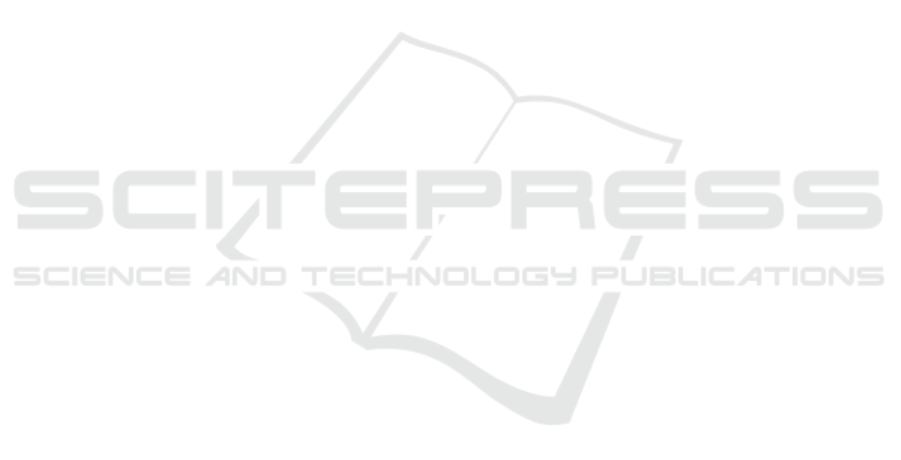
improvements by more advanced segmentation ap-
proaches. From our experience, we thus conclude
that the latter two variants of watershed segmentation
might both serve well as an evaluation baseline for the
automatic segmentation of cell nuclei in fluorescence
microscopy images by more involved methods.
6 CONCLUSIONS
In this paper we addressed the benchmarking of cell
nuclei segmentation algorithms with a particular fo-
cus on fluorescence microscopy images. Specifi-
cally, we first described the considered dataset and ex-
plained how this data needs to be processed to serve
our purposes, where we in particular pointed to sev-
eral snares that might distort results if not properly
taken care of. Afterwards, we recalled the water-
shed transformation and gave a detailed account about
our implementation of a segmentation pipeline built
around this well-known image decomposition tool.
Next, we provided a review of three classes of well-
established evaluation measures for image segmenta-
tion. Finally, we briefly compared the performance
of several variants of our watershed segmentation
pipeline, where we not only relied on the previously
discussed quantitative evaluation measures but rather
combined it with a visual inspection of the cell bound-
ary images to collate the obtained results with our ex-
pectations. Everything combined, we thus explained
the set-up of a watershed segmentation pipeline that
might serve well as a baseline for the assessment of
more sophisticated cell nuclei detection methods and
as such constitutes an important component for our
future efforts to contribute to a more personalised im-
mune checkpoint inhibitor-based cancer therapy.
ACKNOWLEDGEMENTS
This work has been supported by the European Union
and the federal state of North-Rhine-Westphalia
(EFRE-0801303).
The authors would like to thank L. P. Coelho for
sharing useful additional information concerning the
publicly available data and algorithms.
REFERENCES
Antonia, S. J., Villegas, A., et al. (2017). Durvalumab af-
ter Chemoradiotherapy in Stage III Non–Small-Cell
Lung Cancer. New England Journal of Medicine,
377(20):1919–1929.
Baltissen, D., Wollmann, T., et al. (2018). Comparison of
segmentation methods for tissue microscopy images
of glioblastoma cells. In Proceedings of the 2018
IEEE 15th International Symposium on Biomedical
Imaging (ISBI 2018).
Bamford, P. (2003). Empirical comparison of cell segmen-
tation algorithms using an annotated dataset. In Pro-
ceedings of the 2003 International Conference on Im-
age Processing (Cat. No.03CH37429). IEEE.
Chalana, V. and Kim, Y. (1997). A Methodology for Eval-
uation of Boundary Detection Algorithms on Medi-
cal Images. IEEE Transactions on Medical Imaging,
16(5):642–652.
Coelho, L. P., Shariff, A., and Murphy, R. F. (2009).
Nuclear segmentation in microscope cell images:
A hand-segmented dataset and comparison of algo-
rithms. In Proceedings of the 2009 IEEE International
Symposium on Biomedical Imaging: From Nano to
Macro. IEEE.
Digabel, H. and Lantu
´
ejoul, C. (1978). Iterative algorithms.
In Proceedings of the 2nd European Symp. Quantita-
tive Analysis of Microstructures in Material Science,
Biology and Medicine, volume 19, page 8. Stuttgart,
West Germany: Riederer Verlag.
Jaccard, P. (1901).
´
Etude comparative de la distribution flo-
rale dans une portion des Alpes et des Jura. Bull Soc
Vaudoise Sci Nat, 37:547–579.
Kelly, P. N. (2018). The Cancer Immunotherapy Revolu-
tion. Science, 359(6382):1344–1345.
Osuna, E. G., Hua, J., et al. (2007). Large-Scale Au-
tomated Analysis of Location Patterns in Randomly
Tagged 3T3 Cells. Annals of Biomedical Engineer-
ing, 35(6):1081–1087.
Peng, T., Bonamy, G. M. C., et al. (2010). Determining
the distribution of probes between different subcellu-
lar locations through automated unmixing of subcel-
lular patterns. Proceedings of the National Academy
of Sciences, 107(7):2944–2949.
Rand, W. M. (1971). Objective criteria for the evaluation of
clustering methods. Journal of the American Statisti-
cal Association, 66(336):846–850.
Russ, J. C. and Neal, F. B. (2015). The Image Processing
Handbook. Taylor & Francis Inc.
Schadendorf, D., Hodi, F. S., et al. (2015). Pooled Anal-
ysis of Long-Term Survival Data From Phase II and
Phase III Trials of Ipilimumab in Unresectable or
Metastatic Melanoma. Journal of Clinical Oncology,
33(17):1889–1894.
Sonka, M., Hlavac, V., and Boyle, R. (2014). Image
Processing, Analysis, and Machine Vision. Cengage
Learning.
Todaro, G. J. (1963). Quantitative studies of the growth of
mouse embryo cells in culture and their development
into established lines. The Journal of Cell Biology,
17(2):299–313.
Vincent, L. and Soille, P. (1991). Watersheds in digital
spaces: an efficient algorithm based on immersion
simulations. IEEE Transactions on Pattern Analysis
and Machine Intelligence, 13(6):583–598.
On Benchmarking Cell Nuclei Segmentation Algorithms for Fluorescence Microscopy
171