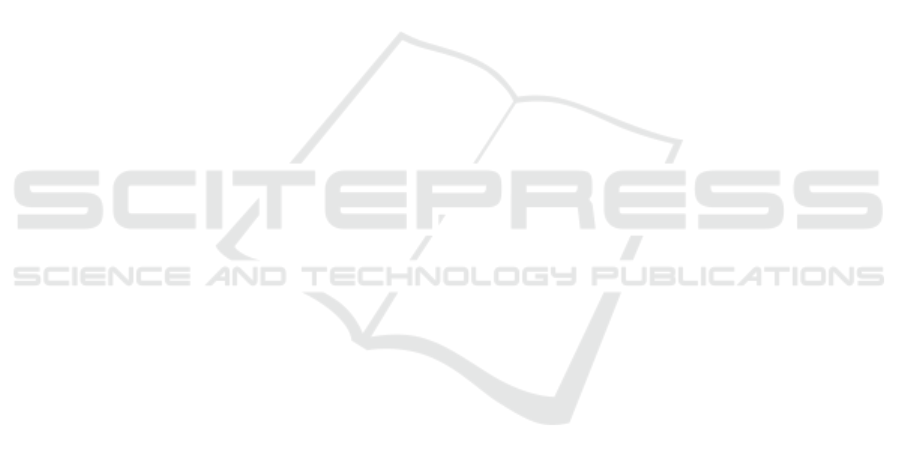
5 CONCLUSIONS
We have presented MORPHYTHM, an approach based
on machine learning that combines rhythmic and mor-
phological features extracted from ECG data to de-
tect AF events. We compared MORPHYTHM with the
method introduced by Zhou et al. (2015), the most
accurate on the AFDB in the literature. The results
show that (i) MORPHYTHM globally achieves better
results compared to the baseline, since it is able to
correctly classify about 4,400 more heart beats, and
(ii) that some of the patients for which all the fibril-
lating heart beat were mis-classified by the baseline
were correctly classified by MORPHYTHM.
The improvement achieved is promising; how-
ever, there is still much room for improving the ac-
curacy. In order to increase the generalizability of
our results, we aim at apply in the future at least one
classifier for each family. In this work, for exam-
ple, we did not consider Bayesian networks, Rules-
based classifiers, and Neural Networks. Also, to max-
imize the accuracy of MORPHYTHM, it would be de-
sirable to use feature selection, to remove useless fea-
tures that could decrease the classification accuracy.
Specifically, since morphological features can be very
patient-dependent, it could be useful performing fea-
ture selection for each single patient rather than glob-
ally. Finally, we plan to perform a cost-benefit anal-
ysis. Indeed, in some online applications, it could be
necessary to have some constraints, such as the total
reduction of FN, even if the FP rate increases. Thus,
we would like to study this specific scenario and ob-
serve if the application of a cost-benefit analysis can
suite some specific constraints.
REFERENCES
Asgari, S., Mehrnia, A., and Moussavi, M. (2015). Au-
tomatic detection of atrial fibrillation using stationary
wavelet transform and support vector machine. Com-
puters in biology and medicine, 60:132–142.
Balestrieri, E., Boldi, F., Colavita, A. R., De Vito, L.,
Laudato, G., Oliveto, R., Picariello, F., Rivaldi, S.,
Scalabrino, S., Torchitti, P., et al. (2019). The ar-
chitecture of an innovative smart t-shirt based on the
internet of medical things paradigm. In 2019 IEEE
International Symposium on Medical Measurements
and Applications (MeMeA), pages 1–6. IEEE.
Barandiaran, I. (1998). The random subspace method for
constructing decision forests. IEEE Trans. Pattern
Anal. Mach. Intell, 20(8):1–22.
Camm, A. J., Corbucci, G., and Padeletti, L. (2012). Use-
fulness of continuous electrocardiographic monitoring
for atrial fibrillation. The American journal of cardi-
ology, 110(2):270–276.
Capucci, A., Calcagnini, G., Mattei, E., Triventi, M., Bar-
tolini, P., Biancalana, G., Gargaro, A., Puglisi, A., and
Censi, F. (2012). Daily distribution of atrial arrhyth-
mic episodes in sick sinus syndrome patients: impli-
cations for atrial arrhythmia monitoring. Europace,
14(8):1117–1124.
Censi, F., Calcagnini, G., Mattei, E., Gargaro, A., Bian-
calana, G., and Capucci, A. (2013). Simulation of
monitoring strategies for atrial arrhythmia detection.
Annali dell’Istituto superiore di sanita, 49:176–182.
Chou, T., Tamura, Y., and Wong, I. (2016). Detection of
atrial fibrillation in ecgs. Computer Methods and Pro-
grams in Biomedicine, 136:143–50.
Clifford, G. D., Azuaje, F., McSharry, P., et al. (2006).
Advanced methods and tools for ECG data analysis.
Artech house Boston.
Colloca, R., Johnson, A. E., Mainardi, L., and Clifford,
G. D. (2013). A support vector machine approach for
reliable detection of atrial fibrillation events. In Com-
puting in Cardiology 2013, pages 1047–1050. IEEE.
Cramer, J. S. (2002). The origins of logistic regression
(technical report). In Tinbergen Institute.
Devasena, C. L. (2014). Comparative analysis of random
forest rep tree and j48 classifiers for credit risk predic-
tion. In International Conference on Communication,
Computing and Information Technology (ICCCMIT-
2014).
Freund, Y. and Schapire, R. E. (1997). A decision-theoretic
generalization of on-line learning and an application
to boosting. Journal of computer and system sciences,
55(1):119–139.
Goldberger, A. L., Amaral, L. A., Glass, L., Hausdorff,
J. M., Ivanov, P. C., Mark, R. G., Mietus, J. E., Moody,
G. B., Peng, C.-K., and Stanley, H. E. (2000). Phys-
iobank, physiotoolkit, and physionet: components of
a new research resource for complex physiologic sig-
nals. Circulation, 101(23):e215–e220.
Haque, A. F., Ali, M. H., Kiber, M. A., and Hasan+, M. T.
(2009). Automatic feature extraction of ecg signal us-
ing fast fourier transform.
Huang, C., Ye, S., Chen, H., Li, D., He, F., and
Tu, Y. (2010). A novel method for detection of
the transition between atrial fibrillation and sinus
rhythm. IEEE Transactions on Biomedical Engineer-
ing, 58(4):1113–1119.
January, C. T., Wann, L. S., Alpert, J. S., Calkins, H.,
Cigarroa, J. E., Cleveland, J. C., Conti, J. B., Ellinor,
P. T., Ezekowitz, M. D., Field, M. E., et al. (2014).
2014 aha/acc/hrs guideline for the management of pa-
tients with atrial fibrillation: a report of the american
college of cardiology/american heart association task
force on practice guidelines and the heart rhythm so-
ciety. Journal of the American College of Cardiology,
64(21):e1–e76.
Kearley, K., Selwood, M., Van den Bruel, A., Thompson,
M., Mant, D., Hobbs, F. R., Fitzmaurice, D., and
Heneghan, C. (2014). Triage tests for identifying
atrial fibrillation in primary care: a diagnostic accu-
racy study comparing single-lead ecg and modified bp
monitors. BMJ open, 4(5):e004565.
HEALTHINF 2020 - 13th International Conference on Health Informatics
164