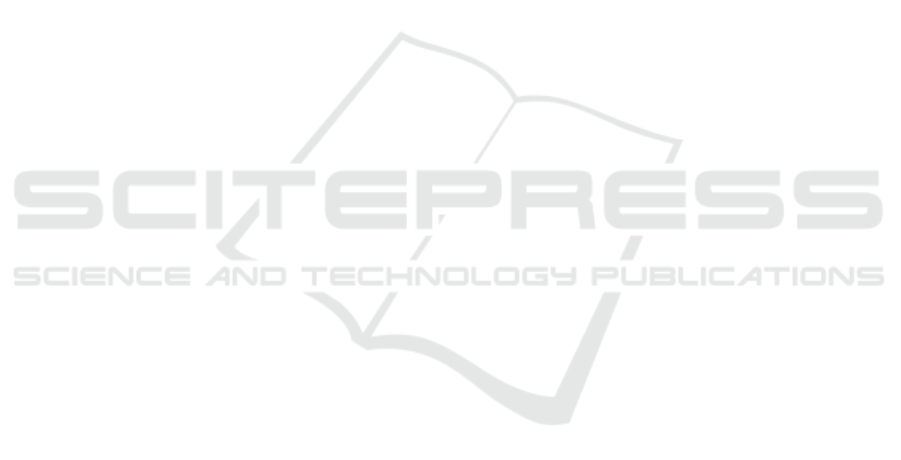
Ebisawa, Y. and Sugiura, M. (1998). Influences of target
and fixation point conditions on characteristics of vi-
sually guided voluntary saccade. The Journal of the
Institute of Image Information and Television Engi-
neers, 52(11):1730–1737.
Engbert, R. (2006). Microsaccades: a microcosm for re-
search on oculomotor control, attention, and visual
perception. Progress in Brain Research, 154:177–192.
Engbert, R. and Kliegl, R. (2003). Microsaccades uncover
the orientation of covert attention. Vision Research,
43:1035–1045.
Engbert, R., Sinn, P., Mergenthaler, K., and Truken-
brod, H. (2015). Microsaccade toolbox 0.9.
http://read.psych.uni-potsdam.de.
Furuta, T., Ishikawa, H., Toyama, N., Suzuki, H., and
Sakamoto, T. (1993). Effects of instruction in de-
vice operation learning. IEICE Transactions, J76–D–
II(9):2031–2041.
Kohama, T., Nakai, Y., Ohtani, S., Yamamoto, M., Ueda,
S., and Yoshida, H. (2017). Quantitative compari-
son of cognitive load derived from voice or manual
responses based on microsaccade rate analysis. The
Transactions of Human Interface Society, 19(2):189–
197.
Krejtz, K., Duchowski, A. T., Niedzielska, A., Biele, C.,
and Krejtz, I. (2018). Eye tracking cognitive load us-
ing pupil diameter and microsaccades with fixed gaze.
PloS One, 13:1–23.
Meyberg, S., Sinn, P., Engbert, R., and Sommer, W. (2017).
Revising the link between microsaccades and the spa-
tial cueing of voluntary attention. Vision Research,
133:47–60.
Mizushina, H., Sakamoto, K., and Kaneko, H. (2011).
The relationship between psychlogical stress induced
by task workload and dynamic characteristics of sac-
cadic eye movements. IEICE Transactions, J94–
D(10):1640–1651.
Nakayama, M. and Hayakawa, Y. (2019). Relationships
between oculo-motor measures as task-evoked mental
workloads during a manupulation task. In 23rd Inter-
national Conference Information Visualisation (IV),
pages 170–174.
Nakayama, M. and Katsukura, M. (2011). Development of
a system usability assessment procedure using oculo-
motors for input operation. Universal Access in Infor-
mation Society, 10(1):51–68.
Nakayama, M. and Shimizu, Y. (2004). Frequency analysis
of task evoked pupillary response and eye-movement.
In Spencer, S. N., editor, Eye-Tracking Research and
Applications Symposium 2002, pages 71–76, New
York, USA. ACM, ACM Press.
Peiß, S., Wickens, C. D., and Baruah, R. (2018). Eye-
tracking measures in aviation: A selective literature
review. The International Journal of Aerospace Psy-
chology, 28(Issue 3–4):98–112.
Toyoda, H. (2007). KYO BUNSAN KOUZOU BUNSEKI
[AMOS HEN]. Tokyo Syoseki, Tokyo, Japan.
Ziv, G. (2016). Gaze behavior and visual attention: A re-
view of eye tracking studies in aviation. The Inter-
national Journal of Aviation Psychology, 26(3–4):75–
104.
Impact of Task-evoked Mental Workloads on Oculo-motor Indices during a Manipulation Task
279