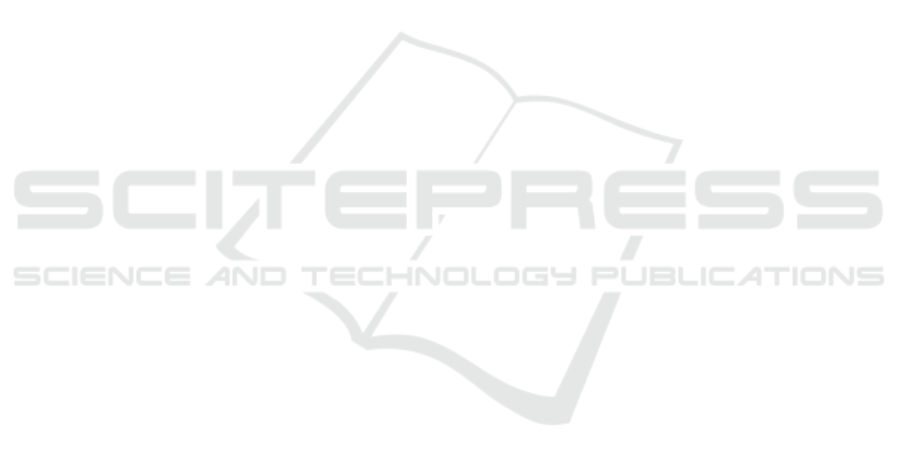
despite the type of image or question and even image
complexity indicates that the visualization system can
be used more broadly for understanding dialogues.
This is important for face-to-face collaborative appli-
cations such as a group of students collaborating on
a task in a classroom. In the future we would like to
analyze the benefits of providing only gaze informa-
tion without the most frequent words and compare it
to other modalities, isolated and combined.
Our visualization system is flexible and can be ex-
panded to include more features. For example, quan-
titative metrics such as mean and standard deviation
for fixation duration and type-token-ratio can also be
added in the future. In addition, if more human gener-
ated data is elicited, it could be added into the system
as new user features. Currently, the system displays
the static image that was used in Experiment I but it
can be extended to display a dynamic stimulus or 3D
real-world scenes. This is challenging and will be par-
ticularly helpful for researchers who want to analyze
data from wearable eye trackers.
Finally, we have shown that our discussion-based
multimodal data elicitation method can capture multi-
party reasoning behavior in visual environments. Our
framework is an important step toward meaningfully
visualizing and interpreting such multiparty multi-
modal data.
ACKNOWLEDGEMENTS
This material is based upon work supported by the
National Science Foundation under Award No. IIS-
1851591. Any opinions, findings, and conclusions
or recommendations expressed in this material are
those of the author(s) and do not necessarily reflect
the views of the National Science Foundation.
REFERENCES
Bird, S., Klein, E., and Loper, E. (2009). Natural Language
Processing with Python. O’Reilly Media, Inc., 1st edi-
tion.
Blascheck, T., John, M., Kurzhals, K., Koch, S., and Ertl, T.
(2016). Va2: A visual analytics approach for evaluat-
ing visual analytics applications. IEEE Transactions
on Visualization and Computer Graphics, 22(1):61–
70.
Blascheck, T., Kurzhals, K., Raschke, M., Burch, M.,
Weiskopf, D., and Ertl, T. (2014). State-of-the-art of
visualization for eye tracking data. In EuroVis.
Blascheck, T., Kurzhals, K., Raschke, M., Burch, M.,
Weiskopf, D., and Ertl, T. (2017). Visualization of
eye tracking data: A taxonomy and survey. Computer
Graphics Forum, 36(8):260–284.
Bull, S. and Kay, J. (2016). Smili: a framework for inter-
faces to learning data in open learner models, learning
analytics and related fields. International Journal of
Artificial Intelligence in Education, 26(1):293–331.
Carter, S. and Bailey, V. (2012). Facial Expressions :
Dynamic Patterns, Impairments and Social Percep-
tions. Hauppauge, N.Y. : Nova Science Publishers,
Inc. 2012.
Fry, B. and Reas, C. (2014). Processing: A Programming
Handbook for Visual Designers and Artists. The MIT
Press.
Gardner, M., Grus, J., Neumann, M., Tafjord, O., Dasigi, P.,
Liu, N. F., Peters, M., Schmitz, M., and Zettlemoyer,
L. (2018). AllenNLP: A deep semantic natural lan-
guage processing platform. pages 1–6.
Kon
´
e, M., May, M., and Iksal, S. (2018). Towards a
dynamic visualization of online collaborative learn-
ing. In Proceedings of the 10th International Con-
ference on Computer Supported Education - Volume
1: CSEDU,, pages 205–212. INSTICC, SciTePress.
Kontogiorgos, D., Avramova, V., Alexanderson, S., Jonell,
P., Oertel, C., Beskow, J., Skantze, G., and Gustafson,
J. (2018). A multimodal corpus for mutual gaze
and joint attention in multiparty situated interaction.
In Proceedings of the Eleventh International Confer-
ence on Language Resources and Evaluation (LREC-
2018), Miyazaki, Japan. European Languages Re-
sources Association (ELRA).
Lee, K., He, L., Lewis, M., and Zettlemoyer, L. (2017).
End-to-end neural coreference resolution. pages 188–
197.
Microsoft (2019). Microsoft Azure Speech to Text. Ac-
cessed: 2019-12-17.
R
¨
aih
¨
a, K.-J., Aula, A., Majaranta, P., Rantala, H., and
Koivunen, K. (2005). Static visualization of tempo-
ral eye-tracking data. In IFIP Conference on Human-
Computer Interaction, pages 946–949. Springer.
Ramloll, R., Trepagnier, C., Sebrechts, M., and Beedasy, J.
(2004). Gaze data visualization tools: Opportunities
and challenges. 2010 14th International Conference
Information Visualisation, 0:173–180.
Sharma, K. and Jermann, P. (2018). Gaze as a proxy for
cognition and communication. 2018 IEEE 18th In-
ternational Conference on Advanced Learning Tech-
nologies (ICALT), Advanced Learning Technologies
(ICALT), 2018 IEEE 18th International Conference
on, ICALT.
Soon, W. M., Ng, H. T., and Lim, D. C. Y. (2001). A ma-
chine learning approach to coreference resolution of
noun phrases. Comput. Linguist., 27(4):521–544.
Stellmach, S., Nacke, L. E., Dachselt, R., and Lindley, C. A.
(2010). Trends and techniques in visual gaze analysis.
CoRR, abs/1004.0258.
Tsai, T. J., Stolcke, A., and Slaney, M. (2015). Multimodal
addressee detection in multiparty dialogue systems. In
Proc. IEEE ICASSP, pages 2314–2318. IEEE - Insti-
tute of Electrical and Electronics Engineers.
Wang, R., Olson, B., Vaidyanathan, P., Bailey, R., and Alm,
C. (2019). Fusing dialogue and gaze from discussions
2d and 3d scenes. In Adjunct of the 2019 International
Conference on Multimodal Interaction (ICMI ’19 Ad-
junct), October 14–18, 2019, Suzhou, China. ACM,
New York, NY, USA 6 Pages.
Dynamic Visualization System for Gaze and Dialogue Data
145