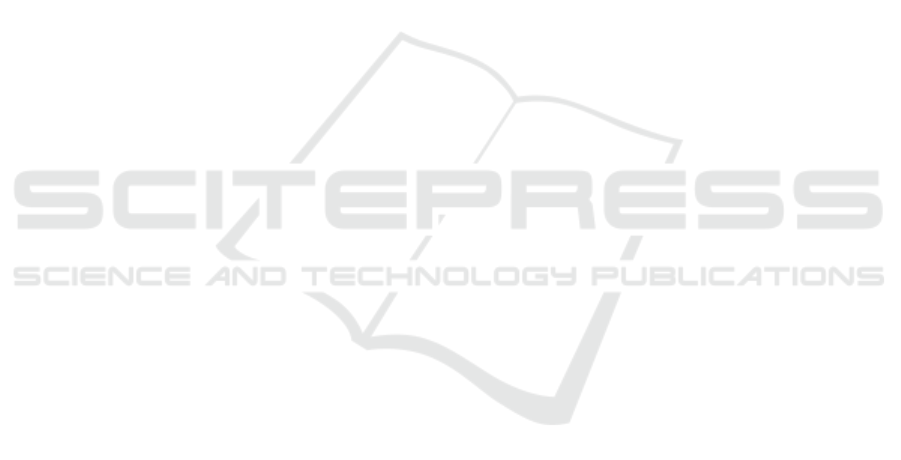
reconstruction, however, it is worth noticing that the
solutions by sLORETA are smooth (Pascual-Marqui,
2002), while the other hand, MSP present more sparse
solutions (L
´
opez et al., 2014; Friston et al., 2008).
Therefore in future works, we will consider the use
of error measurements that involve the temporal evo-
lution of the reconstructed sources and the sparseness
of the solutions.
The pipeline presented considers a basic filter
stage using band-pass filters with the intention to fo-
cus on the partial brain models to source activity
reconstruction. However, several studies (Mu
˜
noz-
Guti
´
errez et al., 2018; Hansen et al., 2019) have
shown that the using of advanced techniques for fre-
quency decomposition like empirical mode decompo-
sition EMD, multivariate EMD, noise assisted EMD,
and wavelets can offer a solution for unmixing the
source activity improving the brain mapping algo-
rithms. Those techniques will be studied on partial
brain models in future publications.
AUTHOR CONTRIBUTIONS
This part was intentionally removed for reviewing
purposes All the authors conceived and designed the
experiments. AFS performed the experiments. All
the authors analyzed the data, wrote and refined the
article.
ACKNOWLEDGMENT
This part was intentionally removed for reviewing
purposes This work was supported by the Norwegian
University of Science and Technology NTNU, project
”David and Goliath: single-channel EEG unravels its
power through adaptive signal analysis”.
REFERENCES
Biscay, R. J., Bosch-Bayard, J. F., and Pascual-Marqui,
R. D. (2018). Unmixing EEG Inverse solutions based
on brain segmentation. Frontiers in Neuroscience,
12(MAY).
Burianov
´
a, H., Marstaller, L., Sowman, P., Tesan, G., Rich,
A. N., Williams, M., Savage, G., and Johnson, B. W.
(2013). Multimodal functional imaging of motor im-
agery using a novel paradigm. NeuroImage.
Fonov, V., Evans, A., McKinstry, R., Almli, C., and
Collins, D. (2009). Unbiased nonlinear average age-
appropriate brain templates from birth to adulthood.
NeuroImage, 47:S102.
Friston, K., Harrison, L., Daunizeau, J., Kiebel, S., Phillips,
C., Trujillo-Barreto, N., Henson, R., Flandin, G., and
Mattout, J. (2008). Multiple sparse priors for the
M/EEG inverse problem. NeuroImage, 39(3):1104–
1120.
Giraldo, E., Peluffo-Ordo
˜
nez, D., and Castellanos-
Dominguez, G. (2012). Weighted Time Series Analy-
sis for Electroencephalographic Source Localization.
DYNA Universidad Nacional de Colombia, 79:64–70.
H
¨
am
¨
al
¨
ainen, M. S. and Ilmoniemi, R. J. (1994). Interpret-
ing magnetic fields of the brain: minimum norm esti-
mates. Medical & Biological Engineering & Comput-
ing, 32(1):35–42.
Hansen, S. T., Hemakom, A., Gylling Safeldt, M., Krohne,
L. K., Madsen, K. H., Siebner, H. R., Mandic, D. P.,
and Hansen, L. K. (2019). Unmixing oscillatory
brain activity by EEG source localization and empiri-
cal mode decomposition. Computational Intelligence
and Neuroscience, 2019.
Huang, Y., Parra, L. C., and Haufe, S. (2016). The new
york head—a precise standardized volume conduc-
tor model for eeg source localization and tes target-
ing. NeuroImage, 140:150 – 162. Transcranial electric
stimulation (tES) and Neuroimaging.
Jatoi, M. A. and Kamel, N. (2018). Brain source localiza-
tion using reduced eeg sensors. Signal, Image and
Video Processing, 12(8):1447–1454.
Jatoi, M. A., Kamel, N., Malik, A. S., Faye, I., and Be-
gum, T. (2014). A survey of methods used for source
localization using eeg signals. Biomedical Signal Pro-
cessing and Control, 11:42 – 52.
L
´
opez, J. D., Litvak, V., Espinosa, J. J., Friston, K., and
Barnes, G. R. (2014). Algorithmic procedures for
Bayesian MEG/EEG source reconstruction in SPM.
NeuroImage, 84:476–487.
Mu
˜
noz-Guti
´
errez, P. A., Giraldo, E., Bueno-L
´
opez, M.,
and Molinas, M. (2018). Localization of active brain
sources from EEG signals using empirical mode de-
composition: a comparative study. Frontiers in Inte-
grative Neuroscience, 12.
O’Leary, J. (1970). Hans berger on the electroencephalo-
gram of man. the fourteen original reports on the hu-
man electroencephalogram. translated from the ger-
man and edited by pierre gloor. Science, 168:562–563.
Pascual-Marqui, R. D. (2002). Standardized low-resolution
brain electromagnetic tomography (sLORETA): Tech-
nical details. Methods and Findings in Experimental
and Clinical Pharmacology, 24(SUPPL. D):5–12.
Pascual-Marqui, R. D., Michel, C., and Lehmann, D.
(1994). Low resolution electromagnetic tomography:
a new method for localizing electrical activity in the
brain. International Journal of Psychophysiology,
18(1):49–65.
Qiu, Z., Allison, B. Z., Jin, J., Zhang, Y., Wang, X., Li, W.,
and Cichocki, A. (2017). Optimized motor imagery
paradigm based on imagining Chinese characters writ-
ing movement. IEEE Transactions on Neural Systems
and Rehabilitation Engineering, 25(7):1009–1017.
Soler, A., Giraldo, E., and Molinas, M. (2019). Partial Brain
Model For Real-Time Classification Of RGB Visual
BIOIMAGING 2020 - 7th International Conference on Bioimaging
62