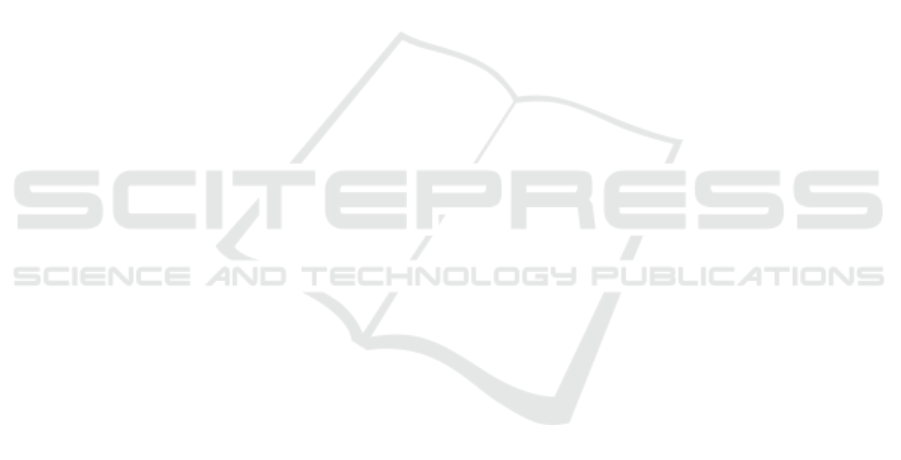
ACKNOWLEDGEMENTS
This research was supported by the PRECISE4Q
project, funded through the European Union’s Hori-
zon 2020 research and innovation program under
grant agreement No. 777107, and the ADAPT Re-
search Centre, funded by Science Foundation Ireland
(Grant 13/RC/2106) and is co-funded by the Euro-
pean Regional Development fund.
REFERENCES
Asadi, H., Dowling, R., Yan, B., and Mitchell, P. (2014).
Machine Learning for Outcome Prediction of Acute
Ischemic Stroke Post Intra-Arterial Therapy. PLoS
ONE, 9(2):e88225.
Bacchi, S., Zerner, T., Oakden-Rayner, L., Kleinig, T., Pa-
tel, S., and Jannes, J. (2019). Deep Learning in the
Prediction of Ischaemic Stroke Thrombolysis Func-
tional Outcomes: A Pilot Study. Academic Radiology,
pages 1–5.
Baltrusaitis, T., Ahuja, C., and Morency, L. P. (2019). Mul-
timodal Machine Learning: A Survey and Taxonomy.
IEEE Transactions on Pattern Analysis and Machine
Intelligence, 41(2):423–443.
Cui, H., Wang, X., Bian, Y., Song, S., and Feng, D. D.
(2018). Ischemic stroke clinical outcome prediction
based on image signature selection from multimodal-
ity data. In 2018 40th Annual International Confer-
ence of the IEEE Engineering in Medicine and Biol-
ogy Society (EMBC), pages 722–725. IEEE.
Dharmasaroja, P. and Dharmasaroja, P. A. (2012). Pre-
diction of intracerebral hemorrhage following throm-
bolytic therapy for acute ischemic stroke using multi-
ple artificial neural networks. Neurological Research,
34(2):120–128.
Esmaeilzadeh, S., Belivanis, D. I., Pohl, K. M., and Adeli,
E. (2018). End-To-End Alzheimer’s Disease Diagno-
sis and Biomarker Identification. pages 337–345.
Heo, J., Yoon, J. G., Park, H., Kim, Y. D., Nam, H. S., and
Heo, J. H. (2019). Machine Learning–Based Model
for Prediction of Outcomes in Acute Stroke. Stroke,
50(5):1263–1265.
Hilbert, A., Ramos, L. A., van Os, H. J., Olabarriaga, S. D.,
Tolhuisen, M. L., Wermer, M. J., Barros, R. S., van der
Schaaf, I., Dippel, D., Roos, Y. B., van Zwam, W. H.,
Yoo, A. J., Emmer, B. J., Lycklama
`
a Nijeholt, G. J.,
Zwinderman, A. H., Strijkers, G. J., Majoie, C. B., and
Marquering, H. A. (2019). Data-efficient deep learn-
ing of radiological image data for outcome prediction
after endovascular treatment of patients with acute is-
chemic stroke. Computers in Biology and Medicine,
115(October):103516.
Hotter, B., Pittl, S., Ebinger, M., Oepen, G., Jegzentis, K.,
Kudo, K., Rozanski, M., Schmidt, W. U., Brunecker,
P., Xu, C., Martus, P., Endres, M., Jungeh
¨
ulsing, G. J.,
Villringer, A., and Fiebach, J. B. (2009). Prospective
study on the mismatch concept in acute stroke patients
within the first 24 h after symptom onset - 1000Plus
study. BMC Neurology, 9(1):60.
Johnston, K. C., Wagner, D. P., Haley, E. C., and Connors,
A. F. (2002). Combined Clinical and Imaging Infor-
mation as an Early Stroke Outcome Measure. Stroke,
33(2):466–472.
Kelleher, J. D. (2019). Deep Learning. MIT Press.
Kim, E. and McCoy, K. F. (2018). Multi modal deep
learning using images and text for information graphic
classification. ASSETS 2018 - Proceedings of the 20th
International ACM SIGACCESS Conference on Com-
puters and Accessibility, pages 143–148.
Mouzannar, H., Rizk, Y., and Awad, M. (2018). Dam-
age Identification in Social Media Posts Using Mul-
timodal Deep Learning. Proceedings of the Inter-
national ISCRAM Conference, 2018-May(May):529–
543.
Nielsen, A., Hansen, M. B., Tietze, A., and Mouridsen, K.
(2018). Prediction of Tissue Outcome and Assessment
of Treatment Effect in Acute Ischemic Stroke Using
Deep Learning. Stroke, 49(6):1394–1401.
Oramas, S., Nieto, O., Sordo, M., and Serra, X. (2017). A
deep multimodal approach for cold-start music recom-
mendation. ACM International Conference Proceed-
ing Series, Part F1301:32–37.
Pinto, A., Mckinley, R., Alves, V., Wiest, R., Silva, C. A.,
and Reyes, M. (2018). Stroke Lesion Outcome Predic-
tion Based on MRI Imaging Combined With Clinical
Information. Frontiers in Neurology, 9.
Poria, S., Cambria, E., Bajpai, R., and Hussain, A. (2017).
A review of affective computing: From unimodal
analysis to multimodal fusion. Information Fusion,
37:98–125.
Ruder, S. (2016). An overview of gradient descent opti-
mization algorithms.
Slizovskaia, O., Gomez, E., and Haro, G. (2017). Mu-
sical instrument recognition in user-generated videos
using a multimodal convolutional neural network ar-
chitecture. ICMR 2017 - Proceedings of the 2017
ACM International Conference on Multimedia Re-
trieval, pages 226–232.
Vora, N. A., Shook, S. J., Schumacher, H. C., Tievsky,
A. L., Albers, G. W., Wechsler, L. R., and Gupta, R.
(2011). A 5-Item Scale to Predict Stroke Outcome Af-
ter Cortical Middle Cerebral Artery Territory Infarc-
tion. Stroke, 42(3):645–649.
Wang, D., Mao, K., and Ng, G.-W. (2017). Convolutional
neural networks and multimodal fusion for text aided
image classification. In 2017 20th International Con-
ference on Information Fusion (Fusion), pages 1–7.
IEEE.
Whiteley, W. N., Slot, K. B., Fernandes, P., Sandercock,
P., and Wardlaw, J. (2012). Risk Factors for Intracra-
nial Hemorrhage in Acute Ischemic Stroke Patients
Treated With Recombinant Tissue Plasminogen Ac-
tivator. Stroke, 43(11):2904–2909.
Wouters, A., Nysten, C., Thijs, V., and Lemmens, R. (2018).
Prediction of Outcome in Patients With Acute Is-
chemic Stroke Based on Initial Severity and Improve-
ment in the First 24 h. Frontiers in Neurology, 9.
HEALTHINF 2020 - 13th International Conference on Health Informatics
428