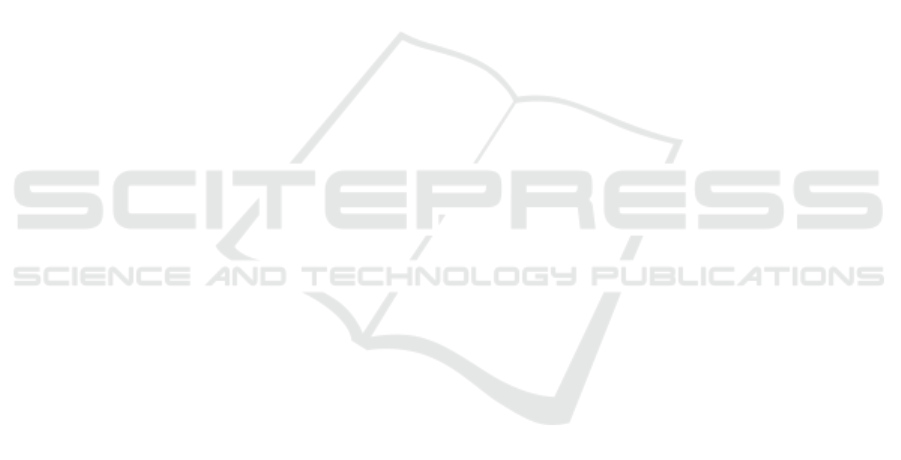
Technological Innovation (CONCYTEC-PERU). I
thank all the people who directly or indirectly helped
me with this work.
REFERENCES
Agrawal, A., Raskar, R., Nayar, S. K., and Li, Y. (2005).
Removing photography artifacts using gradient pro-
jection and flash-exposure sampling. ACM Trans.
Graph., 24(3):828–835.
Aksoy, Y., Kim, C., Kellnhofer, P., Paris, S., Elgharib, M.,
Pollefeys, M., and Matusik, W. (2018). A dataset of
flash and ambient illumination pairs from the crowd.
In Proceedings of the European Conference on Com-
puter Vision (ECCV), pages 634–649.
Capece, N., Banterle, F., Cignoni, P., Ganovelli, F.,
Scopigno, R., and Erra, U. (2019). Deepflash: Turning
a flash selfie into a studio portrait. Signal Processing:
Image Communication, 77:28 – 39.
Chen, C., Chen, Q., Xu, J., and Koltun, V. (2018). Learn-
ing to see in the dark. In The IEEE Conference on
Computer Vision and Pattern Recognition (CVPR).
Deng, J., Dong, W., Socher, R., Li, L.-J., Li, K., and Fei-
Fei, L. (2009). Imagenet: A large-scale hierarchical
image database. In 2009 IEEE conference on com-
puter vision and pattern recognition, pages 248–255.
Ieee.
Eisemann, E. and Durand, F. (2004). Flash photography
enhancement via intrinsic relighting. ACM Trans.
Graph., 23(3):673–678.
Fu, X., Zeng, D., Huang, Y., Zhang, X.-P., and Ding, X.
(2016). A weighted variational model for simultane-
ous reflectance and illumination estimation. In The
IEEE Conference on Computer Vision and Pattern
Recognition (CVPR).
Goodfellow, I., Pouget-Abadie, J., Mirza, M., Xu, B.,
Warde-Farley, D., Ozair, S., Courville, A., and Ben-
gio, Y. (2014). Generative adversarial nets. In
Advances in neural information processing systems,
pages 2672–2680.
Guo, X., Li, Y., and Ling, H. (2017). Lime: Low-light
image enhancement via illumination map estimation.
IEEE Transactions on Image Processing, 26(2):982–
993.
Isola, P., Zhu, J.-Y., Zhou, T., and Efros, A. A. (2017).
Image-to-image translation with conditional adversar-
ial networks. In The IEEE Conference on Computer
Vision and Pattern Recognition (CVPR).
Kingma, D. P. and Ba, J. (2015). Adam: A method for
stochastic optimization. In 3rd International Confer-
ence on Learning Representations, ICLR 2015, San
Diego, CA, USA, May 7-9, 2015, Conference Track
Proceedings.
Long, J., Shelhamer, E., and Darrell, T. (2015). Fully con-
volutional networks for semantic segmentation. In
2015 IEEE Conference on Computer Vision and Pat-
tern Recognition (CVPR), pages 3431–3440.
Mirza, M. and Osindero, S. (2014). Conditional generative
adversarial nets. arXiv preprint arXiv:1411.1784.
Parkhi, O. M., Vedaldi, A., and Zisserman, A. (2015). Deep
face recognition. In Xianghua Xie, M. W. J. and
Tam, G. K. L., editors, Proceedings of the British Ma-
chine Vision Conference (BMVC), pages 41.1–41.12.
BMVA Press.
Petschnigg, G., Szeliski, R., Agrawala, M., Cohen, M.,
Hoppe, H., and Toyama, K. (2004). Digital photogra-
phy with flash and no-flash image pairs. ACM Trans.
Graph., 23(3):664–672.
Ronneberger, O., Fischer, P., and Brox, T. (2015). U-net:
Convolutional networks for biomedical image seg-
mentation. In International Conference on Medical
image computing and computer-assisted intervention,
pages 234–241. Springer.
Shen, L., Yeo, C., and Hua, B. (2013). Intrinsic image
decomposition using a sparse representation of re-
flectance. IEEE Transactions on Pattern Analysis and
Machine Intelligence, 35(12):2904–2915.
Simonyan, K. and Zisserman, A. (2015). Very deep con-
volutional networks for large-scale image recognition.
In International Conference on Learning Representa-
tions.
VISAPP 2020 - 15th International Conference on Computer Vision Theory and Applications
388