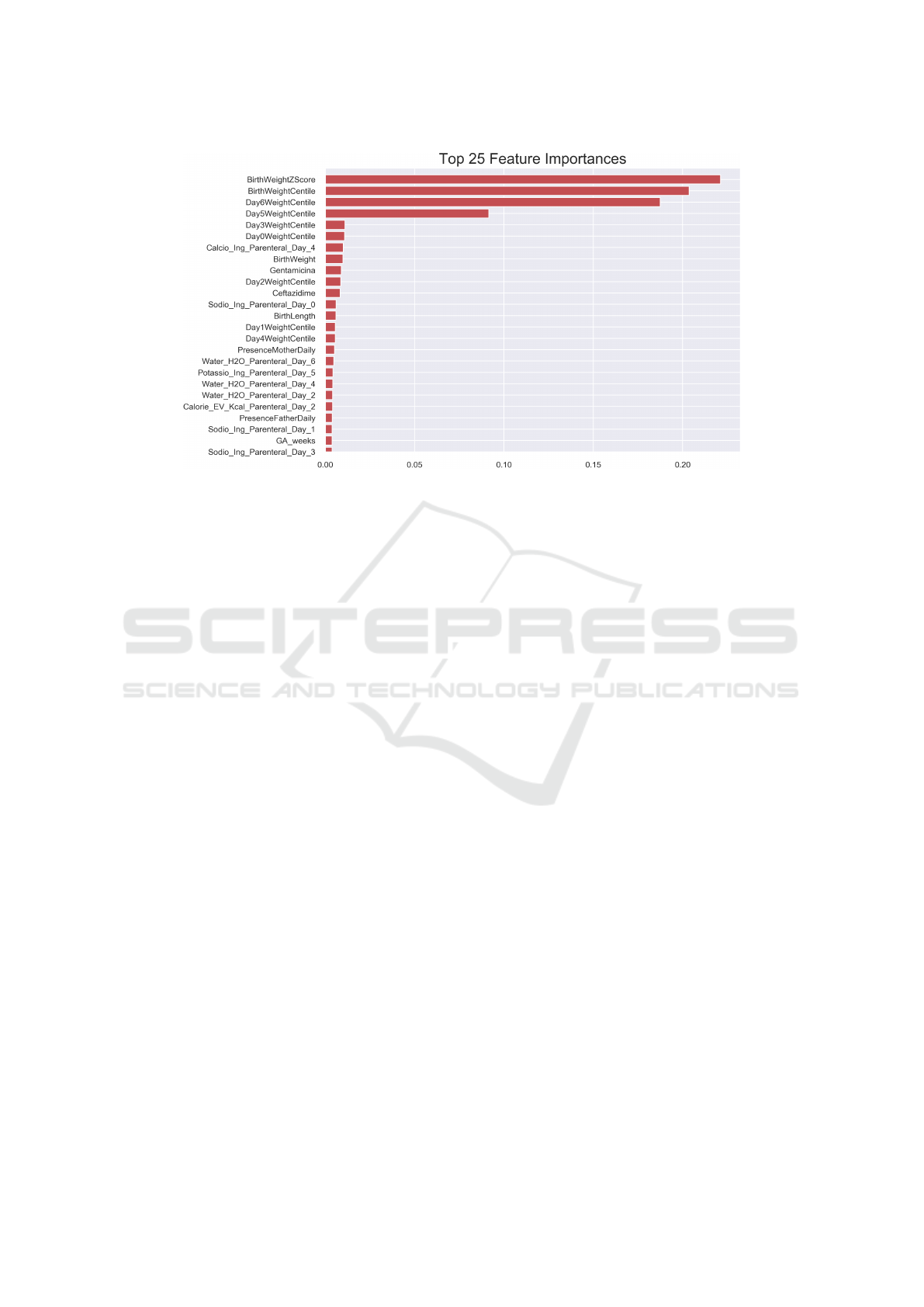
Figure 4: Feature importance. The x axis marks the average decrease in variance over all the estimators (trees).
lacks a pragmatic validation in the field of work (Cab-
itza and Zeitoun, 2019).
REFERENCES
Cabitza, F. and Zeitoun, J.-D. (2019). The proof of the pud-
ding: in praise of a culture of real-world validation for
medical artificial intelligence. Annals of translational
medicine, 7(8).
Clark, R. H., Thomas, P., and Peabody, J. (2003). Extrauter-
ine growth restriction remains a serious problem in
prematurely born neonates. Pediatrics, 111(5):986–
990.
Ehrenkranz, R. A., Dusick, A. M., Vohr, B. R., Wright,
L. L., Wrage, L. A., Poole, W. K., et al. (2006).
Growth in the neonatal intensive care unit influ-
ences neurodevelopmental and growth outcomes of
extremely low birth weight infants. Pediatrics,
117(4):1253–1261.
Fenton, T. R. (2003). A new growth chart for preterm ba-
bies: Babson and benda’s chart updated with recent
data and a new format. BMC pediatrics, 3(1):13.
Hayakawa, M., Okumura, A., Hayakawa, F., Kato, Y.,
Ohshiro, M., Tauchi, N., and Watanabe, K. (2003).
Nutritional state and growth and functional maturation
of the brain in extremely low birth weight infants. Pe-
diatrics, 111(5):991–995.
Lee, S. M., Kim, N., Namgung, R., Park, M., Park, K., and
Jeon, J. (2018). Prediction of postnatal growth fail-
ure among very low birth weight infants. Scientific
reports, 8(1):3729.
Lin, Z., Green, R. S., Chen, S., Wu, H., Liu, T., Li, J., Wei,
J., and Lin, J. (2015). Quantification of eugr as a mea-
sure of the quality of nutritional care of premature in-
fants. PloS one, 10(7):e0132584.
Lucas, A., Morley, R., and Cole, T. J. (1998). Randomised
trial of early diet in preterm babies and later intelli-
gence quotient. Bmj, 317(7171):1481–1487.
Miller, M., Vaidya, R., Rastogi, D., Bhutada, A., and Ras-
togi, S. (2014). From parenteral to enteral nutri-
tion: a nutrition-based approach for evaluating post-
natal growth failure in preterm infants. Journal of Par-
enteral and Enteral Nutrition, 38(4):489–497.
Radmacher, P. G., Looney, S. W., Rafail, S. T., and
Adamkin, D. H. (2003). Prediction of extrauterine
growth retardation (eugr) in vvlbw infants. Journal
of Perinatology, 23(5):392.
Ribeiro, M. T., Singh, S., and Guestrin, C. (2016). Why
should i trust you?: Explaining the predictions of any
classifier. In Proceedings of the 22nd ACM SIGKDD
international conference on knowledge discovery and
data mining, pages 1135–1144. ACM.
Stoll, B. J., Hansen, N. I., Bell, E. F., Shankaran, S., Lap-
took, A. R., Walsh, M. C., Hale, E. C., Newman, N. S.,
Schibler, K., Carlo, W. A., et al. (2010). Neonatal
outcomes of extremely preterm infants from the nichd
neonatal research network. Pediatrics, 126(3):443–
456.
The International Fetal and Newborn Growth Consor-
tium for the 21st Century (2019). Intergrowth-21
standards and tools. https://intergrowth21.tghn.org/
standards-tools/. Accessed: 2019-10-10.
Villar, J., Ismail, L. C., Victora, C. G., Ohuma, E. O.,
Bertino, E., Altman, D. G., Lambert, A., Papa-
georghiou, A. T., Carvalho, M., Jaffer, Y. A., et al.
(2014). International standards for newborn weight,
length, and head circumference by gestational age
and sex: the newborn cross-sectional study of the
intergrowth-21st project. The Lancet, 384(9946):857–
868.
Developing a Machine Learning Model for Predicting Postnatal Growth in Very Low Birth Weight Infants
497