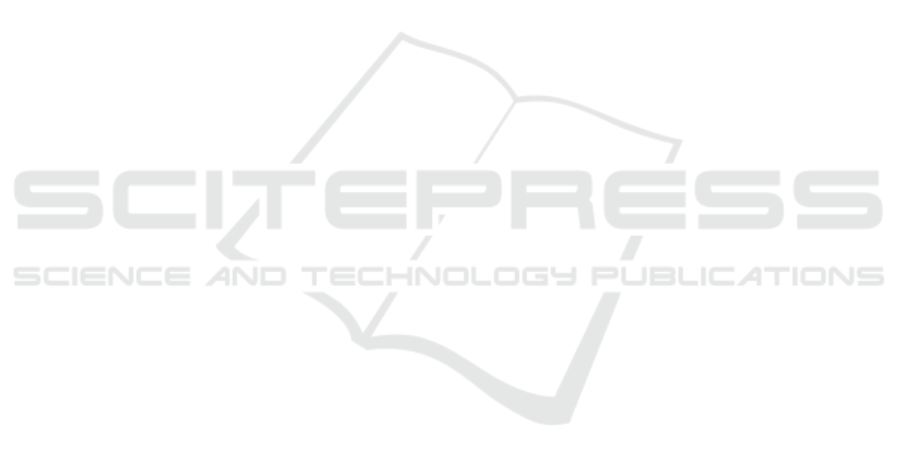
essarily that the entire regions of person body and ob-
ject are visible). As can be seen from scenes (c) and
(d) in Figure 7, when an object is hold by one per-
son’s hand after another, the proposed method detects
such human-object interaction individually and cor-
rectly (frames 180, 250 of scene (c) and frames 143,
195 of scene (d)).
These experiment results shows the effectiveness
of the proposed method in detecting human-object in-
teraction for diverse situations.
5 CONCLUSIONS
In this paper, we have focused on the type of human-
object interaction where a person is in the middle of
moving an object with his/her hand, and proposed a
novel method for detecting such type of human-object
interaction by the motion distribution in an individual
area surrounding each hand. Since our method needs
not explicitly extract object regions from input im-
ages and recognize their correspondence to person re-
gions, the effectiveness in detecting the human-object
interaction is expected to be improved for diverse sit-
uations. Through the experiments on human activity
video images, we confirmed the effectiveness of our
proposed method in the situations where a person is
right in the middle of moving a relatively large object
roughly parallel to the image plane.
We will conduct further experiments on a variety
of environments such as the different angles of cam-
eras, the various types of objects, the different num-
bers of persons, and the diverse conditions of occlu-
sion areas. Currently, our proposed method achieves
several decision processes as thresholding procedures
by Eqs. (1), (9), and (10). We would like to investigate
approaches for achieving these processes as machine
learning based procedures.
In future work, we plan to extend our proposed
method to multiple camera environment. This is be-
cause, we can expect to deal with the decrease in in-
teraction detection accuracy from unsuitable image
condition by the following approach: several images
of the same person are taken from different angles,
unsuitable condition images, where his/her hand is
hard to detect, overlaps considerably with other body
part regions, or moves roughly perpendicular to the
image plane, are excluded from the taken images, and
human-object interaction is detected by using the re-
maining images.
REFERENCES
Cao, Z., Simon, T., Wei, S.-E., and Sheikh, Y. (2017).
Realtime multi-person 2D pose estimation using part
affinity fields. In IEEE Conf. Comput. Vision Pattern
Recognit., pages 1302–1310.
Chao, Y.-W., Wang, Z., He, Y., Wang, J., and Deng, J.
(2015). HICO: A benchmark for recognizing human-
object interactions in images. In Int. Conf. Comput.
Vision, pages 1017–1025.
Drillis, R., Contini, R., and Bluestein, M. (1964). Body
segment parameters: A survey of measurement tech-
niques. Artif. Limbs, 8(1):44–66.
Ghuge, N. and Dhulekar, P. (2017). Abandoned object
detection. Int. J. Mod. Trends Sci.ence Technol.,
3(6):215–218.
Koppula, H. S., Gupta, R., and Saxena, A. (2013). Learn-
ing human activities and object affordances from rgb-
d videos. Int. J. Rob. Res., 32(8):951–970.
Kroeger, T., Timofte, R., Dai, D., and Gool, L. V. (2016).
Fast optical flow using dense inverse search. In Eur.
Conf. Comput. Vision, pages 471–488.
Le, D.-T., Uijlings, J., and Bernardi, R. (2014). TUHOI:
Trento universal human object interaction dataset. In
The 3rd Workshop Vision Lang., pages 17–24.
Leo, M., Mosca, N., Spagnolo, P., Mazzeo, P. L., D’Orazio,
T., and Distante, A. (2008). Real-time multiview anal-
ysis of soccer matches for understanding interactions
between ball and players. In Int. Conf. Content-Based
Image Video Retrieval, pages 525–534.
Liciotti, D., Contigiani, M., Frontoni, E., Mancini, A., Zin-
garetti, P., and Placidi, V. (2014). Shopper analytics:
A customer activity recognition system using a dis-
tributed rgb-d camera network. In Int. Workshop Video
Anal. Audience Meas. Retail Digital Signage, pages
146–157.
Lin, K., Chen, S.-C., Chen, C.-S., Lin, D.-T., and Hung,
Y.-P. (2015). Abandoned object detection via tempo-
ral consistency modeling and back-tracing verification
for visual surveillance. IEEE Trans. Inf. Forensics Se-
curity, 10(7):1359–1370.
Meng, M., Drira, H., and Boonaert, J. (2018). Distances
evolution analysis for online and off-line human ob-
ject interaction recognition. Image Vision Comput.,
70:32–45.
Mitsuhashi, Y., Abe, T., and Suganuma, T. (2014). A detec-
tion method of human-object interactions in crowded
environment based on hierarchical image analysis.
Tech. Rep. IEICE, 114(PRMU2014-77):69–74.
Roy, D. and Chalavadi, K. M. (2018). Snatch theft detec-
tion in unconstrained surveillance videos using action
attribute modelling. Pattern Recognit. Lett., 108:56–
61.
Shih, H.-C. (2018). A survey of content-aware video anal-
ysis for sports. IEEE Trans. Circuits Syst. Video Tech-
nol., 28(5):1212–1231.
Ubalde, S., Liu, Z., and Mejail, M. (2014). Detecting sub-
tle human-object interactions using Kinect. In 19th
Iberoam. Congr. Pattern Recognit., pages 770–777.
Zhang, H.-B., Zhang, Y.-X., Zhong, B., Lei, Q., Yang, L.,
Du, J.-X., and Chen, D.-S. (2019). A comprehen-
sive survey of vision-based human action recognition
methods. Sensors, 19(5):1005.
A Method for Detecting Human-object Interaction based on Motion Distribution around Hand
469