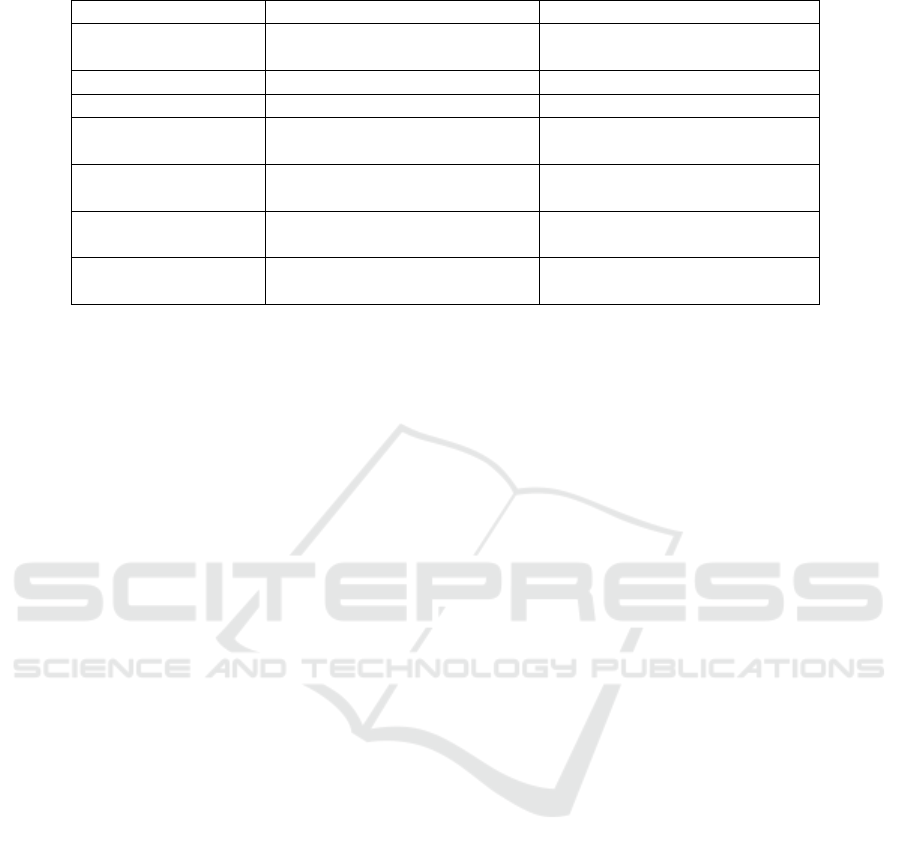
Table 3: Evaluation of the proposed method comparatively to the other methods (unit: %).
Methods Accuracy
Ozyilmaz et al(2002) Conic Section Function Neural
Network
88.3
Keles et al(2008) neuro fuzzy Classification 95.33
Iakovidis et al(2010) fuzzy local binary pattern 97.5
Acharya et Al(2011) Discrete Wavelet Transform
(DWT) and texture parameters
98
Acharya et Al(2017) Two-Threshold Binary Decom-
position algorithm
97.52
Chi et al(2017) deep learning features extrac-
tion
98.29
Proposed methods Shearlet Transform and
Generic Fourier Descriptor
98.51
quired from the laboratory CIM @ LAB. The classifi-
cation performance of textural feature has also been
optimized by the RASER dimensionality reduction
method. A comparative study shows that the metrics
performance is better with the application of feature
selection step. In addition, GFD was used to extract
the boundary information. Finally, the combination of
texture feature obtained with shearlet decomposition
and boundary information acquired using GFD give
the highest classification performance.
Thus, in our future work, we want to propose an
automated CAD system for detection and classifica-
tion of thyroid nodules.We aim also to study and pro-
pose other feature selection methods.
REFERENCES
(2017). Uci repository of machine learning databases, uni-
versity of columbia at irvine.
Abbasian Ardakani, A., Reiazi, R., and Mohammadi, A.
(2018). A clinical decision support system using ultra-
sound textures and radiologic features to distinguish
metastasis from tumor-free cervical lymph nodes in
patients with papillary thyroid carcinoma. Journal of
Ultrasound in Medicine, 37(11):2527–2535.
Acharya, U. R., Faust, O., Sree, S. V., Molinari, F., Gar-
beroglio, R., and Suri, J. (2011). Cost-effective and
non-invasive automated benign & malignant thyroid
lesion classification in 3d contrast-enhanced ultra-
sound using combination of wavelets and textures: a
class of thyroscan
TM
algorithms. Technology in can-
cer research & treatment, 10(4):371–380.
Acharya, U. R., Sree, S. V., Krishnan, M. M. R., Moli-
nari, F., Garberoglio, R., and Suri, J. S. (2012). Non-
invasive automated 3d thyroid lesion classification in
ultrasound: a class of thyroscan
TM
systems. Ultrason-
ics, 52(4):508–520.
Chi, J., Walia, E., Babyn, P., Wang, J., Groot, G., and
Eramian, M. (2017). Thyroid nodule classification
in ultrasound images by fine-tuning deep convolu-
tional neural network. Journal of digital imaging,
30(4):477–486.
Easley, G., Labate, D., and Lim, W.-Q. (2008a). Sparse
directional image representations using the discrete
shearlet transform. Applied and Computational Har-
monic Analysis, 25(1):25–46.
Easley, G., Labate, D., and Lim, W.-Q. (2008b). Sparse
directional image representations using the discrete
shearlet transform. Applied and Computational Har-
monic Analysis, 25(1):25–46.
Guo, K., Kutyniok, G., and Labate, D. (2006). Sparse multi-
dimensional representations using anisotropic dilation
and shear operators.
Iakovidis, D. K., Keramidas, E. G., and Maroulis, D.
(2010). Fusion of fuzzy statistical distributions for
classification of thyroid ultrasound patterns. Artificial
Intelligence in Medicine, 50(1):33–41.
Keles¸, A. and Keles¸, A. (2008). Estdd: Expert system for
thyroid diseases diagnosis. Expert Systems with Ap-
plications, 34(1):242–246.
Labate, D., Lim, W.-Q., Kutyniok, G., and Weiss, G.
(2005). Sparse multidimensional representation using
shearlets. In Wavelets XI, volume 5914, page 59140U.
International Society for Optics and Photonics.
Noura, A., Shili, H., and Romdhane, L. B. (2016). Reliable
attribute selection based on random forest (raser). In
International Conference on Intelligent Systems De-
sign and Applications, pages 11–24. Springer.
Ozyilmaz, L. and Yildirim, T. (2002). Diagnosis of thy-
roid disease using artificial neural network methods.
In Proceedings of the 9th International Conference on
Neural Information Processing, 2002. ICONIP’02.,
volume 4, pages 2033–2036. IEEE.
Raghavendra, U., Acharya, U. R., Gudigar, A., Tan, J. H.,
Fujita, H., Hagiwara, Y., Molinari, F., Kongmebhol,
P., and Ng, K. H. (2017). Fusion of spatial gray level
dependency and fractal texture features for the charac-
terization of thyroid lesions. Ultrasonics, 77:110–120.
Zhang, D. and Lu, G. (2002). Generic fourier descriptor for
shape-based image retrieval. In Proceedings. IEEE In-
ternational Conference on Multimedia and Expo, vol-
ume 1, pages 425–428. IEEE.
VISAPP 2020 - 15th International Conference on Computer Vision Theory and Applications
298