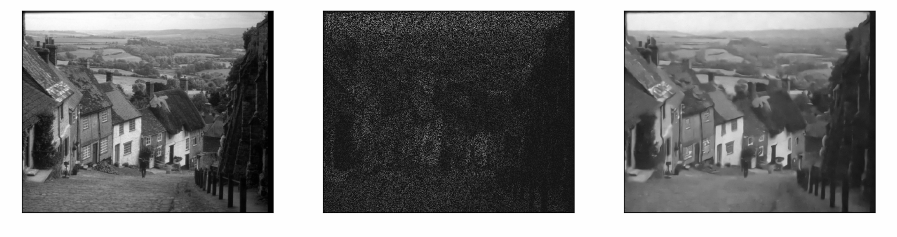
Figure 7: Image inpainting example. Left: Original image, Middle: Degraded image with 80% of the pixels set to zero,
Right: Inpainted image using our method.
REFERENCES
Ahmad, R., Bouman, C. A., Buzzard, G. T., Chan, S.,
Reehorst, E. T., and Schniter, P. (2019). Plug and
play methods for magnetic resonance imaging. arXiv
preprint arXiv:1903.08616.
Bigdeli, S. A. and Zwicker, M. (2017). Image restora-
tion using autoencoding priors. arXiv preprint
arXiv:1703.09964.
Bigdeli, S. A., Zwicker, M., Favaro, P., and Jin, M. (2017).
Deep mean-shift priors for image restoration. In
Advances in Neural Information Processing Systems,
pages 763–772.
Chang, J.-H. R., Li, C.-L., Poczos, B., Kumar, B. V., and
Sankaranarayanan, A. C. (2017). One network to
solve them all-solving linear inverse problems using
deep projection models. In ICCV, pages 5889–5898.
Chen, Y. and Pock, T. (2016). Trainable nonlinear reaction
diffusion: A flexible framework for fast and effective
image restoration. IEEE transactions on pattern anal-
ysis and machine intelligence, 39(6):1256–1272.
Heide, F., Steinberger, M., Tsai, Y.-T., Rouf, M., Paj ˛ak, D.,
Reddy, D., Gallo, O., Liu, J., Heidrich, W., Egiazar-
ian, K., et al. (2014). Flexisp: A flexible camera image
processing framework. ACM Transactions on Graph-
ics (TOG), 33(6):231.
Isola, P., Zhu, J.-Y., Zhou, T., and Efros, A. A. (2017).
Image-to-image translation with conditional adversar-
ial networks. In Proceedings of the IEEE conference
on computer vision and pattern recognition, pages
1125–1134.
Jin, M., Roth, S., and Favaro, P. (2017). Noise-blind image
deblurring. In Proceedings of the IEEE Conference
on Computer Vision and Pattern Recognition, pages
3510–3518.
Krishnan, D. and Fergus, R. (2009). Fast image deconvo-
lution using hyper-laplacian priors. In Advances in
neural information processing systems, pages 1033–
1041.
Martin, D., Fowlkes, C., Tal, D., and Malik, J. (2001).
A database of human segmented natural images and
its application to evaluating segmentation algorithms
and measuring ecological statistics. In Proc. 8th Int’l
Conf. Computer Vision, volume 2, pages 416–423.
Reehorst, E. T. and Schniter, P. (2018). Regulariza-
tion by denoising: Clarifications and new interpreta-
tions. IEEE Transactions on Computational Imaging,
5(1):52–67.
Schelten, K., Nowozin, S., Jancsary, J., Rother, C., and
Roth, S. (2015). Interleaved regression tree field cas-
cades for blind image deconvolution. In 2015 IEEE
Winter Conference on Applications of Computer Vi-
sion, pages 494–501. IEEE.
Schmidt, U. and Roth, S. (2014). Shrinkage fields for ef-
fective image restoration. In Proceedings of the IEEE
Conference on Computer Vision and Pattern Recogni-
tion, pages 2774–2781.
Sønderby, C. K., Caballero, J., Theis, L., Shi, W., and
Huszár, F. (2016). Amortised map inference for image
super-resolution. arXiv preprint arXiv:1610.04490.
Sun, L., Cho, S., Wang, J., and Hays, J. (2013). Edge-based
blur kernel estimation using patch priors. In IEEE In-
ternational Conference on Computational Photogra-
phy (ICCP), pages 1–8. IEEE.
Tirer, T. and Giryes, R. (2018). Image restoration by itera-
tive denoising and backward projections. IEEE Trans-
actions on Image Processing, 28(3):1220–1234.
Ulyanov, D., Vedaldi, A., and Lempitsky, V. (2018). Deep
image prior. In Proceedings of the IEEE Conference
on Computer Vision and Pattern Recognition, pages
9446–9454.
Venkatakrishnan, S. V., Bouman, C. A., and Wohlberg, B.
(2013). Plug-and-play priors for model based recon-
struction. In 2013 IEEE Global Conference on Signal
and Information Processing, pages 945–948. IEEE.
Zhang, C., Bengio, S., Hardt, M., Recht, B., and Vinyals, O.
(2016). Understanding deep learning requires rethink-
ing generalization. arXiv preprint arXiv:1611.03530.
Zhang, K., Zuo, W., Chen, Y., Meng, D., and Zhang, L.
(2017a). Beyond a gaussian denoiser: Residual learn-
ing of deep cnn for image denoising. IEEE Transac-
tions on Image Processing, 26(7):3142–3155.
Zhang, K., Zuo, W., Gu, S., and Zhang, L. (2017b). Learn-
ing deep cnn denoiser prior for image restoration. In
Proceedings of the IEEE conference on computer vi-
sion and pattern recognition, pages 3929–3938.
Zoran, D. and Weiss, Y. (2011). From learning models of
natural image patches to whole image restoration. In
2011 International Conference on Computer Vision,
pages 479–486. IEEE.