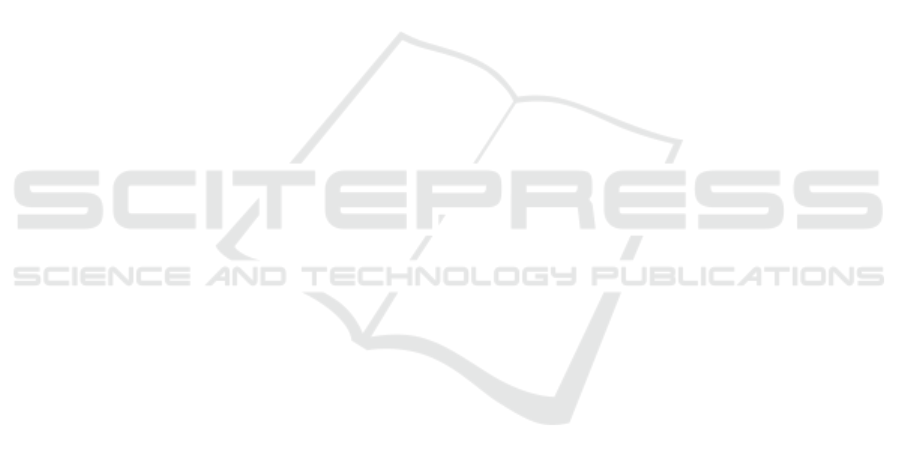
Labby et al. (Labby et al., 2013) report relative
95% limits of agreement between five observers span-
ning 311% for area measurement of MPM tumours,
across 31 subjects. Although we report volumetric
measurement, the 95% limits of agreement in this
evaluation span just 129.2%. However, we note that
this is only comparing against a single observer; the
same observer used to train the model. Labby et al.
also includes figures showing how different observers
consistently annotate differently, i.e. some observers
consistently segment less tumour than others.
For the task of MPM segmentation, where the dis-
ease characteristics can vary dramatically between sub-
jects, time-points and observers, performance of an
algorithm depends heavily on the training and testing
cohort. An increased variance between subjects means
that a large and diverse test set is required to truly es-
tablish whether any automated method can generalise
to unseen cases. A potential limitation of this work
is that we have demonstrated the performance of the
algorithm on 80 subjects which have not undergone
treatment for the disease, all from imaging centres
based in Glasgow, annotated by a single observer. Al-
though this is an unusually large cohort for which to
have full volume annotation of MPM tumour, we ex-
pect that a large, independent and varied test set by
multiple observers is still necessary to truly determine
the performance of this algorithm.
4.3 Future Work
The automated algorithm will shortly be evaluated on
the remaining unseen evaluation datasets, acquired
from multiple institutions (only 123/403 datasets were
used in the internal validation). This evaluation will
determine whether the algorithm performance exceeds
that of the current clinical standard mRECIST scor-
ing system. Cross-validation can only tell us so much
about the performance of an algorithm. The future
external validation will also provide a more realis-
tic and unbiased assessment of its performance using
data from multiple independent centres not involved
in training the algorithm. In addition, inter- and intra-
observer repeatability measurements for these subjects
will provide further context for the performance of this
algorithm.
5 CONCLUSIONS
We have performed an internal validation to explore
the utility of a deep learning approach for fully auto-
mated measurements of MPM in CT images. Binary
closing was found to improve the inter-slice consis-
tency of manual annotations. Following binary closing
there was no significant mean difference between the
manual and automated measurements. To our knowl-
edge, this is the first volumetric evaluation of a fully
automated system to segment pleural volume. The
next step will be to evaluate the method on the re-
maining unseen multi-centre evaluation set. Such an
algorithm has possible future application to pharma-
ceutical trials (where it offers a repeatable study end
point) and to routine care (where it allows tumour pro-
gression to be assessed rapidly to enhance therapeutic
clinical decision making).
REFERENCES
Armato, S. G., Nowak, A. K., Francis, R. J., Kocherginsky,
M., and Byrne, M. J. (2014). Observer variability in
mesothelioma tumor thickness measurements: Defin-
ing minimally measurable lesions. Journal of Thoracic
Oncology.
Attanoos, R. L. and Gibbs, A. R. (1997). Pathology of
malignant mesothelioma. Histopathology, 30(5):403–
418.
Blyth, K., Kidd, A., Winter, A., Baird, W., Dick, C., Hair, J.,
Bylesjo, M., Lynagh, S., Sloan, W., Cowell, G., Noble,
C., Smith, A., Westwood, P., Hopkins, T., Williams, N.,
Walter, H., King, A., and Fennell, D. (2018). An update
regarding the Prediction of ResIstance to chemotherapy
using Somatic copy number variation in Mesothelioma
(PRISM) study. Lung Cancer.
Brahim, W., Mestiri, M., Betrouni, N., and Hamrouni, K.
(2018). Malignant pleural mesothelioma segmentation
for photodynamic therapy planning. Computerized
Medical Imaging and Graphics.
Byrne, M. J. and Nowak, A. K. (2004). Modified RECIST
criteria for assessment of response in malignant pleural
mesothelioma. Annals of Oncology.
Chaisaowong, K., Akkawutvanich, C., Wilkmann, C., and
Kraus, T. (2013). A fully automatic probabilistic 3D
approach for the detection and assessment of pleural
thickenings from CT data. In Computational Intelli-
gence in Medical Imaging.
Chen, M., Helm, E., Joshi, N., Gleeson, F., and Brady, M.
(2017). Computer-aided volumetric assessment of ma-
lignant pleural mesothelioma on CT using a random
walk-based method. International Journal of Computer
Assisted Radiology and Surgery.
Chollet, F. (2015). Keras.
Eisenhauer, E. A., Therasse, P., Bogaerts, J., Schwartz, L. H.,
Sargent, D., Ford, R., Dancey, J., Arbuck, S., Gwyther,
S., Mooney, M., Rubinstein, L., Shankar, L., Dodd, L.,
Kaplan, R., Lacombe, D., and Verweij, J. (2009). New
response evaluation criteria in solid tumours: Revised
RECIST guideline (version 1.1). European Journal of
Cancer.
Frauenfelder, T., Tutic, M., Weder, W., G
¨
otti, R. P., Stahel,
R. A., Seifert, B., and Opitz, I. (2011). Volumetry: An
BIOIMAGING 2020 - 7th International Conference on Bioimaging
72