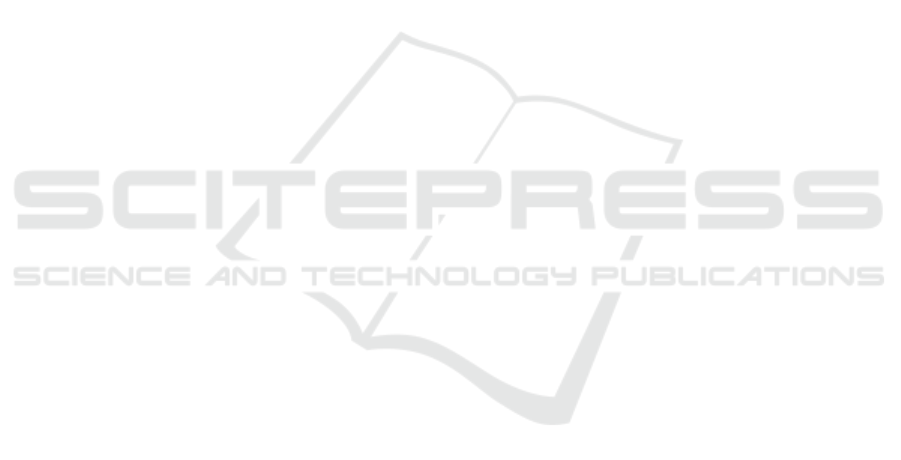
get scene differs from the ones used for training only
in the background or in the lighting conditions, but
exhibit similar perspective and scale; their perfor-
mance is considerably worse when target and training
scenes significantly differ in perspective and scale,
instead. As a possible solution to improve cross-
scene effectiveness when no manually annotated data
from the target scene is available, and it is also dif-
ficult to obtain non-annotated data for unsupervised
domain adaptation methods, we envisage the use of
synthetic data sets reproducing the same perspective
of the target scene. We are currently investigating
this approach, and preliminary results can be found
in (Delussu et al., 2020).
As a final remark, the still large gap between
same- and cross-scene performance suggests to avoid
focusing future work on improving crowd counting
accuracy on benchmark data sets under same-scene
scenarios (somewhat according to the suggestions
given in (Torralba et al., 2011) for other computer vi-
sion tasks), and to address the efforts toward achiev-
ing a higher invariance in perspective and scale.
ACKNOWLEDGEMENT
This work was supported by the project “Law
Enforcement agencies human factor methods and
Toolkit for the Security and protection of CROWDs in
mass gatherings” (LETSCROWD), EU Horizon 2020
programme, grant agreement No. 740466.
REFERENCES
Chan, A. B., Liang, Z.-S. J., and Vasconcelos, N. (2008).
Privacy preserving crowd monitoring: Counting peo-
ple without people models or tracking. In IEEE
CVPR, pages 1–7.
Chen, K., Loy, C. C., Gong, S., and Xiang, T. (2012). Fea-
ture mining for localised crowd counting. In BMVC,
pages 1–11.
Delussu, R., Putzu, L., and Fumera, G. (2020). Investigating
synthetic data sets for crowd density estimation. In
VISAPP. In press.
Ferryman, J. and Shahrokni, A. (2009). Pets2009: Dataset
and challenge. In PETS, pages 1–6.
Li, Y., Zhang, X., and Chen, D. (2018). Csrnet: Dilated
convolutional neural networks for understanding the
highly congested scenes. In IEEE CVPR, pages 1091–
1100.
Liu, W., Salzmann, M., and Fua, P. (2019). Context-aware
crowd counting. In IEEE CVPR, pages 5099–5108.
Liu, X., van de Weijer, J., and Bagdanov, A. D. (2018).
Leveraging unlabeled data for crowd counting by
learning to rank. In IEEE CVPR.
Loy, C. C., Chen, K., Gong, S., and Xiang, T. (2013).
Crowd counting and profiling: Methodology and eval-
uation. In Modeling, simulation and visual analysis of
crowds, pages 347–382. Springer.
Marsden, M., McGuinness, K., Little, S., and O’Connor,
N. E. (2017). Resnetcrowd: A residual deep learning
architecture for crowd counting, violent behaviour de-
tection and crowd density level classification. In IEEE
AVSS, pages 1–7.
Onoro-Rubio, D. and L
´
opez-Sastre, R. J. (2016). Towards
perspective-free object counting with deep learning.
In ECCV, pages 615–629. Springer.
Ryan, D., Denman, S., Sridharan, S., and Fookes, C. (2015).
An evaluation of crowd counting methods, features
and regression models. Computer Vision and Image
Understanding, 130:1–17.
Sam, D. B., Sajjan, N. N., Maurya, H., and Babu, R. V.
(2019). Almost unsupervised learning for dense
crowd counting. In Association for the Advancement
of Artificial Intelligence.
Sindagi, V. and Patel, V. M. (2017a). A survey of recent
advances in cnn-based single image crowd counting
and density estimation. Pattern Recognition Letters,
107:3–16.
Sindagi, V. A. and Patel, V. M. (2017b). Cnn-based cas-
caded multi-task learning of high-level prior and den-
sity estimation for crowd counting. In IEEE AVSS,
pages 1–6.
Sindagi, V. A. and Patel, V. M. (2020). HA-CCN: hierar-
chical attention-based crowd counting network. IEEE
Trans. on Image Processing, 29:323–335.
Torralba, A., Efros, A. A., et al. (2011). Unbiased look at
dataset bias. In IEEE CVPR, pages 1521–1528.
Wang, Q., Gao, J., Lin, W., and Yuan, Y. (2019). Learning
from synthetic data for crowd counting in the wild. In
IEEE CVPR, pages 8198–8207.
Zhang, C., Li, H., Wang, X., and Yang, X. (2015). Cross-
scene crowd counting via deep convolutional neural
networks. In IEEE CVPR, pages 833–841.
Zhang, Q. and Chan, A. B. (2019). Wide-area crowd count-
ing via ground-plane density maps and multi-view fu-
sion cnns. In IEEE CVPR, page 8297–8306.
Zhang, Y., Zhou, C., Chang, F., and Kot, A. C. (2019). A
scale adaptive network for crowd counting. Neuro-
computing, 362:139–146.
Zhang, Y., Zhou, D., Chen, S., Gao, S., and Ma, Y. (2016).
Single-image crowd counting via multi-column con-
volutional neural network. In IEEE CVPR, pages 589–
597.
Zou, Z., Su, X., Qu, X., and Zhou, P. (2018). Da-net: Learn-
ing the fine-grained density distribution with defor-
mation aggregation network. IEEE Access, 6:60745–
60756.
VISAPP 2020 - 15th International Conference on Computer Vision Theory and Applications
380