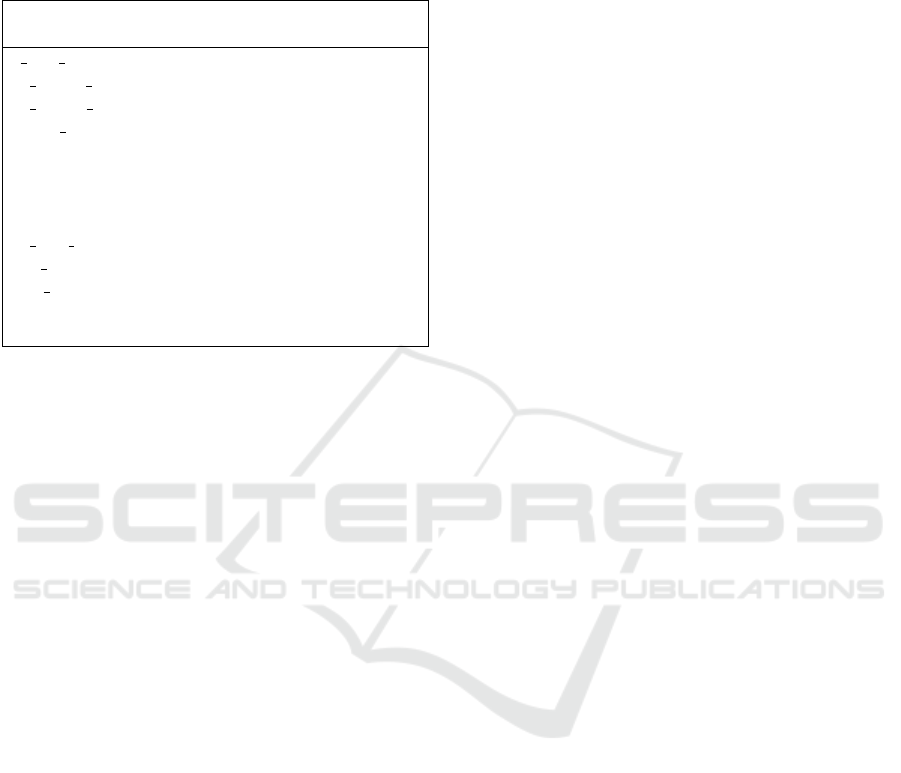
Table 5: Most relevant features using deep Taylor decompo-
sition, for each Spatial Relation, ranked by relevance (top 3
shown). # refers to the number of classified instances for that
Spatial Relation. L denotes language features, D denotes depth
features and F0-F30 denote geometric features.
Rank
Spatial Relation # 1 2 3
a cote de (beside) 242
F18
F3
F20
au dessus de (above) 2
F12
L F1
au niveau de (at the level of) 146
F18
F3
F20
autour de (around) 2 L F0
F23
contre (against) 26 F5
F23 F14
dans (in) 5 L
F30 F23
derriere (behind) 159 D L
F21
devant (in front) 150 D
F30
F5
en face de (opposite) 2 D L
F30
loin de (far from) 52 D
F30 F28
pres de (near) 499 L
F13 F12
sous (under) 69 L D
F24
sur (on) 68 L D
F30
6 CONCLUSIONS
We can conclude that
LRP
is useful for generating human-
interpretable explanations. This is partly due to the fact
that some of the geometric features lend themselves
to human-understandable terms, for example distance
between objects, whereas others, although they are not
terms used by human beings, are one step away from be-
ing so. For example, area overlap of bounding boxes can
act as a proxy to occlusion and to a lesser extent to depth.
The results show that language is important for
some prepositions but not for others, which concur with
observations from the cognitive linguistics literature
(Dobnik et al., 2018). On the other hand, it’s hard to
isolate a single feature as the most relevant, since feature
relevance was seen to be class-dependent. By employing
the centering approach a feature ordering in terms of
relevance was defined for each class. Applying the
relevance redistribution techniques described here to a
SRD
problem is a process which has not been carried out
before and allowed us to study the importance of features
per-class rather than globally. Additionally, we confirm
that depth features are important for some
SR
s and it
is therefore useful to have access to depth features in
addition to bounding boxes. In the future we plan to ex-
tend this study to more varied datasets and to analyze the
quality of explanations quantitatively, for example using
feature removal or inversion as in (Bach et al., 2015).
REFERENCES
Bach, S., Binder, A., Montavon, G., Klauschen, F., M
¨
uller,
K.-R., and Samek, W. (2015). On pixel-wise explanations
for non-linear classifier decisions by layer-wise relevance
propagation. PLoS One.
Belz, A., Muscat, A., Aberton, M., and Benjelloun, S. (2015).
Describing Spatial Relationships between Objects
in Images in English and French. pages 104–113.
Association for Computational Linguistics.
Belz, A., Muscat, A., Anguill, P., Sow, M., Vincent, G., and
Zinessabah, Y. (2018). SpatialVOC2K: A Multilingual
Dataset of Images with Annotations and Features for
Spatial Relations between Objects. Technical report.
Coventry, K. R., Prat-Sala, M., and Richards, L. (2001).
The Interplay between Geometry and Function in the
Comprehension of Over, Under, Above, and Below. J.
Mem. Lang.
Dai, B., Zhang, Y., and Lin, D. (2017). Detecting visual
relationships with deep relational networks. In Proc. -
30th IEEE Conf. Comput. Vis. Pattern Recognition, CVPR
2017, volume 2017-Janua, pages 3298–3308.
Dobnik, S., Ghanimifard, M., and Kelleher, J. (2018). Exploring
the Functional and Geometric Bias of Spatial Relations
Using Neural Language Models.
Dobnik, S. and Kelleher, J. (2015). Exploration of functional
semantics of prepositions from corpora of descriptions
of visual scenes.
Elliott, D. and Keller, F. (2013). Image Description using Visual
Dependency Representations. Technical report.
Gevrey, M., Dimopoulos, I., and Lek, S. (2003). Review and
comparison of methods to study the contribution of vari-
ables in artificial neural network models. In Ecol. Modell.
Hashem, S. (1992). Sensitivity analysis for feedforward
artificial neural networks with differentiable activation
functions. In Proc. 1992 Int. Jt. Conf. Neural Networks.
Lu, C., Krishna, R., Bernstein, M., and Fei-Fei, L. (2016).
Visual relationship detection with language priors. In
Lect. Notes Comput. Sci. (including Subser. Lect. Notes
Artif. Intell. Lect. Notes Bioinformatics), volume 9905
LNCS, pages 852–869.
Montavon, G., Lapuschkin, S., Binder, A., Samek, W., and
M
¨
uller, K.-R. (2017). Explaining nonlinear classification
decisions with deep Taylor decomposition. Pattern
Recognit.
Muscat, A. and Belz, A. (2017). Learning to Generate Descrip-
tions of Visual Data Anchored in Spatial Relations. IEEE
Comput. Intell. Mag., 12(3).
Ramisa, A., Wang, J., Lu, Y., Dellandrea, E., Moreno-Noguer,
F., and Gaizauskas, R. (2015). Combining geometric,
textual and visual features for predicting prepositions in
image descriptions. In Conf. Proc. - EMNLP 2015 Conf.
Empir. Methods Nat. Lang. Process., pages 214–220.
Association for Computational Linguistics.
Ribeiro, M. T., Singh, S., and Guestrin, C. (2016). Model-
Agnostic Interpretability of Machine Learning.
Zhu, Y. and Jiang, S. (2018). Deep structured learning for
visual relationship detection. In 32nd AAAI Conf. Artif.
Intell. AAAI 2018, pages 7623–7630.
Explaining Spatial Relation Detection using Layerwise Relevance Propagation
385