed, S.,
Fu, C.-Y., and Berg, A. C. (2016). Ssd: Single shot
multibox detector. In European conference on com-
puter vision, pages 21–37. Springer.
Ma, N., Zhang, X., Zheng, H.-T., and Sun, J. (2018). Shuf-
flenet v2: Practical guidelines for efficient cnn archi-
tecture design. In Proceedings of the European Con-
ference on Computer Vision (ECCV), pages 116–131.
Paszke, A., Gross, S., Chintala, S., Chanan, G., Yang, E.,
DeVito, Z., Lin, Z., Desmaison, A., Antiga, L., and
Lerer, A. (2017). Automatic differentiation in Py-
Torch. In NIPS Autodiff Workshop.
Redmon, J., Divvala, S. K., Girshick, R. B., and Farhadi, A.
(2015). You only look once: Unified, real-time object
detection. CoRR, abs/1506.02640.
Redmon, J. and Farhadi, A. (2017). Yolo9000: better, faster,
stronger. In Proceedings of the IEEE conference on
computer vision and pattern recognition, pages 7263–
7271.
Ren, S., He, K., Girshick, R., and Sun, J. (2015). Faster
r-cnn: Towards real-time object detection with region
proposal networks. In Cortes, C., Lawrence, N. D.,
Lee, D. D., Sugiyama, M., and Garnett, R., editors,
Advances in Neural Information Processing Systems
28, pages 91–99. Curran Associates, Inc.
Russakovsky, O., Deng, J., Su, H., Krause, J., Satheesh,
S., Ma, S., Huang, Z., Karpathy, A., Khosla, A.,
Bernstein, M., Berg, A. C., and Fei-Fei, L. (2015).
ImageNet Large Scale Visual Recognition Challenge.
International Journal of Computer Vision (IJCV),
115(3):211–252.
Santurkar, S., Tsipras, D., Ilyas, A., and Madry, A. (2018).
How does batch normalization help optimization?(no,
it is not about internal covariate shift). arXiv preprint
arXiv:1805.11604.
Simonyan, K. and Zisserman, A. (2014). Very deep con-
volutional networks for large-scale image recognition.
arXiv preprint arXiv:1409.1556.
Uijlings, J., van de Sande, K., Gevers, T., and Smeulders,
A. (2013). Selective search for object recognition. In-
ternational Journal of Computer Vision.
Wang, L., Lu, Y., Wang, H., Zheng, Y., Ye, H., and Xue,
X. (2017). Evolving boxes for fast vehicle detection.
CoRR, abs/1702.00254.
Wen, L., Du, D., Cai, Z., Lei, Z., Chang, M., Qi, H., Lim,
J., Yang, M., and Lyu, S. (2015). UA-DETRAC: A
new benchmark and protocol for multi-object detec-
tion and tracking. arXiv CoRR, abs/1511.04136.
VISAPP 2020 - 15th International Conference on Computer Vision Theory and Applications
342