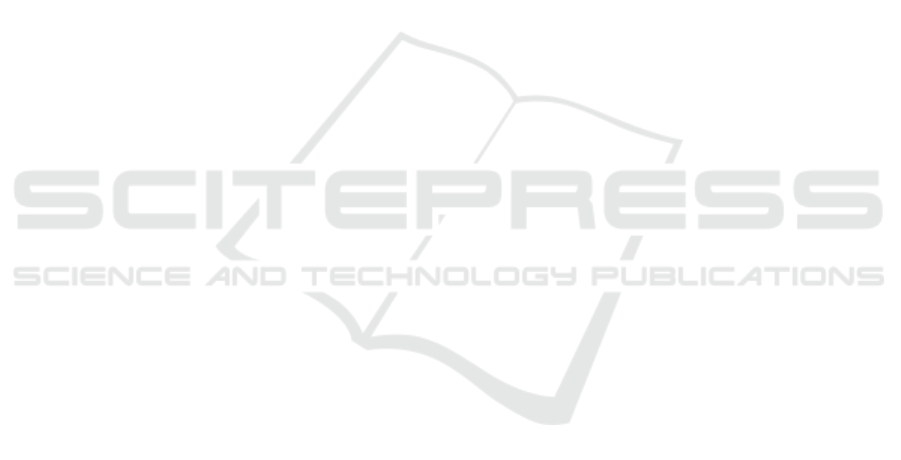
dimensional, representation (Hinton 2014). By sim-
plifying a very rich source of data, such as human mo-
tion, in which unimportant details are ignored, a set of
semiotics could possibly emerge conveying messages
through non-verbal means, such as gestures and body
expressions.
5.2 Multimodal Approach
The input data could be extended, beyond motion cap-
ture data to include other sensorial input, both direct
such as a music score or indirect such as using Elec-
troencephalography (EEG) or other bio-signals from
a participant on stage (Hieda, 2017). By exploring
this sensorial diversity new choreographic forms and
practices can emerge, redefining the role of the per-
former and their artistic relationships.
REFERENCES
Alemi, O. and Pasquier, P. (2019). Machine learning for
data-driven movement generation: a review of the
state of the art. CoRR, abs/1903.08356.
Billeskov, J. A., Møller, T. N., Triantafyllidis, G., and Pala-
mas, G. (2018). Using motion expressiveness and
human pose estimation for collaborative surveillance
art. In Interactivity, Game Creation, Design, Learn-
ing, and Innovation, pages 111–120. Springer.
Bishop, C. M. (1994). Mixture density networks. Technical
report, Aston University.
Crnkovic-Friis, L. and Crnkovic-Friis, L. (2016). Genera-
tive choreography using deep learning. arXiv preprint
arXiv:1605.06921.
Dorin, A., McCabe, J., McCormack, J., Monro, G., and
Whitelaw, M. (2012). A framework for understand-
ing generative art. Digital Creativity, 23:3–4.
Eckersall, P., Grehan, H., and Scheer, E. (2017). Cue
black shadow effect: The new media dramaturgy ex-
perience. In New Media Dramaturgy, pages 1–23.
Springer.
Feng, Q. (2019). Interactive performance and immersive
experience in dramaturgy-installation design for chi-
nese kunqu opera “the peony pavilion”. In The In-
ternational Conference on Computational Design and
Robotic Fabrication, pages 104–115. Springer.
Grba, D. (2017). Avoid setup: Insights and implications of
generative cinema. Technoetic Arts, 15(3):247–260.
Hieda, N. (2017). Mobile brain-computer interface for
dance and somatic practice. In Adjunct Publication of
the 30th Annual ACM Symposium on User Interface
Software and Technology, pages 25–26. ACM.
Hochreiter, S. (1998). The vanishing gradient problem dur-
ing learning recurrent neural nets and problem solu-
tions. International Journal of Uncertainty, Fuzziness
and Knowledge-Based Systems, 6(02):107–116.
Hochreiter, S. and Schmidhuber, J. (1997). Long short-term
memory. Neural computation, 9(8):1735–1780.
Kakoudaki, D. (2014). Anatomy of a robot: Literature, cin-
ema, and the cultural work of artificial people. Rut-
gers University Press.
Liou, C.-Y., Cheng, W.-C., Liou, J.-W., and Liou, D.-R.
(2014). Autoencoder for words. Neurocomputing,
139:84–96.
Lister, M., Giddings, S., Dovey, J., Grant, I., and Kelly, K.
(2008). New media: A critical introduction. Rout-
ledge.
Mokhov, S. A., Kaur, A., Talwar, M., Gudavalli, K., Song,
M., and Mudur, S. P. (2018). Real-time motion capture
for performing arts and stage. In ACM SIGGRAPH
2018 Educator’s Forum on - SIGGRAPH ’18, page
nil.
Pavllo, D., Feichtenhofer, C., Auli, M., and Grangier, D.
(2019). Modeling human motion with quaternion-
based neural networks. International Journal of Com-
puter Vision.
Schedel, M. and Rootberg, A. (2009). Generative tech-
niques in hypermedia performance. Contemporary
Music Review, 28(1):57–73.
Seo, J. H. and Bergeron, C. (2017). Art and technology col-
laboration in interactive dance performance. Teaching
Computational Creativity, page 142.
Shu, Z., Sahasrabudhe, M., Alp Guler, R., Samaras, D.,
Paragios, N., and Kokkinos, I. (2018). Deforming au-
toencoders: Unsupervised disentangling of shape and
appearance. In Proceedings of the European Confer-
ence on Computer Vision (ECCV), pages 650–665.
Socha, B. and Eber-Schmid, B. (2014). What is new me-
dia. Retrieved from New Media Institute http://www.
newmedia. org/what-is-new-media. html.
Villegas, R., Yang, J., Ceylan, D., and Lee, H. (2018). Neu-
ral kinematic networks for unsupervised motion retar-
getting. In Proc. IEEE/CVF Conference on Computer
Vision and Pattern Recognition.
Webb, A. M., Wang, C., Kerne, A., and Cesar, P. (2016).
Distributed liveness: understanding how new tech-
nologies transform performance experiences. In Pro-
ceedings of the 19th ACM Conference on Computer-
Supported Cooperative Work & Social Computing,
pages 432–437. ACM.
GRAPP 2020 - 15th International Conference on Computer Graphics Theory and Applications
326