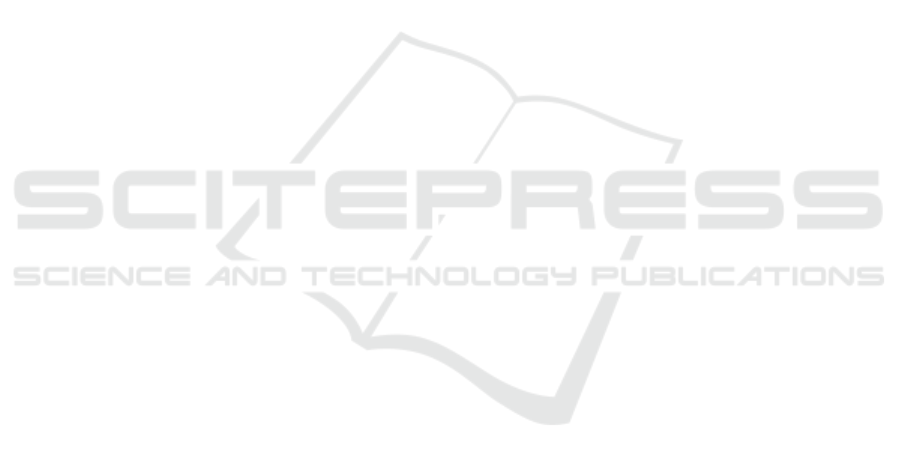
fusion approaches, e.g. using video recordings to pe-
riodically calibrate the system. Video recordings are
limited to defined camera angles however, that shall
not be a problem since during repetitive tasks we can
anticipate the action places and position the cameras
accordingly. Additionally, the explanation approach
can be increased through the analysis of three dimen-
sions of risk factors exposure: intensity, duration and
frequency.
REFERENCES
Battini, D., Persona, A., and Sgarbossa, F. (2014). Inno-
vative real-time system to integrate ergonomic evalu-
ations into warehouse design and management. Com-
puters & Industrial Engineering, 77:1 – 10.
Bauters, K., Cottyn, J., Claeys, D., Slembrouck, M., Vee-
laert, P., and van Landeghem, H. (2018). Auto-
mated work cycle classification and performance mea-
surement for manual work stations. Robotics and
Computer-Integrated Manufacturing, 51.
Beavers, I. (2017). The Case of the Misguided
Gyro. https://www.analog.com/en/analog-dialogue/
raqs/raq-issue-139.html. Accessed: 2019-12-05.
Cao, Z., Hidalgo, G., Simon, T., Wei, S.-E., and Sheikh,
Y. (2018). OpenPose: realtime multi-person 2D pose
estimation using Part Affinity Fields. In arXiv preprint
arXiv:1812.08008.
Caputo, F., Greco, A., D‘Amato, E., Notaro, I., and Spada,
S. (2019). IMU-Based Motion Capture Wearable Sys-
tem for Ergonomic Assessment in Industrial Environ-
ment. Springer Nature, pages 215–225.
Colton, S. (2007). The Balance Filter - A Simple Solution
for Integrating Accelerometer and Gyroscope Mea-
surements for a Balancing Platform.
Dam, E. B., Koch, M., and Lillholm, M. (1998). Quater-
nions, interpolation and animation. Technical Re-
port DIKU-TR-98/5, Department of Computer Sci-
ence University of Copenhagen, Universitets parken
1DK-2100Kbh, Denmark.
Filippeschi, A., Schmitz, N., Miezal, M., Bleser, G., Ruf-
faldi, E., and Stricker, D. (2017). Survey of motion
tracking methods based on inertial sensors: A focus
on upper limb human motion. Sensors, 17.
H. Seidel, D., Ditchen, D., Hoehne-H
¨
uckst
¨
adt, U., Rieger,
M., and Steinhilber, B. (2019). Quantitative measures
of physical risk factors associated with work-related
musculoskeletal disorders of the elbow: A systematic
review. International Journal of Environmental Re-
search and Public Health, 16:130.
Irastorza, E. S., Xabier, and Copsey, S. (2010). OSH in
figures: Work-related musculoskeletal disorders in the
EU — Facts and figures. European Agency for Safety
and Health at Work.
Lerner, G. M. (2012). Three axis attitude determination.
In Wertz, J., editor, Spacecraft Attitude Determination
and Control, volume 73 of Astrophysics and Space
Science Library, chapter 12, pages 420–435. Springer
Netherlands.
Luttmann, A., J
¨
ager, M., Griefahn, B., Caffier, G., and
Liebers, F. (2003). Preventing Musculoskeletal Dis-
orders in the Workplace. World Health Organization.
McAtamney, L. and Corlett, E. N. (1993). Rula: a sur-
vey method for the investigation of work-related up-
per limb disorders. Applied Ergonomics, 24(2):91 –
99.
Merriaux, P., Dupuis, Y., Boutteau, R., Vasseur, P., and Sa-
vatier, X. (2017). A study of vicon system positioning
performance. Sensors, 17(7).
Peppoloni, L., Filippeschi, A., Ruffaldi, E., and Avizzano,
C. (2016). A novel wearable system for the online
assessment of risk for biomechanical load in repeti-
tive efforts. International Journal of Industrial Er-
gonomics, 52:1 – 11. New Approaches and Interven-
tions to Prevent Work Related Musculoskeletal Disor-
ders.
Pereira, A., Guimar
˜
aes, V., and Sousa, I. (2017). Joint an-
gles tracking for rehabilitation at home using inertial
sensors: a feasibility study. In Proceedings of the 11th
EAI International Conference on Pervasive Comput-
ing Technologies for Healthcare, pages 146–154.
Romero, D., Stahre, J., Wuest, T., Noran, O., Bernus, P.,
Fasth, Fast-Berglund, A., and Gorecky, D. (2016). To-
wards an Operator 4.0 Typology: A Human-Centric
Perspective on the Fourth Industrial Revolution Tech-
nologies. In International conference on computers
and industrial engineering (CIE46) proceedings.
Uva, A., Carnide, F., Serranheira, F., Miranda, L., and
Lopes, M. (2008). Les
˜
oes m
´
usculoesquel
´
eticas rela-
cionadas com o trabalho-guia de orientac¸
˜
ao para a
prevenc¸
˜
ao. Direcc¸
˜
ao Geral da Sa
´
ude.
Valenti, R., Dryanovski, I., and Xiao, J. (2015). Keeping
a good attitude: A quaternion-based orientation filter
for imus and margs. Sensors, 15:19302–19330.
Varandas., R., Folgado., D., and Gamboa., H. (2019). Eval-
uation of spatial-temporal anomalies in the analysis of
human movement. In Proceedings of the 12th Inter-
national Joint Conference on Biomedical Engineering
Systems and Technologies - Volume 4: BIOSIGNALS,,
pages 163–170. INSTICC, SciTePress.
Vicon Motion Systems (2007). Upper Limb Model. Product
Guide. http://www.vicon.com.
Vignais, N., Bernard, F., Touvenot, G., and Sagot, J. C.
(2017). Physical risk factors identification based on
body sensor network combined to videotaping. Ap-
plied Ergonomics, 65.
Wang., H., Mohamed Refai, M., and van Beijnum., B. F.
(2019). Measuring upper-extremity use with one imu.
In Proceedings of the 12th International Joint Confer-
ence on Biomedical Engineering Systems and Tech-
nologies - Volume 4: BIOSIGNALS,, pages 93–100.
INSTICC, SciTePress.
BIOSIGNALS 2020 - 13th International Conference on Bio-inspired Systems and Signal Processing
88