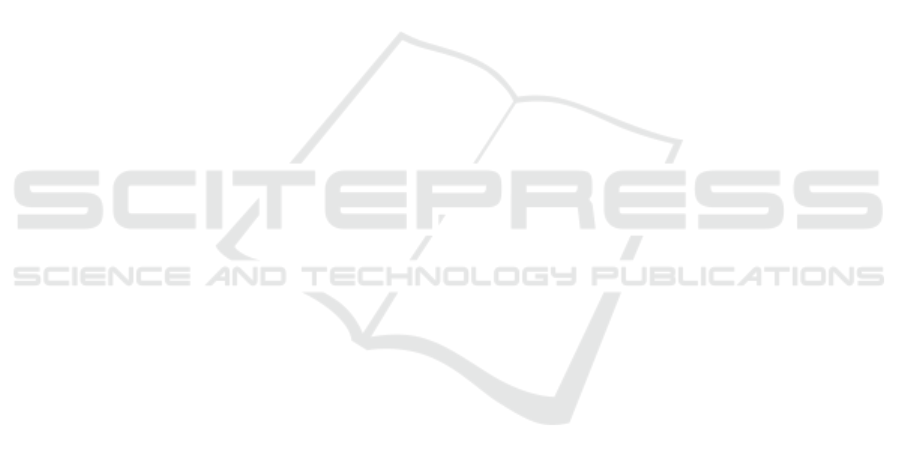
REFERENCES
Adkinson, J. A., Karumuri, B., Hutson, T. N., Liu,
R., Alamoudi, O., Vlachos, I., and Iasemidis, L.
(2019). Connectivity and centrality characteristics of
the epileptogenic focus using directed network analy-
sis. IEEE Transactions on Neural Systems and Reha-
bilitation Engineering, 27(1):22–30.
Akbarian, B. and Erfanian, A. (2017). Automatic detec-
tion of ptz-induced seizures based on functional brain
connectivity network in rats. In 2017 8th Interna-
tional IEEE/EMBS Conference on Neural Engineer-
ing (NER), pages 576–579. IEEE.
Anastasiadou, M. N., Christodoulakis, M., Papathanasiou,
E. S., Papacostas, S. S., Hadjipapas, A., and Mit-
sis, G. D. (2019). Graph theoretical characteristics of
eeg-based functional brain networks in patients with
epilepsy: The effect of reference choice and volume
conduction. Frontiers in Neuroscience, 13:221.
Andrea Avena-Koenigsberger, Bratislav Msic, O. S. (2017).
Communication dynamics in complex brain networks.
Nature Reviews Neuroscience, 19:17 – 33.
Bartolomei, F., Lagarde, S., Wendling, F., McGonigal, A.,
Jirsa, V., Guye, M., and B
´
enar, C. (2017). Defining
epileptogenic networks: Contribution of seeg and sig-
nal analysis. Epilepsia, 58(7):1131–1147.
Beghi, E. (2019). Social functions and socioeconomic vul-
nerability in epilepsy. Epilepsy & Behavior.
Beghi, E., Giussani, G., Abd-Allah, F., Abdela, J., Abde-
lalim, A., Abraha, H. N., Adib, M. G., Agrawal, S.,
Alahdab, F., Awasthi, A., et al. (2019). Global, re-
gional, and national burden of epilepsy, 1990–2016:
a systematic analysis for the global burden of disease
study 2016. The Lancet Neurology, 18(4):357–375.
Berglind, F., Andersson, M., and Kokaia, M. (2018). Dy-
namic interaction of local and transhemispheric net-
works is necessary for progressive intensification of
hippocampal seizures. Scientific reports, 8(1):5669.
Biasiucci, A., Franceschiello, B., and Murray, M. M.
(2019). Electroencephalography. Current Biology,
29(3):R80–R85.
Chapeton, J. I., Inati, S. K., and Zaghloul, K. A. (2017).
Stable functional networks exhibit consistent timing
in the human brain. Brain, 140(3):628–640.
Cota, V., Marcela Bacellar Drabowski, B., Carla de
Oliveira, J., and Moraes, M. (2016). The epilep-
tic amygdala: Toward the development of a neural
prosthesis by temporally coded electrical stimulation.
Journal of Neuroscience Research, 94:463–485.
Cover, T. M. and Thomas, J. A. (2012). Elements of infor-
mation theory. John Wiley & Sons.
Devinsky, O., Vezzani, A., O´Brien, T. J., Scheffer, I. E.,
Curtis, M., and Perucca, P. (2018). Epilepsy. Nature
Reviews Disease Primers, 4.
Endo, W., Santos, F. P., Simpson, D., Maciel, C. D., and
Newland, P. L. (2015). Delayed mutual information
infers patterns of synaptic connectivity in a proprio-
ceptive neural network. Journal of computational neu-
roscience, 38(2):427–438.
Faes, L., Porta, A., and Nollo, G. (2010). Testing
frequency-domain causality in multivariate time se-
ries. IEEE Transactions on Biomedical Engineering,
57(8):1897–1906.
Gribkova, E. D., Ibrahim, B. A., and Llano, D. A. (2018). A
novel mutual information estimator to measure spike
train correlations in a model thalamocortical network.
Journal of neurophysiology, 120(6):2730–2744.
Ibrahim, F., Abd-Elateif El-Gindy, S., El-Dolil, S. M., El-
Fishawy, A. S., El-Rabaie, E.-S. M., Dessouky, M. I.,
Eldokany, I. M., Alotaiby, T. N., Alshebeili, S. A.,
and Abd El-Samie, F. E. (2019). A statistical frame-
work for eeg channel selection and seizure prediction
on mobile. International Journal of Speech Technol-
ogy, 22(1):191–203.
Kim, H.-R., Go, H.-J., and Kim, S.-Y. (2018). Discrimina-
tion of mild alzheimer’s disease patients using cluster
analysis of information transmission in eeg. Journal
of the Korean Physical Society, 73(3):377–387.
Lancaster, G., Iatsenko, D., Pidde, A., Ticcinelli, V., and
Stefanovska, A. (2018). Surrogate data for hypothesis
testing of physical systems. Physics Reports, 748:1 –
60. Surrogate data for hypothesis testing of physical
systems.
Li, S., Xiao, Y., Zhou, D., and Cai, D. (2018). Causal infer-
ence in nonlinear systems: Granger causality versus
time-delayed mutual information. Physical Review E,
97(5):052216.
Li, S., Xu, J., Chen, G., Lin, L., Zhou, D., and Cai, D.
(2017). The characterization of hippocampal theta-
driving neurons—a time-delayed mutual information
approach. Scientific reports, 7(1):5637.
Mei, T., Wei, X., Chen, Z., Tian, X., Dong, N., Li, D.,
and Zhou, Y. (2019). Epileptic foci localization based
on mapping the synchronization of dynamic brain net-
work. BMC medical informatics and decision making,
19(1):19.
Nichols, J., Seaver, M., Trickey, S., Todd, M., Olson, C.,
and Overbey, L. (2005). Detecting nonlinearity in
structural systems using the transfer entropy. Physi-
cal Review E, 72(4):046217.
Niriayo, Y. L., Mamo, A., Gidey, K., and Demoz, G. T.
(2019). Medication belief and adherence among pa-
tients with epilepsy. Behavioural neurology, 2019.
Olamat, A. E. and Akan, A. (2017). Synchronization analy-
sis of epilepsy data using global field synchronization.
In 2017 25th Signal Processing and Communications
Applications Conference (SIU), pages 1–4.
Organization, W. H. et al. (2017). Atlas: country resources
for neurological disorders 2004. geneva: World health
organization; 2004.
Paxinos, G. and Watson, C. (2013). The Rat Brain in Stereo-
taxic Coordinates. Elsevier. 7th edition.
Pereda, E., Quiroga, R. Q., and Bhattacharya, J. (2005).
Nonlinear multivariate analysis of neurophysiological
signals. Progress in Neurobiology, 77(1):1 – 37.
Proakis, J. G. and Salehi, M. (2001). Digital communica-
tions, volume 4. McGraw-hill New York.
Schreiber, T. and Schmitz, A. (1996). Improved surrogate
BIOSIGNALS 2020 - 13th International Conference on Bio-inspired Systems and Signal Processing
96