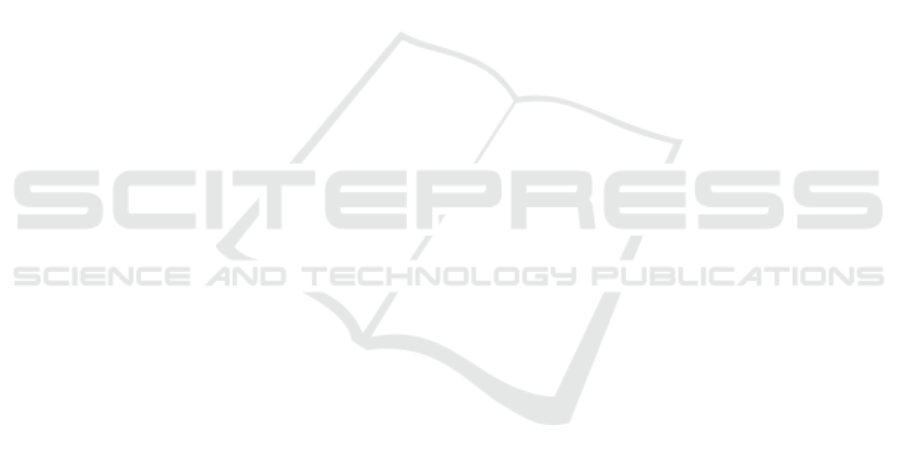
REFERENCES
Alsentzer, E., Murphy, J. R., Boag, W., Weng, W.-H.,
Jin, D., Naumann, T., and McDermott, M. (2019).
Publicly available clinical bert embeddings. arXiv
preprint arXiv:1904.03323.
Barman, U., Das, A., Wagner, J., and Foster, J. (2014).
Code mixing: A challenge for language identification
in the language of social media. In Proceedings of the
first workshop on computational approaches to code
switching, pages 13–23.
Bender, E. M. (2009). Linguistically na
¨
ıve != language in-
dependent: Why NLP needs linguistic typology. In
Proceedings of the EACL 2009 Workshop on the In-
teraction between Linguistics and Computational Lin-
guistics: Virtuous, Vicious or Vacuous?, pages 26–32,
Athens, Greece. ACL.
Coulmas, F. (2003). Writing systems: An introduction to
their linguistic analysis. Cambridge University Press.
De Saussure, F. (1989). Cours de linguistique g
´
en
´
erale:
´
Edition critique, volume 1. Otto Harrassowitz Verlag.
Devlin, J., Chang, M.-W., Lee, K., and Toutanova, K.
(2018). Bert: Pre-training of deep bidirectional trans-
formers for language understanding. arXiv preprint
arXiv:1810.04805.
Eldesouki, M., Dalvi, F., Sajjad, H., and Darwish, K.
(2016). QCRI @ DSL 2016: Spoken Arabic dialect
identification using textual features. In Proceedings
of the Third Workshop on NLP for Similar Languages,
Varieties and Dialects (VarDial3), pages 221–226,
Osaka, Japan. The COLING 2016 Organizing Com-
mittee.
Giwa, O. and Davel, M. H. (2014). Language identification
of individual words with joint sequence models. In
Interspeech 2014.
Holt, R. J., Stanaszek, M. J., and Stanaszek, W. F. (1998).
Understanding Medical Terms: A Guide for Phar-
macy Practice. CRC Press.
Jauhiainen, T. S., Lui, M., Zampieri, M., Baldwin, T., and
Lind
´
en, K. (2019). Automatic language identification
in texts: A survey. Journal of Artificial Intelligence
Research, 65:675–782.
Joulin, A., Grave, E., Bojanowski, P., Douze, M., J
´
egou,
H., and Mikolov, T. (2016). Fasttext. zip: Com-
pressing text classification models. arXiv preprint
arXiv:1612.03651.
Lee, J., Yoon, W., Kim, S., Kim, D., Kim, S., So, C. H.,
and Kang, J. (2019). Biobert: pre-trained biomedi-
cal language representation model for biomedical text
mining. arXiv preprint arXiv:1901.08746.
Lui, M. and Baldwin, T. (2012). langid. py: An off-the-
shelf language identification tool. In Proceedings of
the ACL 2012 system demonstrations, pages 25–30.
ACL.
Mandal, S., Das, S. D., and Das, D. (2018). Language
identification of bengali-english code-mixed data us-
ing character & phonetic based lstm models. arXiv
preprint arXiv:1803.03859.
Manousogiannis, E., Mesbah, S., Bozzon, A., Baez, S., and
Sips, R. J. (2019). Give it a shot: Few-shot learning
to normalize adr mentions in social media posts. In
Proceedings of the Fourth Social Media Mining for
Health Applications (# SMM4H), pages 114–116.
Molina, G., AlGhamdi, F., Ghoneim, M., Hawwari, A.,
Rey-Villamizar, N., Diab, M., and Solorio, T. (2016).
Overview for the second shared task on language iden-
tification in code-switched data. In Proceedings of
the Second Workshop on Computational Approaches
to Code Switching, pages 40–49. ACL.
Nguyen, D. and Cornips, L. (2016). Automatic detec-
tion of intra-word code-switching. In Proceedings of
the 14th SIGMORPHON Workshop on Computational
Research in Phonetics, Phonology, and Morphology,
pages 82–86.
Noreen, E. W. (1989). Computer-intensive methods for test-
ing hypotheses. Wiley New York.
Pershad, Y., Hangge, P. T., Albadawi, H., and Oklu, R.
(2018). Social medicine: Twitter in healthcare. Jour-
nal of clinical medicine, 7(6):121.
Pustejovsky, J. and Stubbs, A. (2012). Natural Language
Annotation for Machine Learning: A guide to corpus-
building for applications. ” O
´
Reilly Media, Inc.”.
Ruder, S., Vuli
´
c, I., and Søgaard, A. (2019). A survey of
cross-lingual word embedding models. Journal of Ar-
tificial Intelligence Research, 65:569–631.
Tromp, E. and Pechenizkiy, M. (2011). Graph-based n-
gram language identification on short texts. In Proc.
20th Machine Learning conference of Belgium and
The Netherlands, pages 27–34.
Van den Bercken, L., Sips, R.-J., and Lofi, C. (2019). Eval-
uating neural text simplification in the medical do-
main. In The World Wide Web Conference, pages
3286–3292. ACM.
Wehrmann, J., Becker, W. E., and Barros, R. C. (2018).
A multi-task neural network for multilingual senti-
ment classification and language detection on twitter.
In Proceedings of the 33rd Annual ACM Symposium
on Applied Computing - SAC 18, pages 1805–1812.
ACM Press.
Xia, M. X. (2016). Codeswitching language identification
using subword information enriched word vectors. In
Proceedings of The Second Workshop on Computa-
tional Approaches to Code Switching, pages 132–136.
Yin, Y. and Jin, Z. (2015). Document sentiment classifi-
cation based on the word embedding. In 2015 4th
International Conference on Mechatronics, Materials,
Chemistry and Computer Engineering. Atlantis Press.
Zsiga, E. C. (2012). The sounds of language: An introduc-
tion to phonetics and phonology. John Wiley & Sons.
HEALTHINF 2020 - 13th International Conference on Health Informatics
406