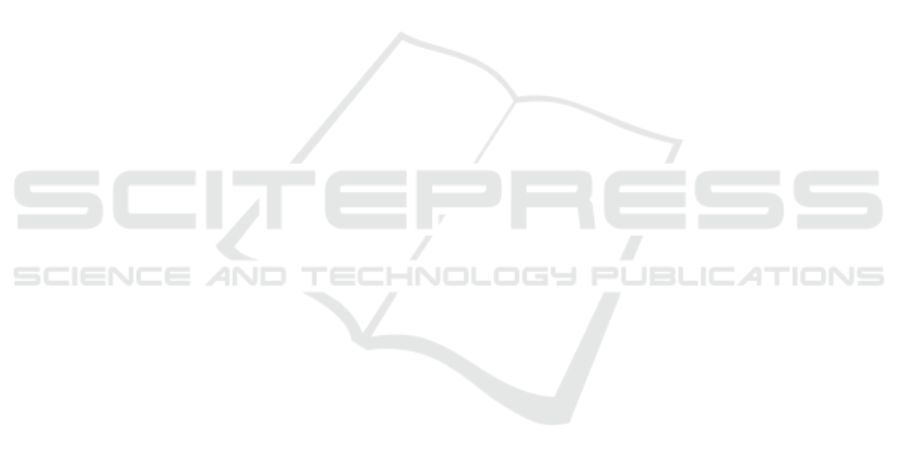
Elder, T. J., Sutton, R. M., and Douglas, K. M. (2005).
Keeping it to ourselves: Effects of audience size and
composition on reactions to criticisms of the ingroup.
Group Processes & Intergroup Relations, 8(3):231–
244.
Guadagno, R. E., Blascovich, J., Bailenson, J. N., and Mc-
Call, C. (2007). Virtual humans and persuasion: The
effects of agency and behavioral realism. Media Psy-
chology, 10(1):1–22.
Hamilton, A., Pan, X. S., Forbes, P., and Hale, J. (2016).
Using virtual characters to study human social cogni-
tion. In International Conference on Intelligent Vir-
tual Agents, pages 494–499. Springer.
Harris, S. R., Kemmerling, R. L., and North, M. M. (2002).
Brief virtual reality therapy for public speaking anxi-
ety. Cyberpsychology & behavior, 5(6):543–550.
Jacobs, M., Snow, J., Geraci, M., Vythilingam, M., Blair,
R., Charney, D. S., Pine, D. S., and Blair, K. S. (2008).
Association between level of emotional intelligence
and severity of anxiety in generalized social phobia.
Journal of anxiety disorders, 22(8):1487–1495.
Kim, H.-G., Cheon, E.-J., Bai, D.-S., Lee, Y. H., and Koo,
B.-H. (2018). Stress and heart rate variability: A meta-
analysis and review of the literature. Psychiatry inves-
tigation, 15(3):235.
Kusserow, M., Amft, O., and Tr
¨
oster, G. (2008). Analy-
sis of heart stress response for a public talk assistant
system. In European Conference on Ambient Intelli-
gence, pages 326–342. Springer.
Kyriakou, M. and Chrysanthou, Y. (2018). How responsive-
ness, group membership and gender affect the feeling
of presence in immersive virtual environments popu-
lated with virtual crowds. In Proceedings of the 11th
Annual International Conference on Motion, Interac-
tion, and Games, page 12. ACM.
Lindner, P., Miloff, A., Fagern
¨
as, S., Andersen, J., Sige-
man, M., Andersson, G., Furmark, T., and Carlbring,
P. (2019). Therapist-led and self-led one-session vir-
tual reality exposure therapy for public speaking anx-
iety with consumer hardware and software: A ran-
domized controlled trial. Journal of anxiety disorders,
61:45–54.
Nowak, K. L., Hamilton, M. A., and Hammond, C. C.
(2009). The effect of image features on judgments of
homophily, credibility, and intention to use as avatars
in future interactions. Media Psychology, 12(1):50–
76.
Nowak, K. L. and Rauh, C. (2005). The influence of
the avatar on online perceptions of anthropomor-
phism, androgyny, credibility, homophily, and attrac-
tion. Journal of Computer-Mediated Communication,
11(1):153–178.
Paladino, M.-P. and Castelli, L. (2008). On the immediate
consequences of intergroup categorization: Activation
of approach and avoidance motor behavior toward in-
group and outgroup members. Personality and Social
Psychology Bulletin, 34(6):755–768.
Pertaub, D.-P., Slater, M., and Barker, C. (2002). An exper-
iment on public speaking anxiety in response to three
different types of virtual audience. Presence: Teleop-
erators & Virtual Environments, 11(1):68–78.
Petrides, K. V. (2009). Psychometric properties of the
trait emotional intelligence questionnaire (teique).
In Assessing emotional intelligence, pages 85–101.
Springer.
Petrides, K. V. and Furnham, A. (2006). The role of trait
emotional intelligence in a gender-specific model of
organizational variables 1. Journal of Applied Social
Psychology, 36(2):552–569.
Pull, C. B. (2012). Current status of knowledge on public-
speaking anxiety. Current opinion in psychiatry,
25(1):32–38.
Salovey, P. E. and Sluyter, D. J. (1997). Emotional develop-
ment and emotional intelligence: Educational impli-
cations. Basic Books.
Sani, F., Madhok, V., Norbury, M., Dugard, P., and Wake-
field, J. R. (2015). Greater number of group identifica-
tions is associated with healthier behaviour: Evidence
from a scottish community sample. British Journal of
Health Psychology, 20(3):466–481.
Slater, M., Pertaub, D.-P., and Steed, A. (1999). Pub-
lic speaking in virtual reality: Facing an audience of
avatars. IEEE Computer Graphics and Applications,
19(2):6–9.
Stupar-Rutenfrans, S., Ketelaars, L. E., and van Gisber-
gen, M. S. (2017). Beat the fear of public speaking:
Mobile 360 video virtual reality exposure training in
home environment reduces public speaking anxiety.
Cyberpsychology, Behavior, and Social Networking,
20(10):624–633.
Summerfeldt, L. J., Kloosterman, P. H., Antony, M. M., and
Parker, J. D. (2006). Social anxiety, emotional intelli-
gence, and interpersonal adjustment. Journal of Psy-
chopathology and Behavioral Assessment, 28(1):57–
68.
Troxler, M., Qurashi, S., Tjon, D., Gao, H., Rombout, L.,
and Nalepa, G. (2018). The virtual hero: The influ-
ence of narrative on affect and presence in a vr game.
In CEUR Workshop Proceedings.
Vinayagamoorthy, V., Gillies, M., Steed, A., Tanguy, E.,
Pan, X., Loscos, C., and Slater, M. (2006). Building
expression into virtual characters building expression
into virtual characters. The Eurographics Association,
pages 1–42.
Wallach, H. S., Safir, M. P., and Bar-Zvi, M. (2009). Virtual
reality cognitive behavior therapy for public speaking
anxiety: a randomized clinical trial. Behavior modifi-
cation, 33(3):314–338.
Witmer, B. G. and Singer, M. J. (1998). Measuring pres-
ence in virtual environments: A presence question-
naire. Presence, 7(3):225–240.
Wittenbrink, B., Judd, C. M., and Park, B. (1997). Evi-
dence for racial prejudice at the implicit level and its
relationship with questionnaire measures. Journal of
personality and social psychology, 72(2):262.
HUCAPP 2020 - 4th International Conference on Human Computer Interaction Theory and Applications
24