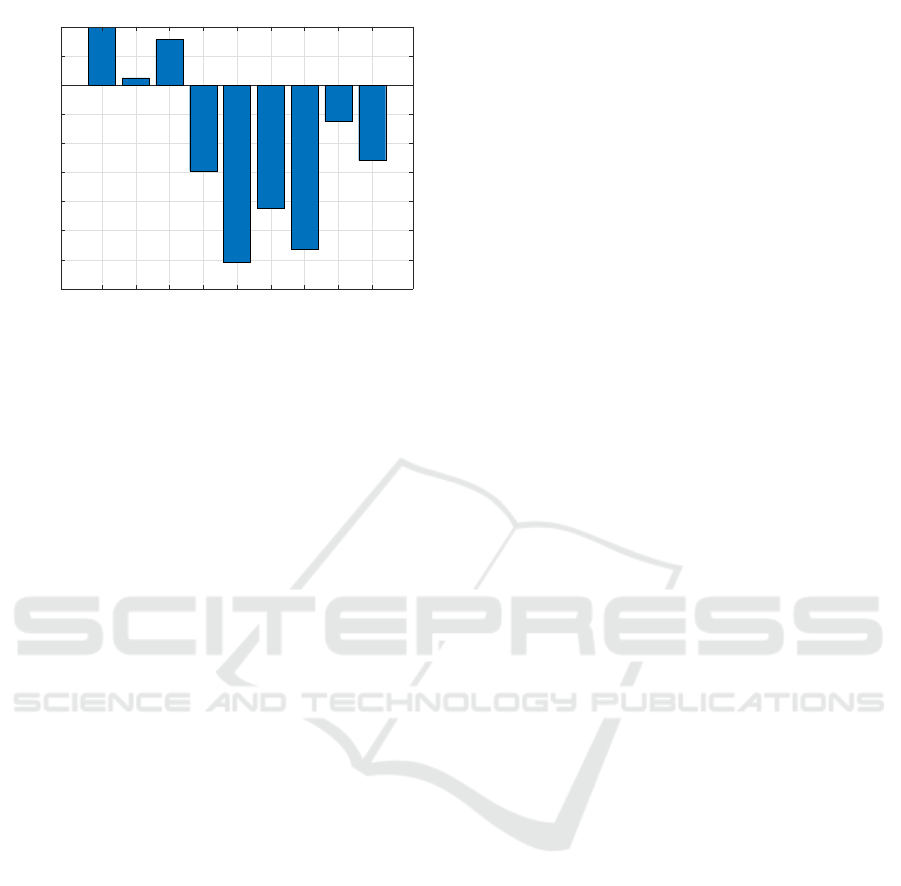
Relevance Of Each Features of Plethysmogram
SDPTGAI
heartRate
RRInterval
logHF
logLF
logLFPHF
MeanHR
Lyapunov
entropy
Feature of Plethysmogram
-1.4
-1.2
-1
-0.8
-0.6
-0.4
-0.2
0
0.2
0.4
Log of length scale
Figure 12: Feature Selection by using ARD with the GPR.
sion accuracy by selecting good quality data sets
based on standard deviation from GPR or finding
other criteria for discriminating low-level mental
states.
ACKNOWLEDGEMENTS
The author would like to thank Mr. Gesshi Higashida,
TAOS Institute, Inc. R & D Department for his tech-
nical support in the experiments.
REFERENCES
Alafeef, M. (2017). Smartphone-based photoplethysmo-
graphic imaging for heart rate monitoring. Journal of
Medical Engineering & Technology, 41(5):387–395.
PMID: 28300460.
Allen, J. (2007). Photoplethysmography and it application
in clinical physiological measurement. Physiological
measurement, 28:R1–39.
Cho, Y., Julier, S. J., and Bianchi-Berthouze, N. (2019).
Instant stress: Detection of perceived mental stress
through smartphone photoplethysmography and ther-
mal imaging. JMIR Ment Health, 6(4):e10140.
Kimura, S., Fukuomoto, M., and Horikoshi, T. (2013).
Eyeglass-based hands-free videophone. Proceedings
of the 2013 International Symposium on Wearable
Computers, pages 117–124.
Loube, D. I., Andrada, T., and Howard, R. S. (1999). Accu-
racy of respiratory inductive plethysmography for the
diagnosis of upper airway resistance syndrome. Chest,
115(5):1333 – 1337.
Mohamed, E. (2012). On the analysis of fingertip photo-
plethysmogram signals. Current cardiology reviews
vol. 8,1 14-25.
Murata, K., Fujita, E., Kojima, S., Maeda, S., Ogura, Y.,
Kamei, T., Tsuji, T., Kaneko, S., Yoshizumi, M., and
Suzuki, N. (2011). Noninvasive biological sensor sys-
tem for detection of drunk driving. IEEE Transactions
on Information Technology in Biomedicine, 15(1):19–
25.
Oyama-Higa, M. and Miao, T. (2005). Representation
of a physio-psychological index through constellation
graphs. In ICNC (1)’05, pages 811–817.
Oyama-Higa, M., Miao, T., and Mizuno-Matsumoto, Y.
(2006). Analysis of dementia in aged subjects through
chaos analysis of fingertip pulse waves. In 2006 IEEE
International Conference on Systems, Man and Cy-
bernetics, volume 4, pages 2863–2867.
Rasmussen, B. C. E. and Williams, C. K. I. (2006). Gaus-
sian Processes for Machine Learning . The MIT Press.
Romem, A., Romem, A., Koldobskiy, D., and Schar
(2014). Diagnosis of obstructive sleep apnea using
pulse oximeter derived photoplethysmographic sig-
nals. Journal of Clinical Sleep Medicine, 10(3):285–
290.
Sano, M. and Sawada, Y. (1985). Measurement of the lya-
punov spectrum from a chaotic time series. Phys. Rev.
Lett., 55:1082–1085.
Shaffer, F. and Ginsberg, J. P. (2017). An overview of heart
rate variability metrics and norms. Frontiers in Public
Health, 5:258.
Smola, A. J. and Sch
¨
olkopf, B. (2004). A tutorial on
support vector regression. Statistics and Computing,
14(3):199–222.
Sumida, T., Arimitu, Y., Tahara, T., and Iwanaga, H. (2000).
Mental conditions reflected by the chaos of pulsation
in capillary vessels. International Journal of Bifurca-
tion and Chaos, 10(09):2245–2255.
Tsuda, I., Tahara, T., and Iwanaga, H. (1992). Chaotic pul-
sation in human capillary vessels and its dependence
on mental and physical conditions. International Jour-
nal of Bifurcation and Chaos, 02(02):313–324.
Vicente, J., Laguna, P., Bartra, A., and Bail
´
on, R. (2016).
Drowsiness detection using heart rate variability.
Medical & Biological Engineering & Computing,
54(6):927–937.
Wipf, D. P. and Nagarajan, S. S. (2008). A new view of au-
tomatic relevance determination. In Platt, J. C., Koller,
D., Singer, Y., and Roweis, S. T., editors, Advances
in Neural Information Processing Systems 20, pages
1625–1632. Curran Associates, Inc.
Wong, W., Bartels, M., and Chrobot, N. (2014). Practi-
cal eye tracking of the ecommerce website user ex-
perience. In Stephanidis, C. and Antona, M., edi-
tors, Universal Access in Human-Computer Interac-
tion. Design for All and Accessibility Practice, pages
109–118, Cham. Springer International Publishing.
Inferring Low-level Mental States of Mobile Users from Plethysmogram Features by Regression Models based on Kernel Method
257