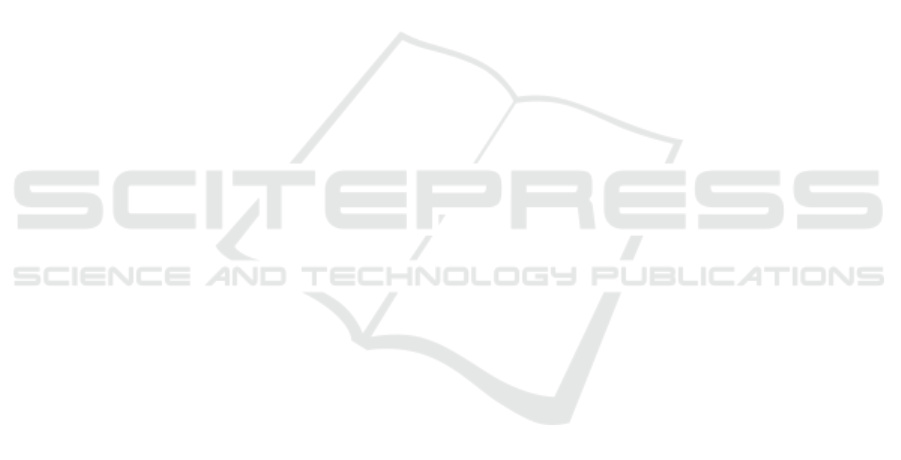
about human behaviour feature extraction than the re-
viewed literature. For instance, (Zheng et al., 2019)
and (Suzuki et al., 2007) only focuses in human tra-
jectory, (Tomforde et al., 2018) considers both loca-
tion and activities sequences but no time information
is extracted to model behaviour. This study extracts
an extensive list of features from human behaviour,
comprising time and frequency information from the
trajectories and activities of the user. The second part
investigates the usage of the extracted features for un-
derstanding and discovery of human patterns. Simi-
larly to the study conducted by Rahim (Rahim et al.,
2015), this study uses statistical models for the es-
timation of human behaviour patterns and anomaly
detection. However, instead of a gaussian distribu-
tion, this study uses a KDE to define the probabil-
ity density function since behaviour features may not
follow a gaussian distribution as it was verified on
the behaviour features acquired during this study. Fi-
nally, an anomaly detection algorithm was introduced
to detect abnormal behaviour. Experimental results
demonstrate the effectiveness of the proposed frame-
work that revealed an increase potential to learn be-
havioural patterns and detect anomalies considering
different case studies. This study may be a key insight
for monitoring elderly daily routines as well as mar-
keting analysis, security and tourism management.
Although the developed study revealed promising
results there is still room for improvement that can be
addressed in the future. Firstly, the framework should
be tested in more users, including different anomalous
behaviours. Then, the distance to the pattern can be
improved by a weight optimisation and parameteriza-
tion process based on the intrinsic characteristics of
each feature. This way, strongly correlated features
will have lower influence on the anomaly detection.
The weights should be learned according to real case
scenarios. For instance, it can be studied which fea-
tures are more affected considering different anoma-
lous behaviours, and the weights learned accordingly.
The framework can also be improved by an automatic
detection of the number of days needed to learn the
pattern. Finally, the smartphone used for data acqui-
sition should be replaced by a smartwatch or bracelet
containing the sensors embedded in a smartphone.
REFERENCES
Evans, D., Price, K., and Meyer, J. (2016). Home Alone
With Dementia. SAGE Open, 6(3):1–13.
Guimar
˜
aes, V., Castro, L., Carneiro, S., Monteiro, M.,
Rocha, T., Barandas, M., Machado, J., Vasconcelos,
M., Gamboa, H., and Elias, D. (2016). A motion
tracking solution for indoor localization using smart-
phones. In 2016 International Conference on Indoor
Positioning and Indoor Navigation, IPIN 2016, pages
1–8. IEEE.
Jaul, E. and Barron, J. (2017). Age-Related Diseases and
Clinical and Public Health implications for the 85
Years Old and Over Population. Frontiers in Public
Health, 5:1–7.
Jeebun, S., Ballgobin, R., and Al-ani, T. (2015). Optimal
Number of States in Hidden Markov Models and its
Application to the Detection of Human Movement.
University of Mauritius Research Journal, 21:438–
469.
Kumar, V., Tan, P.-T., and Steinbach, M. (2006). Cluster
analysis: basic concepts and algorithms. In Introduc-
tion to data mining, pages 488–568.
Parzen, E. (1962). On the Estimation of Probability Density
Functions and Mode. Ann. Math. Statist, 33:1065–
1076.
Rabiner, L. R. (1989). A Tuturial on Hidden Markov Mod-
els and Selected Applications in Speech Recognition.
Proceedings of the IEEE, 77(2):257–286.
Rahim, A., Forkan, M., Khalil, I., Tari, Z., Foufou, S.,
and Bouras, A. (2015). A context-aware approach
for long-term behavioural change detection and abnor-
mality prediction in ambient assisted living. Pattern
Recognition, 48(3):628–641.
Rosenblatt, M. (1956). A Central Limit Theorem and a
Strong Mixing Condition. Proceedings of the Na-
tional Academy of Sciences, 42(1):43–47.
Silverman, B. W. (1982). Kernel density estimation us-
ing the fast Fourier transform. Applied Statistics,
31(1):93–99.
Suzuki, N., Hirasawa, K., Tanaka, K., Kobayashi, Y., Sato,
Y., and Fujino, Y. (2007). Learning motion patterns
and anomaly detection by human trajectory analysis.
In Conference Proceedings - IEEE International Con-
ference on Systems, Man and Cybernetics, pages 498–
503.
Tomforde, S., Dehling, T., Haux, R., Huseljic, D., Kottke,
D., Scheerbaum, J., Sick, B., Sunyaev, A., and Wolf,
K. H. (2018). Towards Proactive Health-enabling Liv-
ing Environments: Simulation-based Study and Re-
search Challenges. In 31th International Conference
on Architecture of Computing Systems, pages 1–8.
United Nations (2017). World Population Ageing 2017 -
Highlights (ST/ESA(SER.A/397). Technical report,
Department of Economic and Social Affairs, Popula-
tion Division, New York.
Zhang, D. (2018). Periodic Pattern Mining from Spatio-
temporal Trajectory Data. PhD thesis, James Cook
University.
Zheng, W., Zhou, R., Zhang, Z., Zhong, Y., Wang, S., Wei,
Z., and Ji, H. (2019). Understanding the tourist mobil-
ity using GPS: How similar are the tourists? Tourism
Management, 71:54–66.
Learning Human Behaviour Patterns by Trajectory and Activity Recognition
227