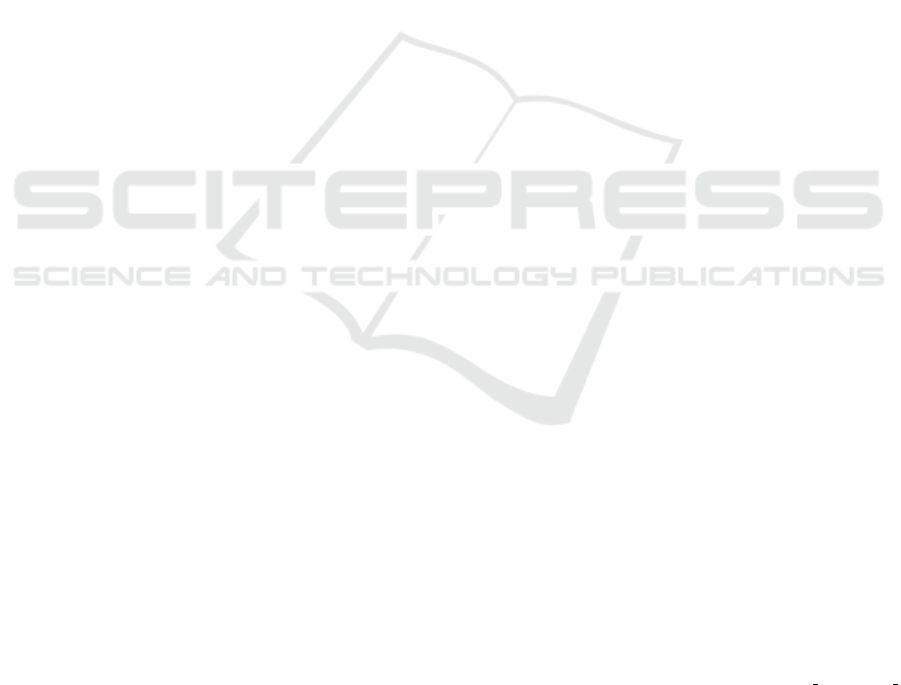
bosacral Spine Using Conventional Technique: Com-
puted Tomography Postoperative Assessment in 102
Consecutive Patients. J. Neurosurg. Spine, 12(3):306–
313.
Bromiley, P. A., Kariki, E. P., Adams, J. E., and Cootes, T. F.
(2016). Fully Automatic Localisation of Vertebrae in
CT Images Using Random Forest Regression Voting.
In Comput. Methods Clin. Appl. Spine Imaging, pages
51–63.
Chen, H., Shen, C., Qin, J., Ni, D., Shi, L., Cheng, J.
C. Y., and Heng, P.-A. (2015). Automatic Localiza-
tion and Identification of Vertebrae in Spine CT via
a Joint Learning Model with Deep Neural Networks.
In Proc. Med. Image Comput. Comput. Interv., pages
515–522.
Chu, C., Belav
´
y, D. L., Armbrecht, G., Bansmann, M.,
Felsenberg, D., and Zheng, G. (2015). Fully Auto-
matic Localization and Segmentation of 3D Vertebral
Bodies from CT/MR Images via a Learning-Based
Method. PLoS One, 10(11):e0143327.
Cootes, T. F., Taylor, C. J., Cooper, D. H., and Graham,
J. (1995). Active Shape Models-Their Training and
Application. Comput. Vis. Image Underst., 61(1):38–
59.
Ebner, T.,
ˇ
Stern, D., Donner, R., Bischof, H., and Urschler,
M. (2014). Towards Automatic Bone Age Estima-
tion from MRI: Localization of 3D Anatomical Land-
marks. In Proc. Med. Image Comput. Comput. Interv.,
pages 421–428.
Forsberg, D., Lundstr
¨
om, C., Andersson, M., Vavruch, L.,
Tropp, H., and Knutsson, H. (2013). Fully Auto-
matic Measurements of Axial Vertebral Rotation for
Assessment of Spinal Deformity in Idiopathic Scolio-
sis. Phys. Med. Biol., 58(6):1775–1787.
Glocker, B., Feulner, J., Criminisi, A., Haynor, D. R., and
Konukoglu, E. (2012). Automatic Localization and
Identification of Vertebrae in Arbitrary Field-of-View
CT Scans. In Proc. Med. Image Comput. Comput. In-
terv., pages 590–598.
Glocker, B., Zikic, D., Konukoglu, E., Haynor, D. R., and
Criminisi, A. (2013). Vertebrae Localization in Patho-
logical Spine CT via Dense Classification from Sparse
Annotations. In Proc. Med. Image Comput. Comput.
Interv., pages 262–270.
Hammernik, K., Ebner, T., Stern, D., Urschler, M., and
Pock, T. (2015). Vertebrae Segmentation in 3D CT
Images Based on a Variational Framework. In Com-
put. Methods Clin. Appl. Spine Imaging, pages 227–
233.
Ibragimov, B., Likar, B., Pernu
ˇ
s, F., and Vrtovec, T. (2014).
Shape Representation for Efficient Landmark-Based
Segmentation in 3-D. IEEE Trans. Med. Imaging,
33(4):861–874.
Janssens, R., Zeng, G., and Zheng, G. (2018). Fully Au-
tomatic Segmentation of Lumbar Vertebrae from CT
Images Using Cascaded 3D Fully Convolutional Net-
works. In Proc. Int. Symp. Biomed. Imaging, pages
893–897. IEEE.
Kingma, D. P. and Ba, J. (2015). Adam: A Method for
Stochastic Optimization. Int. Conf. Learn. Represent.
arXiv1412.6980.
Klinder, T., Ostermann, J., Ehm, M., Franz, A., Kneser, R.,
and Lorenz, C. (2009). Automated Model-Based Ver-
tebra Detection, Identification, and Segmentation in
CT images. Med. Image Anal., 13(3):471–482.
Knez, D., Likar, B., Pernus, F., and Vrtovec, T. (2016).
Computer-Assisted Screw Size and Insertion Trajec-
tory Planning for Pedicle Screw Placement Surgery.
IEEE Trans. Med. Imaging, 35(6):1420–1430.
Korez, R., Likar, B., Pernu
ˇ
s, F., and Vrtovec, T. (2016).
Model-Based Segmentation of Vertebral Bodies from
MR Images with 3D CNNs. In Proc. Med. Image
Comput. Comput. Interv., pages 433–441.
LeCun, Y., Bengio, Y., and Hinton, G. (2015). Deep Learn-
ing. Nature, 521(7553):436–444.
Lessmann, N., van Ginneken, B., de Jong, P. A., and I
ˇ
sgum,
I. (2019). Iterative Fully Convolutional Neural Net-
works for Automatic Vertebra Segmentation and Iden-
tification. Med. Image Anal., 53:142–155.
Liao, H., Mesfin, A., and Luo, J. (2018). Joint Vertebrae
Identification and Localization in Spinal CT Images
by Combining Short- and Long-Range Contextual In-
formation. IEEE Trans. Med. Imaging, 37(5):1266–
1275.
Lindner, C., Bromiley, P. A., Ionita, M. C., and Cootes, T. F.
(2015). Robust and Accurate Shape Model Match-
ing Using Random Forest Regression-Voting. IEEE
Trans. Pattern Anal. Mach. Intell., 37(9):1862–1874.
Mader, A. O., Lorenz, C., von Berg, J., and Meyer, C.
(2019). Automatically Localizing a Large Set of Spa-
tially Correlated Key Points: A Case Study in Spine
Imaging. In Proc. Med. Image Comput. Comput. In-
terv., pages 384–392.
Nesterov, Y. (1983). A Method of Solving A Convex Pro-
gramming Problem With Convergence rate O(1/kˆ2).
In Sov. Math. Dokl., volume 27, pages 372–376.
Payer, C.,
ˇ
Stern, D., Bischof, H., and Urschler, M. (2016).
Regressing Heatmaps for Multiple Landmark Local-
ization Using CNNs. In Proc. Med. Image Comput.
Comput. Interv., pages 230–238.
Payer, C.,
ˇ
Stern, D., Bischof, H., and Urschler, M. (2019).
Integrating Spatial Configuration into Heatmap Re-
gression Based CNNs for Landmark Localization.
Med. Image Anal., 54:207–219.
Pfister, T., Charles, J., and Zisserman, A. (2015). Flowing
ConvNets for Human Pose Estimation in Videos. In
Proc. Int. Conf. Comput. Vis., pages 1913–1921.
Ronneberger, O., Fischer, P., and Brox, T. (2015). U-Net:
Convolutional Networks for Biomedical Image Seg-
mentation. In Proc. Med. Image Comput. Comput. In-
terv., pages 234–241.
Sekuboyina, A. (2019). VerSe 19: Evaluation Re-
port. https://deep-spine.de/verse/verse19 evaluation
report.pdf. Accessed: 2019-11-14.
Sekuboyina, A., Rempfler, M., Kuka
ˇ
cka, J., Tetteh, G.,
Valentinitsch, A., Kirschke, J. S., and Menze, B. H.
(2018). Btrfly Net: Vertebrae Labelling with Energy-
Based Adversarial Learning of Local Spine Prior.
Proc. Med. Image Comput. Comput. Interv., pages
649–657.
VISAPP 2020 - 15th International Conference on Computer Vision Theory and Applications
132