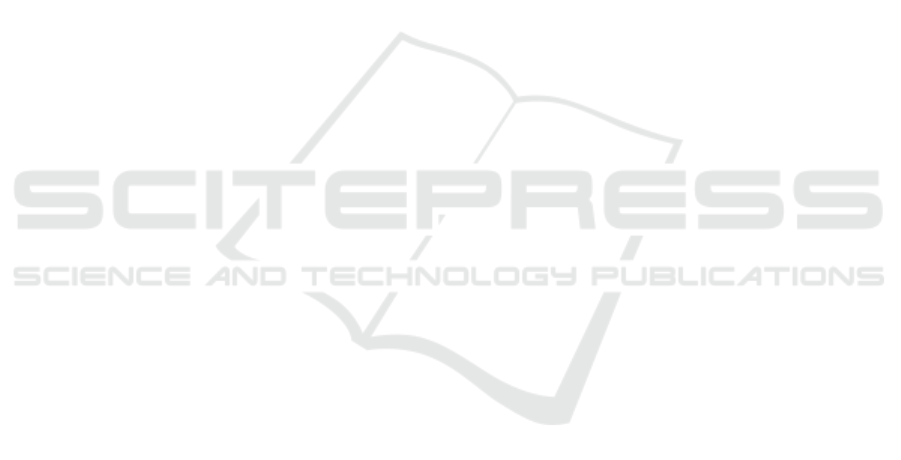
ACKNOWLEDGEMENTS
This work has been partially supported by the
LETSCROWD project, funded by the European
Union Horizon 2020 research and innovation pro-
gramme under grant agreement No 740466, and
by the project ODIS - Optimisation of DIstributed
systems in the Smart-city and smart-grid settings,
CUP:F72F16003170002, funded by Fondazione di
Sardegna. The authors also thank the LETSCROWD
partner Crowd Dynamics for providing some of the
synthetic data sets.
REFERENCES
Abdi, H. (2010). Partial least squares regression and pro-
jection on latent structure regression (pls regression).
Wiley interdisciplinary reviews: computational statis-
tics, 2(1):97–106.
Basak, D., Pal, S., and Patranabis, D. C. (2007). Support
vector regression. Neural Information Processing-
Letters and Reviews, 11(10):203–224.
Benabbas, Y., Ihaddadene, N., Yahiaoui, T., Urruty, T., and
Djeraba, C. (2010). Spatio-temporal optical flow anal-
ysis for people counting. In AVSS, pages 212–217.
Chan, A. B., Liang, Z.-S. J., and Vasconcelos, N. (2008).
Privacy preserving crowd monitoring: Counting peo-
ple without people models or tracking. In CVPR,
pages 1–7.
Change Loy, C., Gong, S., and Xiang, T. (2013). From
semi-supervised to transfer counting of crowds. In
ICCV, pages 2256–2263.
Courty, N., Allain, P., Creusot, C., and Corpetti, T. (2014).
Using the agoraset dataset: Assessing for the quality
of crowd video analysis methods. Pattern Recognition
Letters, 44:161–170.
Criminisi, A., Shotton, J., Konukoglu, E., et al. (2012). De-
cision forests: A unified framework for classification,
regression, density estimation, manifold learning and
semi-supervised learning. Foundations and Trends
R
in Computer Graphics and Vision, 7(2–3):81–227.
Dollar, P., Wojek, C., Schiele, B., and Perona, P. (2011).
Pedestrian detection: An evaluation of the state of the
art. IEEE Trans. on PAMI, 34(4):743–761.
Dong, L., Parameswaran, V., Ramesh, V., and Zoghlami, I.
(2007). Fast crowd segmentation using shape index-
ing. In ICCV, pages 1–8.
Ferryman, J. and Shahrokni, A. (2009). Pets2009: Dataset
and challenge. In PETS, pages 1–6.
Ge, W. and Collins, R. T. (2009). Marked point processes
for crowd counting. In CVPR, pages 2913–2920.
Haralick, R. M., Shanmugam, K., et al. (1973). Textural
features for image classification. IEEE Trans. on sys-
tems, man, and cybernetics, SMC-3(6):610–621.
Kong, D., Gray, D., and Tao, H. (2005). Counting pedes-
trians in crowds using viewpoint invariant training. In
BMVC, pages 1–10.
Leibe, B., Seemann, E., and Schiele, B. (2005). Pedestrian
detection in crowded scenes. In CVPR, pages 878–
885.
Lempitsky, V. and Zisserman, A. (2010). Learning to count
objects in images. In Advances in neural information
processing systems, pages 1324–1332.
Lin, S.-F., Chen, J.-Y., and Chao, H.-X. (2001). Estimation
of number of people in crowded scenes using perspec-
tive transformation. IEEE Trans. on Systems, Man,
and Cybernetics, 31(6):645–654.
Liu, X., Van De Weijer, J., and Bagdanov, A. D. (2019).
Exploiting unlabeled data in cnns by self-supervised
learning to rank. Trans. on PAMI, 41(8):1862–1878.
Loy, C. C., Chen, K., Gong, S., and Xiang, T. (2013).
Crowd counting and profiling: Methodology and eval-
uation. In Modeling, simulation and visual analysis of
crowds, pages 347–382. Springer.
Ma, R., Li, L., Huang, W., and Tian, Q. (2004). On
pixel count based crowd density estimation for visual
surveillance. In CIS, pages 170–173.
Ojala, T., Pietik
¨
ainen, M., and M
¨
aenp
¨
a
¨
a, T. (2002). Mul-
tiresolution gray-scale and rotation invariant texture
classification with local binary patterns. IEEE Trans.
on PAMI, 24(7):971–987.
Ryan, D., Denman, S., Sridharan, S., and Fookes, C. (2015).
An evaluation of crowd counting methods, features
and regression models. Computer Vision and Image
Understanding, 130:1–17.
Schr
¨
oder, G., Senst, T., Bochinski, E., and Sikora, T. (2018).
Optical flow dataset and benchmark for visual crowd
analysis. In AVSS, pages 1–6.
Sindagi, V. and Patel, V. M. (2017a). A survey of recent
advances in cnn-based single image crowd counting
and density estimation. Pattern Recognition Letters,
107:3–16.
Sindagi, V. A. and Patel, V. M. (2017b). Cnn-based cas-
caded multi-task learning of high-level prior and den-
sity estimation for crowd counting. In AVSS, pages
1–6.
Tu, P., Sebastian, T., Doretto, G., Krahnstoever, N.,
Rittscher, J., and Yu, T. (2008). Unified crowd seg-
mentation. In ECCV, pages 691–704. Springer.
Wang, Q., Gao, J., Lin, W., and Yuan, Y. (2019). Learning
from synthetic data for crowd counting in the wild. In
CVPR, pages 8198–8207.
Wu, X., Liang, G., Lee, K. K., and Xu, Y. (2006). Crowd
density estimation using texture analysis and learning.
In ROBIO, pages 214–219.
Zhang, C., Li, H., Wang, X., and Yang, X. (2015). Cross-
scene crowd counting via deep convolutional neural
networks. In CVPR, pages 833–841.
Zhang, Q. and Chan, A. B. (2019). Wide-area crowd count-
ing via ground-plane density maps and multi-view fu-
sion cnns. In CVPR, page 8297–8306.
Zhang, Y., Zhou, C., Chang, F., and Kot, A. C. (2019). A
scale adaptive network for crowd counting. Neuro-
computing, 362:139–146.
Zhang, Y., Zhou, D., Chen, S., Gao, S., and Ma, Y. (2016).
Single-image crowd counting via multi-column con-
volutional neural network. In CVPR, pages 589–597.
VISAPP 2020 - 15th International Conference on Computer Vision Theory and Applications
372