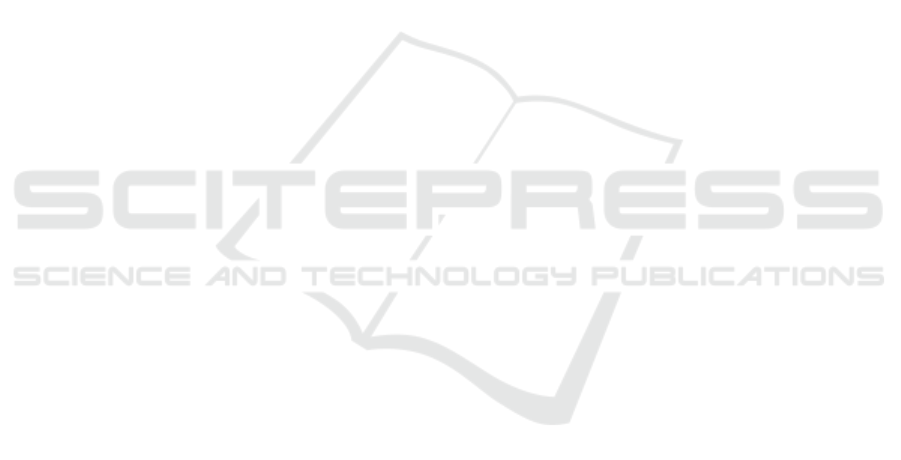
icance of our approach, including a per class analysis.
Our approach did not surpass some state-of-the-art
methods, mainly due to restricted information of the
used datasets. However, our results showed that our
data augmentation might improve HAR accuracy. To
achieve more competitive results, in future works, we
intend to explore the complementarity of our multi-
stream architecture with other features, such as IDT
(Wang et al., 2013) and I3D (Carreira and Zisserman,
2017). In addition, the SEVR principles could also be
employed to 3D CNNs for video classification prob-
lems.
ACKNOWLEDGEMENTS
Authors thank CAPES, FAPEMIG (grant CEX-
APQ-01744-15), FAPESP (grants #2017/09160-1 and
#2017/12646-3), CNPq (grant #305169/2015-7) for
the financial support, and NVIDIA Corporation for
the donation of two Titan Xp (GPU Grant Program).
REFERENCES
Carreira, J. and Zisserman, A. (2017). Quo Vadis, Action
Recognition? A New Model and the Kinetics Dataset.
In IEEE Conference on Computer Vision and Pattern
Recognition, pages 4724–4733.
Choutas, V., Weinzaepfel, P., Revaud, J., and Schmid, C.
(2018). Potion: Pose motion representation for action
recognition. In IEEE Conference on Computer Vision
and Pattern Recognition.
Concha, D. T., Maia, H. D. A., Pedrini, H., Tacon, H., Brito,
A. D. S., Chaves, H. D. L., and Vieira, M. B. (2018).
Multi-stream convolutional neural networks for action
recognition in video sequences based on adaptive vi-
sual rhythms. In IEEE International Conference on
Machine Learning and Applications, pages 473 – 480.
Deng, J., Dong, W., Socher, R., Li, L.-J., Li, K., and Fei-
Fei, L. (2009). ImageNet: A Large-Scale Hierarchical
Image Database. In IEEE Conference on Computer
Vision and Pattern Recognition.
Diba, A., Sharma, V., and Van Gool, L. (2017). Deep tem-
poral linear encoding networks. In IEEE Conference
on Computer Vision and Pattern Recognition, pages
2329–2338.
Horn, B. K. and Schunck, B. G. (1981). Determining Opti-
cal Flow. Artificial intelligence, 17(1-3):185–203.
Kong, Y. and Fu, Y. (2018). Human action recog-
nition and prediction: A survey. arXiv preprint
arXiv:1806.11230.
Krizhevsky, A., Sutskever, I., and Hinton, G. E. (2012). Im-
agenet Classification with Deep Convolutional Neural
Networks. In Advances in Neural Information Pro-
cessing Systems, pages 1097–1105.
Ngo, C.-W., Pong, T.-C., and Chin, R. T. (1999). Detection
of Gradual Transitions through Temporal Slice Anal-
ysis. In IEEE Computer Society Conference on Com-
puter Vision and Pattern Recognition, volume 1, pages
36–41.
Simonyan, K. and Zisserman, A. (2014). Two-Stream
Convolutional Networks for Action Recognition in
Videos. In Advances in Neural Information Process-
ing Systems, pages 568–576.
Sun, S., Kuang, Z., Sheng, L., Ouyang, W., and Zhang, W.
(2018). Optical flow guided feature: a fast and ro-
bust motion representation for video action recogni-
tion. In IEEE Conference on Computer Vision and
Pattern Recognition, pages 1390–1399.
Tacon, H., Brito, A. S., Chaves, H. L., Vieira, M. B., Vil-
lela, S. M., de Almeida Maia, H., Concha, D. T., and
Pedrini, H. (2019). Human action recognition using
convolutional neural networks with symmetric time
extension of visual rhythms. In International Confer-
ence on Computational Science and Its Applications,
pages 351–366. Springer.
Wang, H., Kl
¨
aser, A., Schmid, C., and Liu, C.-L. (2013).
Dense trajectories and motion boundary descriptors
for action recognition. International Journal of Com-
puter Vision, 103(1):60–79.
Wang, H. and Schmid, C. (2013). Action Recognition with
Improved Trajectories. In IEEE International Confer-
ence on Computer Vision, pages 3551–3558.
Wang, H., Yang, Y., Yang, E., and Deng, C. (2017). Explor-
ing Hybrid Spatio-Temporal Convolutional Networks
for Human Action Recognition. Multimedia Tools and
Applications, 76(13):15065–15081.
Wang, L., Qiao, Y., and Tang, X. (2015a). Action recog-
nition with trajectory-pooled deep-convolutional de-
scriptors. In IEEE Conference on Computer Vision
and Pattern Recognition, pages 4305–4314.
Wang, L., Xiong, Y., Wang, Z., and Qiao, Y. (2015b). To-
wards Good Practices for very Deep Two-Stream Con-
vnets. arXiv preprint arXiv:1507.02159.
Wang, L., Xiong, Y., Wang, Z., Qiao, Y., Lin, D., Tang,
X., and Van Gool, L. (2016). Temporal Segment
Networks: Towards Good Practices for Deep Action
Recognition. In European Conference on Computer
Vision, pages 20–36. Springer.
Zach, C., Pock, T., and Bischof, H. (2007). A dual-
ity based approach for realtime tv-l 1 optical flow.
In Joint Pattern Recognition Symposium, pages 214–
223. Springer.
Zhu, J., Zhu, Z., and Zou, W. (2018). End-to-end video-
level representation learning for action recognition.
In 24th International Conference on Pattern Recog-
nition, pages 645–650.
VISAPP 2020 - 15th International Conference on Computer Vision Theory and Applications
358