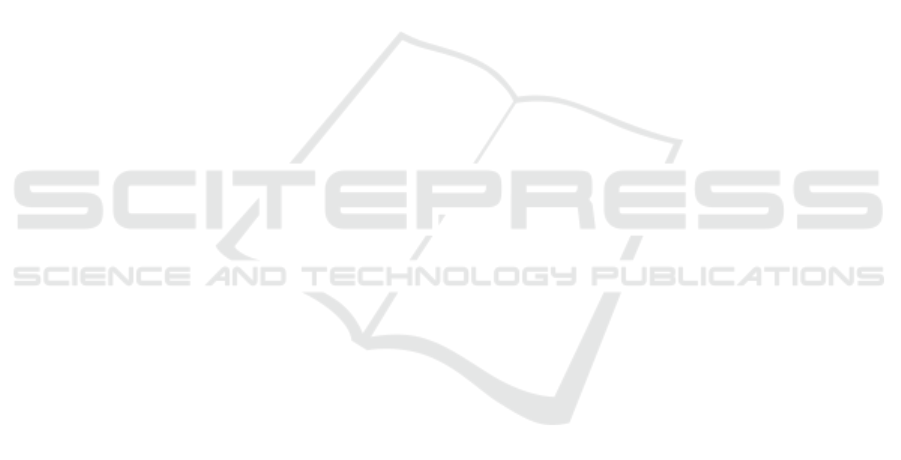
cerebellum and brainstem (detection rate of 0.6829).
Indeed, some lesions seems to be more challenging,
specially in the cerebellum, whose appearances are
similar to their surrounding tissues (Fig. 6, Image
6). However, its FP scores are similar to those of
hemispheric lesions which confirms the stability of
the method (compare rows 2-4 for BADRESC in Ta-
ble 1).
5 CONCLUSIONS
We presented a new unsupervised method for brain
anomaly detection that combines registration errors
and supervoxel classification. Our approach, named
BADRESC, adapts a recent supervoxel-based ap-
proach (SAAD) to detect outliers as anomalies from
registration errors in the hemispheres, cerebellum,
and brainstem. BADRESC was validated on 3T MR-
T1 images of stroke patients with annotated lesions,
attaining similar detection accuracy to SAAD for le-
sions in the hemispheres and substantially less false
positives. BADRESC also detects lesions in the cere-
bellum and brainstem with promising results.
For future work, we intend to improve BADRESC
by optimizing its parameters and using additional vi-
sual analytics techniques to improve seeding and fur-
ther investigate other anomaly features and classifiers
to yield better detection rates, specially for the cere-
bellum and brainstem.
ACKNOWLEDGEMENTS
The authors thank CNPq (303808/2018-7), and
FAPESP (2014/12236-1) for the financial support.
REFERENCES
Akkus, Z. and et al. (2017). Deep learning for brain MRI
segmentation: state of the art and future directions. J
Digit Imaging, 30(4):449–459.
Aslani, S., Dayan, M., Murino, V., and Sona, D.
(2018). Deep 2D encoder-decoder convolutional neu-
ral network for multiple sclerosis lesion segmenta-
tion in brain MRI. In Medical Image Computing
and Computer-Assisted Intervention (MICCAI), pages
132–141.
Fonov, V. S. and et al. (2009). Unbiased nonlinear average
age-appropriate brain templates from birth to adult-
hood. Neuroimage, 47:S102.
Gao, Y., Riklin-Raviv, T., and Bouix, S. (2014). Shape
analysis, a field in need of careful validation. Human
Brain Mapping, 35(10):4965–4978.
Goetz, M. and et al. (2014). Extremely randomized trees
based brain tumor segmentation. Proc. of BRATS
challenge-MICCAI, pages 006–011.
Guo, D. et al. (2015). Automated lesion detection on MRI
scans using combined unsupervised and supervised
methods. BMC Medical Imaging, 15(1):50.
Juan-Albarrac
´
ın, J., Fuster-Garcia, E., Manj
´
on, J. V., Rob-
les, M., Aparici, F., Mart
´
ı-Bonmat
´
ı, L., and Garc
´
ıa-
G
´
omez, J. M. (2015). Automated glioblastoma seg-
mentation based on a multiparametric structured un-
supervised classification. PLoS One, 10(5):e0125143.
Klein, S., Staring, M., Murphy, K., Viergever, M., and
Pluim, J. (2010). elastix: A toolbox for intensity-
based medical image registration. IEEE T Med Imag,
29(1):196–205.
Kooi, T., Litjens, G., Van Ginneken, B., Gubern-M
´
erida, A.,
S
´
anchez, C. I., Mann, R., den Heeten, A., and Karsse-
meijer, N. (2017). Large scale deep learning for com-
puter aided detection of mammographic lesions. Med
Image Anal, 35:303–312.
Liew, S.-L. and et al. (2018). A large, open source dataset
of stroke anatomical brain images and manual lesion
segmentations. Scientific Data, 5:180011.
Manevitz, L. M. and Yousef, M. (2001). One-class SVMs
for document classification. J Mach Learn Res,
2:139–154.
Manj
´
on, J. V. and Coup
´
e, P. (2016). volBrain: An online
MRI brain volumetry system. Front Neuroinform, 10.
Martins, S. B., Bragantini, J., Yasuda, C. L., and Falc
˜
ao,
A. X. (2019a). An adaptive probabilistic atlas for
anomalous brain segmentation in MR images. Med
Phys, 46(11):4940–4950.
Martins, S. B., Ruppert, G., Reis, F., Yasuda, C. L., and
Falc
˜
ao, A. X. (2019b). A supervoxel-based approach
for unsupervised abnormal asymmetry detection in
MR images of the brain. In Proc. IEEE ISBI, pages
882–885.
Martins, S. B., Telea, A. C., and Falc
˜
ao, A. X. (2019c). Ex-
tending supervoxel-based abnormal brain asymmetry
detection to the native image space. In IEEE Eng in
Med Bio Society (EMBC), pages 450–453.
Otsu, N. (1979). A threshold selection method from gray-
level histograms. IEEE Trans. on systems, man, and
cybernetics, 9(1):62–66.
Pereira, S., Pinto, A., Alves, V., and Silva, C. A. (2016).
Brain tumor segmentation using convolutional neu-
ral networks in MRI images. IEEE T Med Imag,
35(5):1240–1251.
Pinto, A., Pereira, S., Correia, H., Oliveira, J., Rasteiro,
D. M., and Silva, C. A. (2015). Brain tumour seg-
mentation based on extremely randomized forest with
high-level features. In IEEE Eng in Med Bio Society
(EMBC), pages 3037–3040.
Qi, K. and et al. (2019). X-Net: Brain stroke lesion
segmentation based on depthwise separable convolu-
tion and long-range dependencies. In Medical Im-
age Computing and Computer-Assisted Intervention
(MICCAI). to appear. Currently available on arXiv
preprint arXiv:1907.07000.
BIOIMAGING 2020 - 7th International Conference on Bioimaging
80