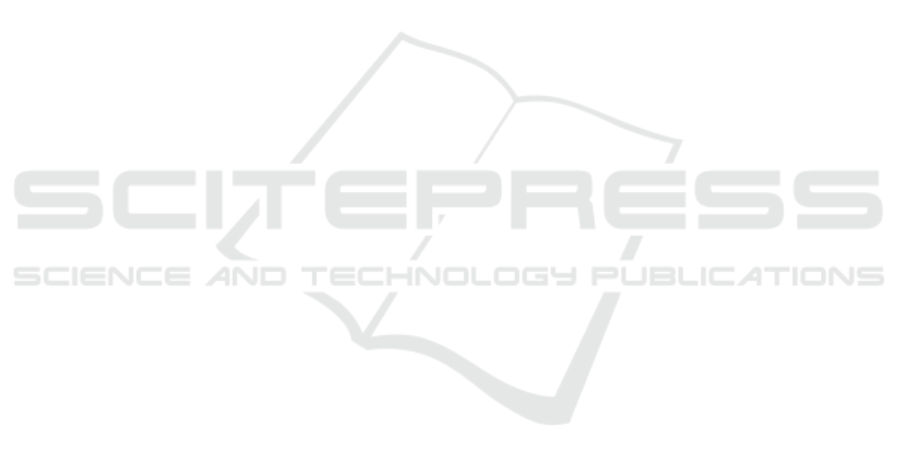
ACKNOWLEDGEMENTS
We would like to thank all participants that contribute
to the study. We would also like to thank the medical
staff employed at PZU d-r Andon Kochev, Javor bb,
Radovish, North Macedonia.
This work was supported by the Slovenian Re-
search Agency Program P2-0098 and has received
funding from the European Union’s Horizon 2020 re-
search and innovation programme under grant agree-
ment No 863059 and No 769661.
Information and the views set out in this publica-
tion are those of the authors and do not necessarily re-
flect the official opinion of the European Union. Nei-
ther the European Union institutions and bodies nor
any person acting on their behalf may be held respon-
sible for the use that may be made of the information
contained herein.
REFERENCES
Ahmad, S., Chen, S., Soueidan, K., Batkin, I., Bolic, M.,
Dajani, H., and Groza, V. (2012). Electrocardiogram-
assisted blood pressure estimation. IEEE Transactions
on Biomedical Engineering, 59(3):608–618.
Association, A. H. et al. (2014). Understanding blood pres-
sure readings. Updated November, 17.
Chan, K., Hung, K., and Zhang, Y. (2001). Noninva-
sive and cuffless measurements of blood pressure for
telemedicine. In Engineering in Medicine and Biol-
ogy Society, 2001. Proceedings of the 23rd Annual In-
ternational Conference of the IEEE, volume 4, pages
3592–3593. IEEE.
Chen, T., He, T., Benesty, M., Khotilovich, V., and Tang,
Y. (2015). Xgboost: extreme gradient boosting. R
package version 0.4-2, pages 1–4.
Chen, Z., Yang, X., Teo, J. T., and Ng, S. H. (2013).
Noninvasive monitoring of blood pressure using op-
tical ballistocardiography and photoplethysmograph
approaches. In Engineering in Medicine and Biol-
ogy Society (EMBC), 2013 35th Annual International
Conference of the IEEE, pages 2425–2428. IEEE.
Christ, M., Braun, N., Neuffer, J., and Kempa-Liehr, A. W.
(2018). Time series feature extraction on basis of scal-
able hypothesis tests (tsfresh–a python package). Neu-
rocomputing, 307:72–77.
Daimiwal, N., Sundhararajan, M., and Shriram, R. (2014).
Respiratory rate, heart rate and continuous measure-
ment of bp using ppg. In Communications and Signal
Processing (ICCSP), 2014 International Conference
on, pages 999–1002. IEEE.
Ding, X.-R., Zhang, Y.-T., Liu, J., Dai, W.-X., and Tsang,
H. K. (2016). Continuous cuffless blood pressure es-
timation using pulse transit time and photoplethysmo-
gram intensity ratio. IEEE Transactions on Biomedi-
cal Engineering, 63(5):964–972.
Fan, X., Ye, Q., Yang, X., and Choudhury, S. D. (2018).
Robust blood pressure estimation using an rgb cam-
era. Journal of Ambient Intelligence and Humanized
Computing, pages 1–8.
Friedman, J. H. (2002). Stochastic gradient boosting. Com-
putational statistics & data analysis, 38(4):367–378.
Gao, S. C., Wittek, P., Zhao, L., and Jiang, W. J. (2016).
Data-driven estimation of blood pressure using photo-
plethysmographic signals. In Engineering in Medicine
and Biology Society (EMBC), 2016 IEEE 38th An-
nual International Conference of the, pages 766–769.
IEEE.
Hailstone, J. and Kilding, A. E. (2011). Reliability and va-
lidity of the zephyr
TM
bioharness
TM
to measure respi-
ratory responses to exercise. Measurement in Physical
Education and Exercise Science, 15(4):293–300.
Hassan, M. K. B. A., Mashor, M., Nasir, N. M., and Mo-
hamed, S. (2008). Measuring of systolic blood pres-
sure based on heart rate. In 4th Kuala Lumpur Interna-
tional Conference on Biomedical Engineering 2008,
pages 595–598. Springer.
Hernando, D., Roca, S., Sancho, J., Alesanco,
´
A., and
Bail
´
on, R. (2018). Validation of the apple watch for
heart rate variability measurements during relax and
mental stress in healthy subjects. Sensors, 18(8):2619.
Ji, X.-j., Li, S.-z., and Li, T. (2001). Application of the
correlation analysis in feature selection. Journal of
Test and Measurement Technology, 15(1):15–18.
Kachuee, M., Kiani, M. M., Mohammadzade, H., and Sha-
bany, M. (2015). Cuff-less high-accuracy calibration-
free blood pressure estimation using pulse transit time.
In Circuits and Systems (ISCAS), 2015 IEEE Interna-
tional Symposium on, pages 1006–1009. IEEE.
Katayama, K., Ishizawa, H., Koyama, S., and Fujimoto, K.
(2018). Improvement of blood pressure prediction us-
ing artificial neural network. In 2018 IEEE Interna-
tional Symposium on Medical Measurements and Ap-
plications (MeMeA), pages 1–5. IEEE.
Kim, N., Krasner, A., Kosinski, C., Wininger, M., Qadri,
M., Kappus, Z., Danish, S., and Craelius, W. (2016).
Trending autoregulatory indices during treatment for
traumatic brain injury. Journal of clinical monitoring
and computing, 30(6):821–831.
Kocev, D., Vens, C., Struyf, J., and D
ˇ
zeroski, S. (2013).
Tree ensembles for predicting structured outputs. Pat-
tern Recognition, 46(3):817–833.
Liaw, A., Wiener, M., et al. (2002). Classification and re-
gression by randomforest. R news, 2(3):18–22.
Mousavi, S. S., Charmi, M., Firouzmand, M., Hemmati, M.,
and Moghadam, M. (2019a). A new approach based
on dynamical model of the ecg signal to blood pres-
sure estimation. In 2019 4th International Conference
on Pattern Recognition and Image Analysis (IPRIA),
pages 210–215. IEEE.
Mousavi, S. S., Firouzmand, M., Charmi, M., Hemmati,
M., Moghadam, M., and Ghorbani, Y. (2019b). Blood
pressure estimation from appropriate and inappropri-
ate ppg signals using a whole-based method. Biomed-
ical Signal Processing and Control, 47:196–206.
BIOSIGNALS 2020 - 13th International Conference on Bio-inspired Systems and Signal Processing
234