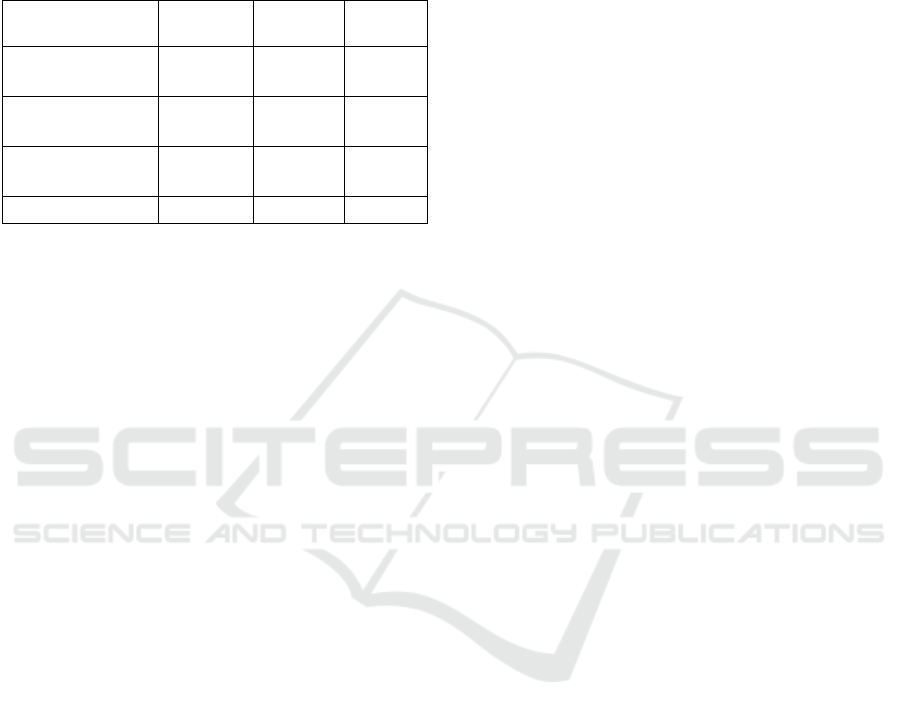
applied to HEp-2 images. In particular, the fine-
tuning strategy proved to be more effective of the
CNN layers features extraction strategy, both in
accuracy and in AUC, of about 1%.
Table 4: Performance comparison between intensity
classification methods on HEp-2 images.
Di Cataldo
(Di Cataldo, 2016)
Benammar
(Benammar, 2016)
4 CONCLUSIONS
In this paper a method for the automatic classification
of fluorescence intensity in HEp-2 images was
presented. This classification is very important for a
correct diagnosis of autoimmune diseases. A method
that uses the well-known GoogLeNet pre-trained
network has been presented. The potential of the
network has been analysed, with a view to optimizing
the classification process, with two strategies: as
feature extractors, in combination with the traditional
SVM classifier, and as classifiers after an appropriate
fine-tuning process. Different levels of freezing were
analysed and the improvement in performance of the
data augmentation was evaluated. The method, which
was developed and tested using a public database,
showed high classification performance: AUC of
98.4%. A comparison with other works of the state of
the art reveals the goodness of the proposed method
and the capabilities of GoogLeNet in the
classification of HEp-2 type images.
In the future, we planned to investigate the use of
ad-hoc CNN architectures instead of pre-trained
CNN. In addition, a study is in progress using several
pre-trained networks in order to identify the best
configuration for the HEp-2 image intensity analysis.
REFERENCES
Benammar, A., et al, 2016. Computer-assisted
classification patterns in autoimmune diagnostics: the
AIDA project.BioMed research international.
Bengio, Y., et al, 2012.Pratical Recommendations for
Gradient-Based Training of Deep Architectures.
Springer Berlin Heidelberg, pp. 437-478.
Cascio, D., et al, 2016. A multi-process system for HEp-2
cells classification based on SVM. Pattern Recogn.
Lett., 82, 56-63.
Cascio, D., et al, 2019. An Automatic HEp-2 Specimen
Analysis System Based on an Active Contours Model
and an SVM Classification.Applied Sciences, vol. 9, pp.
307.
Cascio, D., et al, 2019. Deep Convolutional Neural
Network for HEp-2 Fluorescence Intensity
Classification. Applied Sciences.
Cascio, D., et al, 2019. Deep CNN for IIF Images
Classification in Autoimmune Diagnostics.Applied
Sciences, vol. 9, pp. 1618.
Di Cataldo, S., et al, 2016. ANAlyte: A modular image
analysis tool for ANA testing with indirect
immunofluorescence. Computer methods and
programs in biomedicine, vol. 128, pp. 86-99.
Foggia, P., et al, 2014. Pattern recognition in stained HEp-
2 cells: Where are we now?.Pattern Recognition, 47,
2305-2314.
Gao, Z., et al, 2014. Experimental study of unsupervised
feature learning for HEp-2 cell images clustering.
InDICTA International Conference on Digital Image
Computing: Techniques and Applications.
Gao, Z., et al, 2016. HEp-2 cell image classification with
deep convolutional neural networks.IEEE Journal of
Biomedical and Health Informatics, vol. 21, pp. 416-
428.
Hobson, P., et al, 2016.Computer Aided Diagnosis for Anti-
NuclearAntibodies HEp-2 images: Progress and
challenges. Pattern Recognition Letters, vol. 82, pp. 3-
11.
Iacomi M., et al, 2014. Mammographic images
segmentation based on chaotic map clustering
algorithm. BMC Medical imaging, vol. 14.
Li, H., et al, 2016. HEp-2 specimen classification via deep
CNNs and pattern histogram. In ICPR 2016, 23rd
International Conference on Pattern Recognition.
Manivannan, S., et al, 2016. An automated pattern
recognition system for classifying indirect
immunofluorescence images of HEp-2 cells and
specimens. Pattern Recognition, 51, 12-26.
Merone, M., et al, 2019. A computer-aided diagnosis
system for HEp-2 fluorescence intensity
classification.Artificial intelligence in medicine, vol.
97, pp. 71-78.
Murphy, K. P., 2012. Machine Learning: A Probabilistic
Perspective. The MIT Press, Cambridge,
Massachusetts.
Oraibi, Z., et al, 2018.Learning Local and Deep Features for
Efficient Cell Image Classification Using Random
Forests. In ICIP 2018, 25th International Conference
on Image Processing.
Perner, P., et al, 2002.Mining knowledge for Hep-2 cell
image classification. Artificial Intelligence in Medicine,
26 (1-2), pp. 161-173.
BIOIMAGING 2020 - 7th International Conference on Bioimaging
148