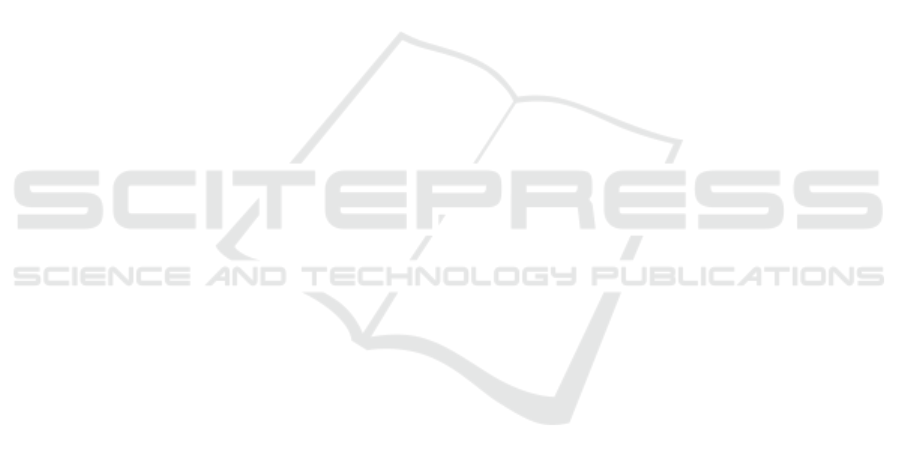
5 CONCLUSION
In this paper, we aimed to solve the problem of rec-
ognizing white cane users by classifying pedestrians
from the temporal transition of their skeletons. We
proposed a 2D skeleton orientation alignment method
named SOANets. Through experiments, we demon-
strated that the accuracy of analyzing pedestrian im-
age sequences improves by incorporating the skeleton
orientation alignment of an input 2D skeleton, and the
effectiveness of the proposed method was confirmed.
In the future, we plan to train SOANets with more
action patterns. We will also integrate object recogni-
tion methods that can directly detect a white cane.
ACKNOWLEDGMENTS
Parts of this research were supported by MEXT,
Grant-in-Aid for Scientific Research
REFERENCES
Baptista, R., Ghorbel, E., Papadopoulos, K., Demisse,
G. G., Aouada, D., and Ottersten, B. (2019). View-
invariant action recognition from RGB data via 3D
pose estimation. In Proceeding of the 2019 IEEE In-
ternational Conference on Acoustics, Speech and Sig-
nal Processing, pages 2542–2546.
Cai, Z. and Vasconcelos, N. (2018). Cascade R-CNN: Delv-
ing into High Quality Object Detection. In Proceeding
of the 2018 IEEE Conference on Computer Vision and
Pattern Recognition, pages 6154–6162.
Cao, Z., Simon, T., Wei, S. E., and Sheikh, Y. (2017). Real-
time multi-person 2D pose estimation using part affin-
ity field. In Proceeding of the 2017 IEEE Conference
on Computer Vision and Pattern Recognition, pages
7291–7299.
He, K., Gkioxari, G., Dollar, P., and Girshick, R. (2017).
Mask R-CNN. In Proceeding of the 2017 IEEE In-
ternational Conference on Computer Vision, pages
2961–2969.
Hochreiter, S. and Schmidhuber, J. (1997). Long short-term
memory. Neural Computation, 9(8):1735–1780.
Le, T. M., Inoue, N., and Shinoda, K. (2018). A fine-to-
coarse convolutional neural network for 3D human ac-
tion recognition. In Proceeding of the 29th British
Machine Vision Conf, pages 184–1–184–13.
Martinez, J., Hossain, R., Romero, J., and Little, J. J.
(2016). A simple yet effective baseline for 3D hu-
man pose estimation. In Proceeding of the 2017 IEEE
International Conference on Computer Vision, pages
2640–2649.
Nishida, N., Kawanishi, Y., Deguchi, D., Ide, I., Murase,
H., and Piao, J. (2019). Exemplar-based Pseudo-
Viewpoint Rotation for White-Cane User Recognition
from a 2D Human Pose Sequence. In Proceeding of
the 16th IEEE International Conference on Advanced
Video and Signal-based Surveillance, number Paper
ID 29.
Redmon, J. and Farhadi, A. (2018). YOLOv3: An Incre-
mental Improvement. Computing Research Reposi-
tory, (arXiv:1804.02767).
Si, C., Chen, W., Wang, W., Wang, L., and Tan, T. (2019).
An attention enhanced graph convolutional LSTM
network for skeleton-based action recognition. In Pro-
ceeding of the 2019 IEEE Conference on Computer
Vision and Pattern Recognition, pages 1227–1236.
Sun, L., Jia, K., Chen, K., Yeung, D. Y., Shi, B. E., and
Savarese, S. (2017). Lattice long short-term memory
for human action recognition. In Proceeding of the
2017 IEEE International Conference on Computer Vi-
sion, pages 2147–2156.
Tanikawa, U., Kawanishi, Y., Deguchi, D., Ide, I., Murase,
H., and Kawai, R. (2017). Wheelchair-user Detection
Combined with Parts-based Tracking. In Proceed-
ing of the 12th Joint Conference on Computer Vision,
Imaging and Computer Graphics Theory and Appli-
cations, volume 5, pages 165–172.
Wang, C., Wang, Y., and Yuille, A. L. (2016). Mining 3D
key-pose-motifs for action recognition. In Proceeding
of the 2016 IEEE Conference on Computer Vision and
Pattern Recognition, pages 2639–2647.
Wei, S. E., Ramakrishna, V., Kanede, T., and Sheikh, Y.
(2016). Convolutional pose machines. In Proceeding
of the 2016 IEEE Conference on Computer Vision and
Pattern Recognition, pages 4724–4732.
SOANets: Encoder-decoder based Skeleton Orientation Alignment Network for White Cane User Recognition from 2D Human Skeleton
Sequence
443