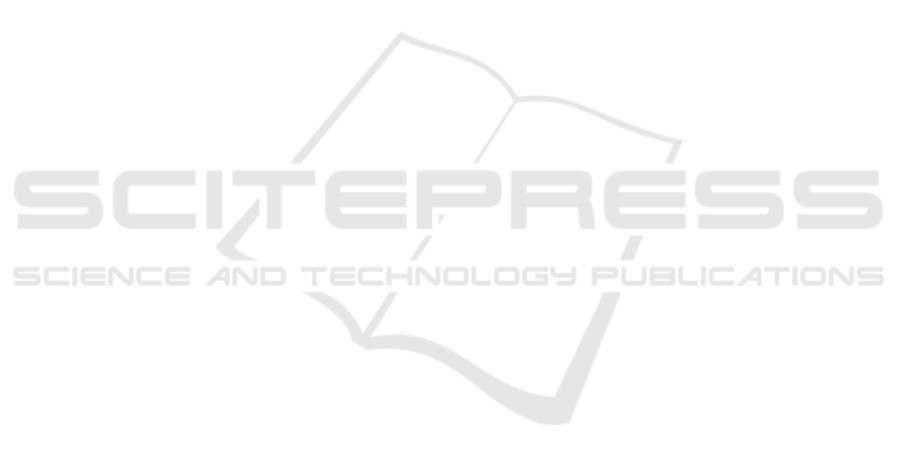
One example is the application of this framework
to active learning mechanisms as most of the available
algorithms are specific to specific conditions, while
not detecting others. The ability to detect similar ab-
normalities in the ECG signal, the classifier could ac-
tively create new labels for patterns that diverge from
the normal ECG signal, while increasing new diag-
nostic possibilities. That said, this technique could
not only aid in the diagnostic of arrhythmias, but also
contribute to the study of these pathological anoma-
lies, by finding correlations between the different ex-
pressions of the ECG morphology, and also by ex-
ploring these manifestations with different variables
in mind such as the patient’s gender, age, medications,
among other factors. Another example is by analysing
billions of data points in wearable data, the medical
doctor could focus only in the parts which contained
different aspects of the signal, so that he could diag-
nose without wasting hours or days of analysis.
This method helps to increase the possibilities, not
only for detection, but also for studying what exactly
is a normal cycle and which are the deviation patterns.
By having early detection procedures, one could early
seek for medical help without developing a symp-
tomatic episode. This preventive point of view could
represent a major change in perspective in how medi-
cal care should be delivered in the future.
REFERENCES
Arrhythmia — National Heart, Lung, and Blood Institute
(NHLBI).
Acharya, R., Krishnan, S. M., Spaan, J. A., and Suri,
J. S. (2007). Advances in cardiac signal processing.
Springer.
Acharya, U. R., Fujita, H., Lih, O. S., Hagiwara, Y., Tan,
J. H., and Adam, M. (2017). Automated detection
of arrhythmias using different intervals of tachycar-
dia ECG segments with convolutional neural network.
Information Sciences, 405:81–90.
Ansari, S., Gryak, J., and Najarian, K. (2018). Noise de-
tection in electrocardiography signal for robust heart
rate variability analysis: A deep learning approach.
In 2018 40th Annual International Conference of the
IEEE Engineering in Medicine and Biology Society
(EMBC), pages 5632–5635. IEEE.
Brown, B. H. (cop. 1999). Medical physics and biomedi-
cal engineering. Medical Science Series. Institute of
Physics Publishing, Bristol.
Coiera, E. (2003). Guide to Health Informatics. Oxford
University Press, second edition.
Faust, O., Hagiwara, Y., Hong, T. J., Lih, O. S., and
Acharya, U. R. (2018). Deep learning for healthcare
applications based on physiological signals: A review.
Computer Methods and Programs in Biomedicine,
161:1 – 13.
Ghimes, A.-M., Avram, A.-M., and Vladuta, V.-A. (2018).
A character prediction approach in a security context
using a recurrent neural network. In 2018 Interna-
tional Symposium on Electronics and Telecommuni-
cations (ISETC), pages 1–4. IEEE.
Goldberger, A. L., Amaral, L. A., Glass, L., Hausdorff,
J. M., Ivanov, P. C., Mark, R. G., Mietus, J. E., Moody,
G. B., Peng, C.-K., and Stanley, H. E. (2000). Phys-
iobank, physiotoolkit, and physionet: components of
a new research resource for complex physiologic sig-
nals. Circulation, 101(23):e215–e220.
Hannun, A. Y., Rajpurkar, P., Haghpanahi, M., Tison, G. H.,
Bourn, C., Turakhia, M. P., and Ng, A. Y. (2019).
Cardiologist-level arrhythmia detection and classifi-
cation in ambulatory electrocardiograms using a deep
neural network. Nature Medicine, 25(1):65–69.
Iyengar, N., Peng, C., Morin, R., Goldberger, A. L., and
Lipsitz, L. A. (1996). Age-related alterations in the
fractal scaling of cardiac interbeat interval dynam-
ics. American Journal of Physiology-Regulatory, Inte-
grative and Comparative Physiology, 271(4):R1078–
R1084.
John, J. N., Galloway, C., and Valys, A. (2018). Deep con-
volutional neural networks for noise detection in ecgs.
arXiv preprint arXiv:1810.04122.
Johnson, K. W., Soto, J. T., Glicksberg, B. S., Shameer,
K., Miotto, R., Ali, M., Ashley, E., and Dudley, J. T.
(2018). Artificial intelligence in cardiology. Journal
of the American College of Cardiology, 71(23):2668 –
2679.
Mao, Z., Yao, W. X., and Huang, Y. (2017). Eeg-based
biometric identification with deep learning. In 2017
8th International IEEE/EMBS Conference on Neural
Engineering (NER), pages 609–612.
Moody, G. B. and Mark, R. G. (2001). The impact of the
mit-bih arrhythmia database. IEEE Engineering in
Medicine and Biology Magazine, 20(3):45–50.
Moody, G. B., Muldrow, W., and Mark, R. G. (1984). A
noise stress test for arrhythmia detectors. Computers
in cardiology, 11(3):381–384.
Pascanu, R., Gulcehre, C., Cho, K., and Bengio, Y. (2013).
How to construct deep recurrent neural networks.
Rodrigues, J., Belo, D., and Gamboa, H. (2017). Noise de-
tection on ecg based on agglomerative clustering of
morphological features. Computers in Biology and
Medicine, 87:322 – 334.
S. Andersen, R., Peimankar, R., and Puthusserypady, S.
(2018). A deep learning approach for real-time de-
tection of atrial fibrillation. Expert Systems with Ap-
plications, 115.
Satija, U., Ramkumar, B., and Manikandan, M. S.
(2018). Automated ecg noise detection and classifi-
cation system for unsupervised healthcare monitoring.
IEEE Journal of Biomedical and Health Informatics,
22(3):722–732.
Silipo, R. and Marchesi, C. (1998). Artificial neural net-
works for automatic ecg analysis. IEEE Transactions
on Signal Processing, 46(5):1417–1425.
Xiong, F., Chen, D., Chen, Z., and Dai, S. (2019). Cancel-
lation of motion artifacts in ambulatory ECG signals
using TD-LMS adaptive filtering techniques. Journal
of Visual Communication and Image Representation,
58:606–618.
Detection of Abnormalities in Electrocardiogram (ECG) using Deep Learning
243