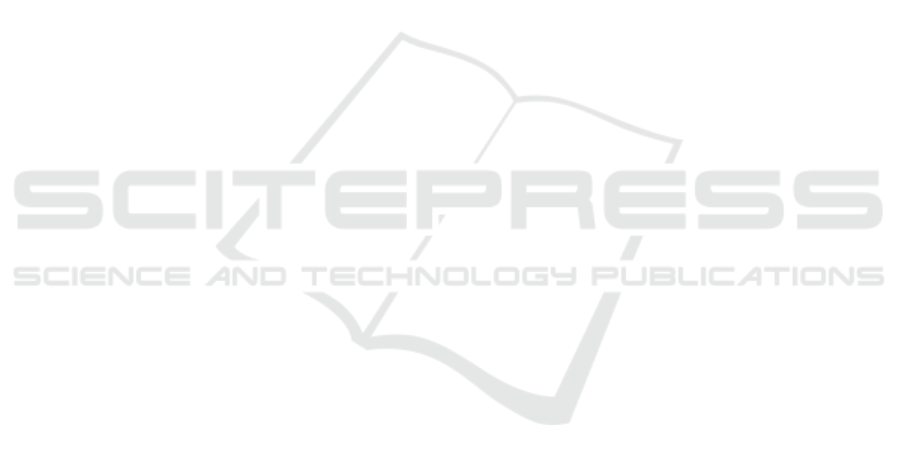
Feigl, T., Mutschler, C., and Philippsen, M. (2018). Super-
vised learning for yaw orientation estimation. In Proc.
Intl. Conf. Indoor Navigation and Positioning (IPIN),
pages 103–113, Nantes, France.
Fraga-Lamas, P., Fern
´
andez-Caram
´
es, T. M., Blanco-
Novoa,
´
O., and Vilar-Montesinos, M. A. (2018). A
review on industrial augmented reality systems for the
industry 4.0 shipyard. In Proc. Intl. Conf. Intelligent
Robots and Systems (IROS), pages 131–139, Madrid,
Spain.
Geiger, A., Lenz, P., Stiller, C., and Urtasun, R. (2013).
Vision meets robotics: The KITTI dataset. Intl. J. of
Robotics Research, 18(17):6908–6926.
Handa, A., Whelan, T., McDonald, J., and Davison, A. J.
(2014). A benchmark for RGB-D visual odometry,
3D reconstruction and SLAM. In Proc. Intl. Conf.
Robotics and Automation (ICRA), pages 1524–1531,
Hong Kong, China.
Kasyanov, A., Engelmann, F., St
¨
uckler, J., and Leibe, B.
(2017). Keyframe-based visual-inertial online SLAM
with relocalization. In Proc. Intl. Conf. Intelligent
Robots and Systems (IROS), pages 6662–6669, Van-
couver, Canada.
Kato, H. and Billinghurst, M. (1999). Marker tracking and
HMD calibration for a video-based augmented reality
conferencing system. In Proc. Intl. Workshop on Aug-
mented Reality (IWAR), pages 85–94, San Francisco,
CA.
Kerl, C., Sturm, J., and Cremers, D. (2013). Dense visual
SLAM for RGB-D cameras. In Proc. Intl. Conf. Intel-
ligent Robots and Systems (IROS), pages 2100–2106,
Tokyo, Japan.
Klein, G. and Murray, D. (2007). Parallel tracking and
mapping for small AR workspaces. In Proc. Intl.
Workshop on Augmented Reality (ISMAR), pages 1–
10, Nara, Japan.
L
¨
offler, C., Riechel, S., Fischer, J., and Mutschler, C.
(2018). Evaluation criteria for inside-out indoor po-
sitioning systems based on machine learning. In Proc.
Intl. Conf. Indoor Positioning and Indoor Navigation
(IPIN), pages 1–8, Nantes, France.
Li, P., Qin, T., Hu, B., Zhu, F., and Shen, S. (2017).
Monocular visual-inertial state estimation for mobile
augmented reality. In Proc. Intl. Conf. Intelligent
Robots and Systems (IROS), pages 11–21, Vancouver,
Canada.
Li, W., Saeedi, S., McCormac, J., Clark, R., Tzoumanikas,
D., Ye, Q., Huang, Y., Tang, R., and Leuteneg-
ger, S. (2018). Interiornet: Mega-scale multi-sensor
photo-realistic indoor scenes dataset. arXiv preprint
arXiv:1809.00716, 18(17).
Linowes, J. and Babilinski, K. (2017). Augmented Real-
ity for Developers: Build practical augmented reality
applications with Unity, ARCore, ARKit, and Vuforia.
Packt Publishing Ltd, Birmingham, UK.
Liu, H., Chen, M., Zhang, G., Bao, H., and Bao, Y.
(2018). Ice-ba: Incremental, consistent and efficient
bundle adjustment for visual-inertial SLAM. In Proc.
Intl. Conf. Computer Vision and Pattern Recognition
(CVPR), pages 1974–1982, Salt Lake City, UT.
Marchand, E., Uchiyama, H., and Spindler, F. (2016). Pose
estimation for augmented reality: A hands-on sur-
vey. Trans. Visualization and Computer Graphics,
22(12):2633–2651.
Marques, B., Carvalho, R., Dias, P., Oliveira, M., Fer-
reira, C., and Santos, B. S. (2018). Evaluating and
enhancing Google Tango localization in indoor en-
vironments using fiducial markers. In Proc. Intl.
Conf. Autonomous Robot Systems and Competitions
(ICARSC), pages 142–147, Torres Vedras, Portugal.
Mur-Artal, R. and Tardos, J. D. (2017). ORB-SLAM2: An
open-source SLAM system for monocular, stereo, and
RGB-D cameras. Trans. Robotics, 33(5):1255–1262.
Neumann, U. and You, S. (1999). Natural feature tracking
for augmented reality. Trans. on Multimedia, 1(1):12–
20.
Palmarini, R., Erkoyuncu, J. A., and Roy, R. (2017). An
innovative process to select augmented reality (AR)
technology for maintenance. In Proc. Intl. Conf. Man-
ufacturing Systems (CIRP), pages 23–28, Taichung,
Taiwan.
Regenbrecht, H., Meng, K., Reepen, A., Beck, S., and Lan-
glotz, T. (2017). Mixed voxel reality: Presence and
embodiment in low fidelity, visually coherent, mixed
reality environments. In Proc. Intl. Conf. Intelligent
Robots and Systems (IROS), pages 90–99, Vancouver,
Canada.
Saputra, M. R. U., Markham, A., and Trigoni, N. (2018).
Visual SLAM and structure from motion in dynamic
environments: A survey. Comput. Surv., 51(2):1–36.
Schubert, D., Goll, T., Demmel, N., Usenko, V., St
¨
ockler,
J., and Cremers, D. (2018). The TUM VI benchmark
for evaluating visual-inertial odometry. In Proc. Intl.
Conf. Intelligent Robots and Systems (IROS), pages
6908–6926, Madrid, Spain.
Simon, G., Fitzgibbon, A., and Zisserman, A. (2000).
Markerless tracking using planar structures in the
scene. In Proc. Intl. Workshop Augmented Reality (IS-
MAR), pages 120–128, Munich, Germany.
Taketomi, T., Uchiyama, H., and Ikeda, S. (2017). Vi-
sual SLAM algorithms: a survey from 2010 to 2016.
Trans. Computer Vision and Applications, 9(1):452–
461.
Terashima, T. and Hasegawa, O. (2017). A visual-SLAM
for first person vision and mobile robots. In Proc. Intl.
Conf. Intelligent Robots and Systems (IROS), pages
73–76, Vancouver, Canada.
Vassallo, R., Rankin, A., Chen, E. C. S., and Peters, T. M.
(2017). Hologram stability evaluation for Microsoft
Hololens. In Proc. Intl. Conf. Robotics and Automa-
tion (ICRA), pages 3–14, Marina Bay Sands, Singa-
pore.
Voinea, G.-D., Girbacia, F., Postelnicu, C. C., and Marto, A.
(2018). Exploring cultural heritage using augmented
reality through Google’s Project Tango and ARCore.
In Proc. Intl. Conf. VR Techn. in Cultural Heritage,
pages 93–106, Brasov, Romania.
Yan, D. and Hu, H. (2017). Application of augmented real-
ity and robotic technology in broadcasting: A survey.
Intl. J. on Robotics, 6(3):18–27.
GRAPP 2020 - 15th International Conference on Computer Graphics Theory and Applications
318