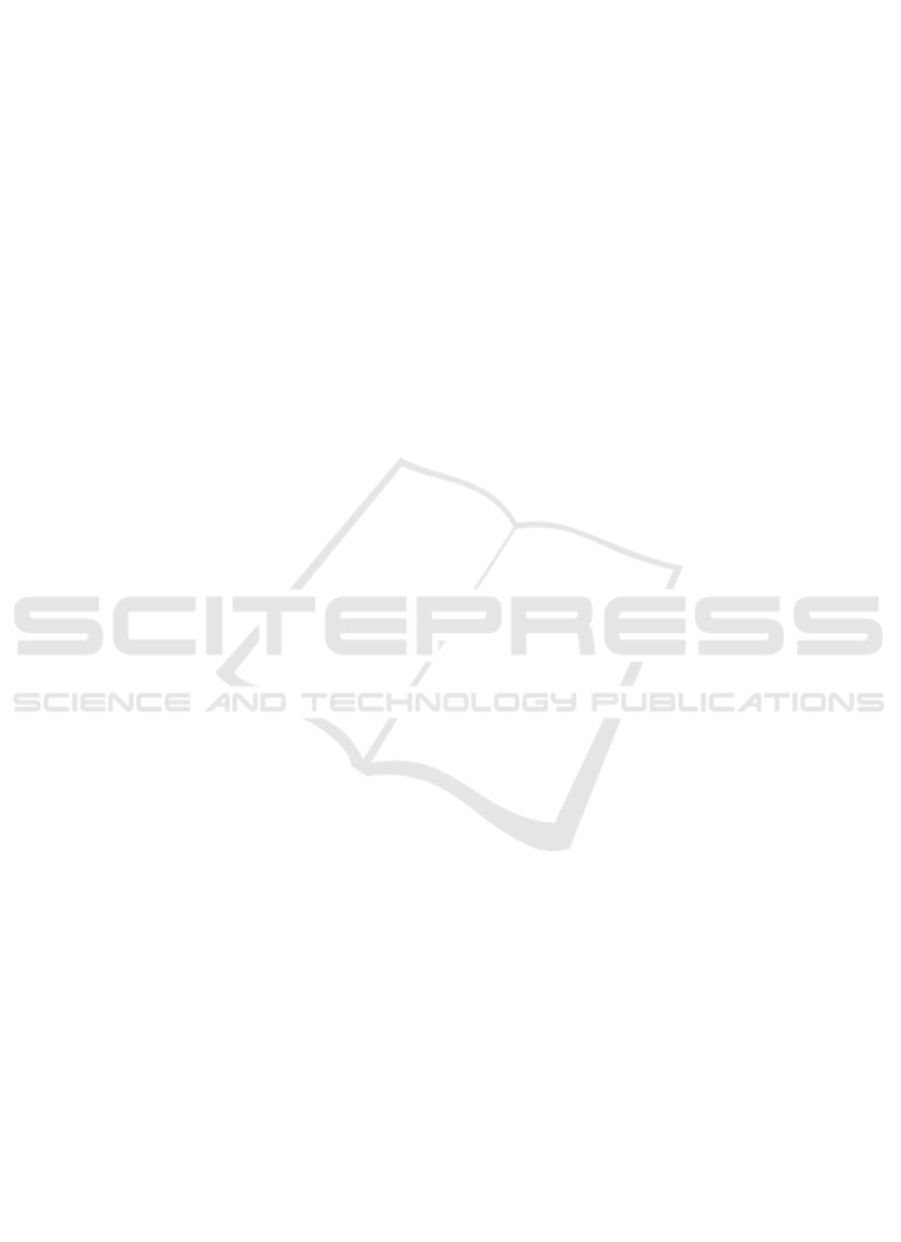
Davidson, M. (2014). Roland-Morris disability
questionnaire. Encyclopedia of Quality of Life and
Well-Being Research, 5587-5590.
Dongen van, J. M., van Hooff, M. L., Spruit, M., de
Kleuver, M., & Ostelo, R. W. (2017). Which patient-
reported factors predict referral to spinal surgery? A
cohort study among 4987 chronic low back pain
patients. European Spine Journal, 26(11), 2782-2788.
Dougherty, J., Kohavi, R., & Sahami, M. (1995).
Supervised and unsupervised discretization of
continuous features. In Machine Learning Proceedings
1995 (pp. 194-202). Morgan Kaufmann.
Dua, S., Acharya, U. R., & Dua, P. (Eds.). (2014). Machine
learning in healthcare informatics (Vol. 56). Berlin:
Springer.
Dueck, A. C., Mendoza, T. R., Mitchell, S. A., Reeve, B.
B., Castro, K. M., Rogak, L. J., ... & Li, Y. (2015).
Validity and reliability of the US National Cancer
Institute’s patient-reported outcomes version of the
common terminology criteria for adverse events (PRO-
CTCAE). JAMA oncology, 1(8), 1051-1059.
Fleiss, J. L., & Cohen, J. (1973). The equivalence of
weighted kappa and the intraclass correlation
coefficient as measures of reliability. Educational and
psychological measurement, 33(3), 613-619.
Gross, D. P., Armijo-Olivo, S., Shaw, W. S., Williams-
Whitt, K., Shaw, N. T., Hartvigsen, J., ... & Steenstra,
I. A. (2016). Clinical decision support tools for
selecting interventions for patients with disabling
musculoskeletal disorders: a scoping review. Journal of
occupational rehabilitation, 26(3), 286-318.
Hall, M., Frank, E., Holmes, G., Pfahringer, B., Reutemann,
P., & Witten, I. H. (2009). The WEKA data mining
software: an update. ACM SIGKDD explorations
newsletter, 11(1), 10-18.
Han, J., Pei, J., & Kamber, M. (2011). Data mining:
concepts and techniques. Elsevier.
Hill, J. C., Whitehurst, D. G., Lewis, M., Bryan, S., Dunn,
K. M., Foster, N. E., ... & Sowden, G. (2011).
Comparison of stratified primary care management for
low back pain with current best practice (STarT Back):
a randomised controlled trial. The Lancet, 378(9802),
1560-1571.
Hooff van, M. L., van Dongen, J. M., Coupé, V. M., Spruit,
M., Ostelo, R. W., & de Kleuver, M. (2018). Can
patient-reported profiles avoid unnecessary referral to a
spine surgeon? An observational study to further
develop the Nijmegen Decision Tool for Chronic Low
Back Pain. PloS one, 13(9), e0203518..
Hoy, D., Brooks, P., Blyth, F., & Buchbinder, R. (2010).
The epidemiology of low back pain. Best practice &
research Clinical rheumatology, 24(6), 769-781.
Koes, B. W., Van Tulder, M., Lin, C. W. C., Macedo, L. G.,
McAuley, J., & Maher, C. (2010). An updated overview
of clinical guidelines for the management of non-
specific low back pain in primary care. European Spine
Journal, 19(12), 2075-2094.
Kool, M., Bastiaannet, E., Van de Velde, C. J., & Marang-
van de Mheen, P. J. (2018). Reliability of Self-reported
Treatment Data by Patients With Breast Cancer
Compared With Medical Record Data. Clinical breast
cancer, 18(3), 234-238.
Landis, J. R., & Koch, G. G. (1977). The measurement of
observer agreement for categorical data. biometrics,
159-174.
Ling, C. X., & Sheng, V. S. (2010). Cost-sensitive
learning. Encyclopedia of machine learning, 231-235.
Linton, S. J., & Boersma, K. (2003). Early identification of
patients at risk of developing a persistent back problem:
the predictive validity of the Örebro Musculoskeletal
Pain Questionnaire. The Clinical journal of pain, 19(2),
80-86.
López, V., Fernández, A., García, S., Palade, V., & Herrera,
F. (2013). An insight into classification with
imbalanced data: Empirical results and current trends
on using data intrinsic characteristics. Information
sciences, 250, 113-141.
Oyeyemi, A. O., & Scott, P. (2018). Interoperability in
health and social care: organizational issues are the
biggest challenge. Journal of innovation in health
informatics, 25(3), 196-198.
Patel, S., Hee, S. W., Mistry, D., Jordan, J., Brown, S.,
Dritsaki, M., ... & Madan, J. (2016). Identifying back
pain subgroups: developing and applying approaches
using individual patient data collected within clinical
trials. Programme Grants for Applied Research, 4(10).
Rice, M. E., & Harris, G. T. (2005). Comparing effect sizes
in follow-up studies: ROC Area, Cohen's d, and r. Law
and human behavior, 29(5), 615-620.
Shafique, U., & Qaiser, H. (2014). A comparative study of
data mining process models (KDD, CRISP-DM and
SEMMA). International Journal of Innovation and
Scientific Research, 12(1), 217-222.
Shortliffe, E. H., & Sepúlveda, M. J. (2018). Clinical
decision support in the era of artificial
intelligence. Jama, 320(21), 2199-2200.
Simpson, A. K., Cholewicki, J., & Grauer, J. (2006).
Chronic low back pain. Current pain and headache
reports, 10(6), 431-436.
Sterne, J. A., White, I. R., Carlin, J. B., Spratt, M., Royston,
P., Kenward, M. G., ... & Carpenter, J. R. (2009).
Multiple imputation for missing data in
epidemiological and clinical research: potential and
pitfalls. Bmj, 338, b2393.
Szende, A. G. O. T. A. (2007). EQ-5D value sets:
inventory, comparative review and user guide. M.
Oppe, & N. J. Devlin (Eds.). Berlin, Germany::
Springer.
Tait, R. C., Chibnall, J. T., & Krause, S. (1990). The pain
disability index: psychometric properties. Pain, 40(2),
171-182.
Widerström, B., Olofsson, N., Boström, C., & Rasmussen-
Barr, E. (2016). Feasibility of the subgroup criteria
included in the treatment-strategy-based (TREST)
classification system (CS) for patients with non-
specific low back pain (NSLBP). Manual therapy, 23,
90-97.
HEALTHINF 2020 - 13th International Conference on Health Informatics
124