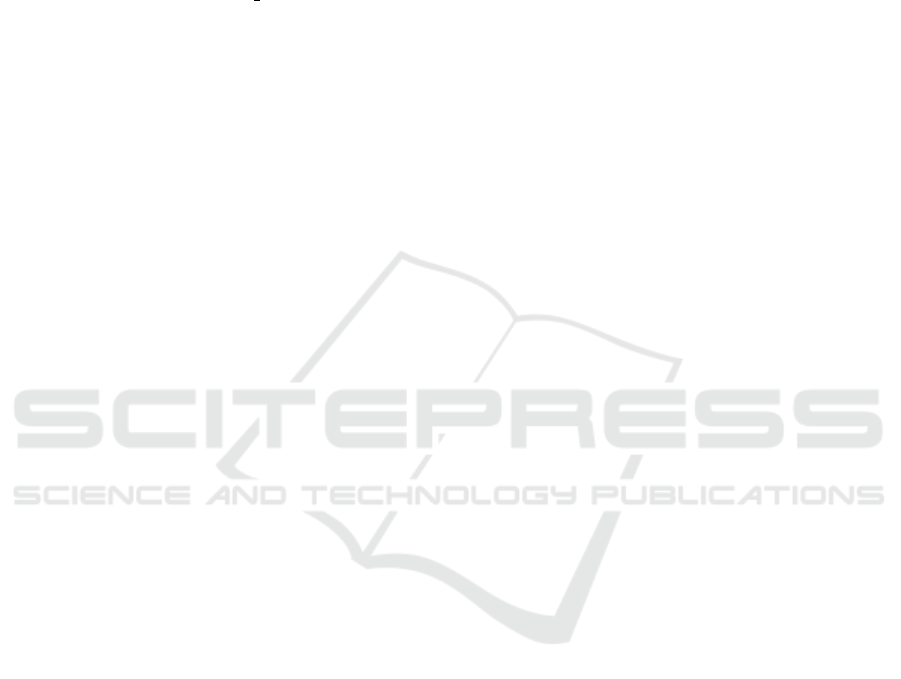
REFERENCES
Aronson, A. R. (2001). Effective mapping of biomedical text
to the umls metathesaurus: The metamap program.
Aronson, A. R. (2006). Metamap: Mapping text to the umls
metathesaurus. Bethesda, MD: NLM, NIH, DHHS,
pages 1–26.
Bodenreider, O. (2004). The unified medical language sys-
tem (umls): integrating biomedical terminology. Nu-
cleic acids research, 32(suppl 1):D267–D270.
Cestnik, B. et al. (1990). Estimating probabilities: a crucial
task in machine learning. In ECAI, volume 90, pages
147–149.
Collier, N., Oellrich, A., and Groza, T. (2015). Concept se-
lection for phenotypes and diseases using learn to rank.
Journal of biomedical semantics, 6(1):24.
Devlin, J., Chang, M.-W., Lee, K., and Toutanova, K.
(2018). Bert: Pre-training of deep bidirectional trans-
formers for language understanding. arXiv preprint
arXiv:1810.04805.
Donnelly, K. (2006). Snomed-ct: The advanced terminol-
ogy and coding system for ehealth. Studies in health
technology and informatics, 121:279.
(EFSA), E. F. S. A. (2015). The food classification and de-
scription system foodex 2 (revision 2). EFSA Support-
ing Publications, 12(5):804E.
Eftimov, T., Ispirova, G., Finglas, P., Korosec, P., and
Korousic-Seljak, B. (2018). Quisper ontology learn-
ing from personalized dietary web services. In KEOD,
pages 277–284.
Eftimov, T., Koro
ˇ
sec, P., and Korou
ˇ
si
´
c Seljak, B. (2017).
Standfood: standardization of foods using a semi-
automatic system for classifying and describing foods
according to foodex2. Nutrients, 9(6):542.
Eftimov, T. and Seljak, B. K. (2015). Pos tagging-
probability weighted method for matching the internet
recipe ingredients with food composition data. In 2015
7th International Joint Conference on Knowledge Dis-
covery, Knowledge Engineering and Knowledge Man-
agement (IC3K), volume 1, pages 330–336. IEEE.
Grcar, M., Krek, S., and Dobrovoljc, K. (2012). Obeliks:
statisticni oblikoskladenjski oznacevalnik in lematiza-
tor za slovenski jezik. In Zbornik Osme konference
Jezikovne tehnologije, Ljubljana, Slovenia.
Griffiths, E. J., Dooley, D. M., Buttigieg, P. L., Hoehn-
dorf, R., Brinkman, F. S., and Hsiao, W. W. (2016).
Foodon: A global farm-to-fork food ontology. In
ICBO/BioCreative.
Ispirova, G., Eftimov, T., Korousic-Seljak, B., and Korosec,
P. (2017). Mapping food composition data from var-
ious data sources to a domain-specific ontology. In
KEOD, pages 203–210.
Korenius, T., Laurikkala, J., J
¨
arvelin, K., and Juhola, M.
(2004). Stemming and lemmatization in the clustering
of finnish text documents. In Proceedings of the thir-
teenth ACM international conference on Information
and knowledge management, pages 625–633. ACM.
Kosub, S. (2019). A note on the triangle inequality for the
jaccard distance. Pattern Recognition Letters, 120:36–
38.
Lan, Z., Chen, M., Goodman, S., Gimpel, K., Sharma, P.,
and Soricut, R. (2019). Albert: A lite bert for self-
supervised learning of language representations. arXiv
preprint arXiv:1909.11942.
Lartey, A. (2015). End hunger, achieve food security and im-
proved nutrition and promote sustainable agriculture.
UN Chronicle, 51(4):6–8.
Liu, Y., Ott, M., Goyal, N., Du, J., Joshi, M., Chen, D.,
Levy, O., Lewis, M., Zettlemoyer, L., and Stoyanov, V.
(2019). Roberta: A robustly optimized bert pretraining
approach. arXiv preprint arXiv:1907.11692.
Lu, Z., Kao, H.-Y., Wei, C.-H., Huang, M., Liu, J., Kuo, C.-
J., Hsu, C.-N., Tsai, R. T.-H., Dai, H.-J., Okazaki, N.,
et al. (2011). The gene normalization task in biocre-
ative iii. BMC bioinformatics, 12(8):S2.
M
`
arquez, L. and Rodr
´
ıguez, H. (1998). Part-of-speech tag-
ging using decision trees. In European Conference on
Machine Learning, pages 25–36. Springer.
Metzler, D., Dumais, S., and Meek, C. (2007). Similar-
ity measures for short segments of text. In Euro-
pean conference on information retrieval, pages 16–
27. Springer.
Mikolov, T., Chen, K., Corrado, G., and Dean, J. (2013a).
Efficient estimation of word representations in vector
space. arXiv preprint arXiv:1301.3781.
Mikolov, T., Sutskever, I., Chen, K., Corrado, G. S., and
Dean, J. (2013b). Distributed representations of words
and phrases and their compositionality. In Advances
in neural information processing systems, pages 3111–
3119.
Morgan, A. A., Lu, Z., Wang, X., Cohen, A. M., Fluck, J.,
Ruch, P., Divoli, A., Fundel, K., Leaman, R., Haken-
berg, J., et al. (2008). Overview of biocreative ii gene
normalization. Genome biology, 9(2):S3.
Pennington, J., Socher, R., and Manning, C. (2014). Glove:
Global vectors for word representation. In Proceedings
of the 2014 conference on empirical methods in natu-
ral language processing (EMNLP), pages 1532–1543.
Popovski, G., Korou
ˇ
si
´
c Seljak, B., and Eftimov, T. (2019).
Foodontomap: Linking food concepts across differ-
ent food ontologies. In Proceedings of the 11th In-
ternational Joint Conference on Knowledge Discov-
ery, Knowledge Engineering and Knowledge Manage-
ment - Volume 2: KEOD,, pages 195–202. INSTICC,
SciTePress.
Pramanik, S. and Hussain, A. (2019). Text normalization us-
ing memory augmented neural networks. Speech Com-
munication, 109:15–23.
Savova, G. K., Masanz, J. J., Ogren, P. V., Zheng, J., Sohn,
S., Kipper-Schuler, K. C., and Chute, C. G. (2010).
Mayo clinical text analysis and knowledge extraction
system (ctakes): architecture, component evaluation
and applications. Journal of the American Medical In-
formatics Association, 17(5):507–513.
Schuyler, P. L., Hole, W. T., Tuttle, M. S., and Sherertz,
D. D. (1993). The umls metathesaurus: representing
different views of biomedical concepts. Bulletin of the
Medical Library Association, 81(2):217.
Voutilainen, A. (2003). Part-of-speech tagging. The Oxford
handbook of computational linguistics, pages 219–
232.
Yang, Z., Dai, Z., Yang, Y., Carbonell, J., Salakhutdinov,
R., and Le, Q. V. (2019). Xlnet: Generalized autore-
gressive pretraining for language understanding. arXiv
preprint arXiv:1906.08237.
HEALTHINF 2020 - 13th International Conference on Health Informatics
216