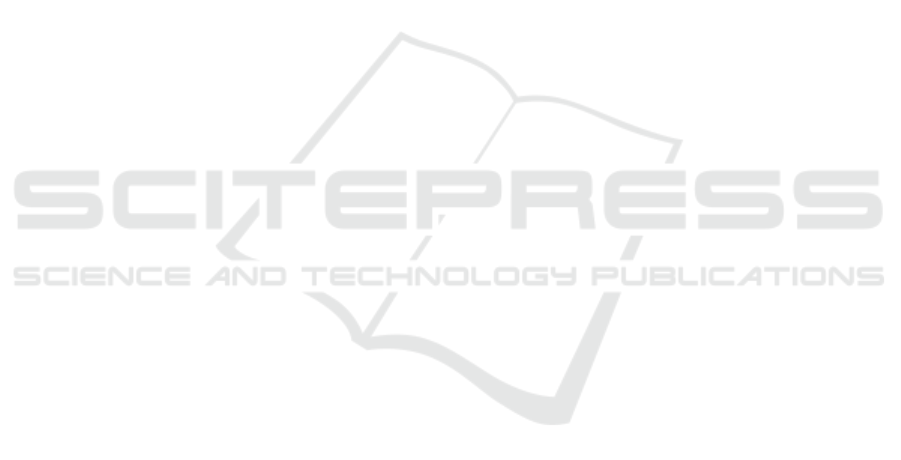
possible to verify the generalization capacity. The
classification model evaluated in this work was shown
to be efficient, obtaining an average of 94.35 % ac-
curacy. Being able to reach a high accuracy, even
with the unbalanced dataset and with the iterations
obtained in the cross validation being proxies.
ACKNOWLEDGMENTS
The elaboration of this work would not have been
possible without the collaboration of the Engineering
and DecisionSupport Research Center (GECAD) of
the Institute of Engineering, Polytechnic Institute of
Porto, Portugal and FAPEMA.
REFERENCES
Alten, F., Lauermann, J., Clemens, C., Heiduschka, P.,
and Eter, N. (2017). Signal reduction in chorio-
capillaris and segmentation errors in spectral domain
oct angiography caused by soft drusen. Graefe’s
Archive for Clinical and Experimental Ophthalmol-
ogy, 255(12):2347–2355.
Baxter, S. L., Pistilli, M., Pujari, S. S., Liesegang, T. L.,
Suhler, E. B., Thorne, J. E., Foster, C. S., Jabs,
D. A., Levy-Clarke, G. A., Nussenblatt, R. B., et al.
(2013). Risk of choroidal neovascularization among
the uveitides. American journal of ophthalmology,
156(3):468–477.
Bressler, S. B., Glassman, A. R., Almukhtar, T., Bressler,
N. M., Ferris, F. L., Googe Jr, J. M., Gupta, S. K., Jam-
pol, L. M., Melia, M., Wells III, J. A., et al. (2016).
Five-year outcomes of ranibizumab with prompt or
deferred laser versus laser or triamcinolone plus de-
ferred ranibizumab for diabetic macular edema. Amer-
ican journal of ophthalmology, 164:57–68.
Chen, Y.-H., Krishna, T., Emer, J. S., and Sze, V. (2017).
Eyeriss: An energy-efficient reconfigurable acceler-
ator for deep convolutional neural networks. IEEE
Journal of Solid-State Circuits, 52(1):127–138.
Dimitrova, G., Chihara, E., Takahashi, H., Amano, H., and
Okazaki, K. (2017). Quantitative retinal optical co-
herence tomography angiography in patients with dia-
betes without diabetic retinopathy. Investigative oph-
thalmology & visual science, 58(1):190–196.
Esteva, A., Kuprel, B., Novoa, R. A., Ko, J., Swetter, S. M.,
Blau, H. M., and Thrun, S. (2017). Dermatologist-
level classification of skin cancer with deep neural net-
works. Nature, 542(7639):115.
Gaier, E. D., Rizzo III, J. F., Miller, J. B., and Ces-
tari, D. M. (2017). Focal capillary dropout asso-
ciated with optic disc drusen using optical coher-
ence tomographic angiography. Journal of Neuro-
ophthalmology, 37(4):405–410.
Gill, A., Cole, E. D., Novais, E. A., Louzada, R. N., Carlo,
T., Duker, J. S., Waheed, N. K., Baumal, C. R., and
Witkin, A. J. (2017). Visualization of changes in the
foveal avascular zone in both observed and treated di-
abetic macular edema using optical coherence tomog-
raphy angiography. International journal of retina and
vitreous, 3(1):19.
Grimm, K. J., Mazza, G. L., and Davoudzadeh, P. (2017).
Model selection in finite mixture models: A k-fold
cross-validation approach. Structural Equation Mod-
eling: A Multidisciplinary Journal, 24(2):246–256.
Havaei, M., Davy, A., Warde-Farley, D., Biard, A.,
Courville, A., Bengio, Y., Pal, C., Jodoin, P.-M., and
Larochelle, H. (2017). Brain tumor segmentation
with deep neural networks. Medical image analysis,
35:18–31.
Kermany, D., Zhang, K., and Goldbaum, M. (2018). La-
beled optical coherence tomography (oct) and chest
x-ray images for classification. Structural Equation
Modeling: A Multidisciplinary Journal.
Kov
´
acs, G., T
´
oth, L., Van Compernolle, D., and Ganapathy,
S. (2017). Increasing the robustness of cnn acoustic
models using autoregressive moving average spectro-
gram features and channel dropout. Pattern Recogni-
tion Letters, 100:44–50.
Maggiori, E., Tarabalka, Y., Charpiat, G., and Alliez, P.
(2017). Convolutional neural networks for large-scale
remote-sensing image classification. IEEE Transac-
tions on Geoscience and Remote Sensing, 55(2):645–
657.
Parhi, K. K., Reinsbach, M., Koozekanani, D. D., and Roy-
chowdhury, S. (2017). Automated oct segmentation
for images with dme. In Medical Image Analysis and
Informatics, pages 115–132. CRC Press.
Preti, R. C., Govetto, A., Aqueta Filho, R. G., Zacharias,
L. C., Pimentel, S. G., Takahashi, W. Y., Monteiro,
M. L., Hubschman, J. P., Sarraf, D., et al. (2018). Op-
tical coherence tomography analysis of outer retinal
tubulations: sequential evolution and pathophysiolog-
ical insights. Retina, 38(8):1518–1525.
Roy, R., Saurabh, K., Bansal, A., Kumar, A., Majum-
dar, A. K., and Paul, S. S. (2017). Inflammatory
choroidal neovascularization in indian eyes: Etiology,
clinical features, and outcomes to anti-vascular en-
dothelial growth factor. Indian journal of ophthalmol-
ogy, 65(4):295.
Salamon, J. and Bello, J. P. (2017). Deep convolutional neu-
ral networks and data augmentation for environmental
sound classification. IEEE Signal Processing Letters ,
24(3):279–283.
Saraiva, A., Melo, R., Filipe, V., Sousa, J., Ferreira, N. F.,
and Valente, A. (2018). Mobile multirobot manipula-
tion by image recognition.
Saraiva., A. A., Ferreira., N. M. F., de Sousa., L. L., Costa.,
N. J. C., Sousa., J. V. M., Santos., D. B. S., Valente.,
A., and Soares., S. (2019a). Classification of images
of childhood pneumonia using convolutional neural
networks. In Proceedings of the 12th International
Joint Conference on Biomedical Engineering Systems
and Technologies - Volume 2: BIOIMAGING,, pages
112–119. INSTICC, SciTePress.
Saraiva., A. A., Santos., D. B. S., Costa., N. J. C., Sousa.,
J. V. M., Ferreira., N. M. F., Valente., A., and Soares.,
BIOINFORMATICS 2020 - 11th International Conference on Bioinformatics Models, Methods and Algorithms
174