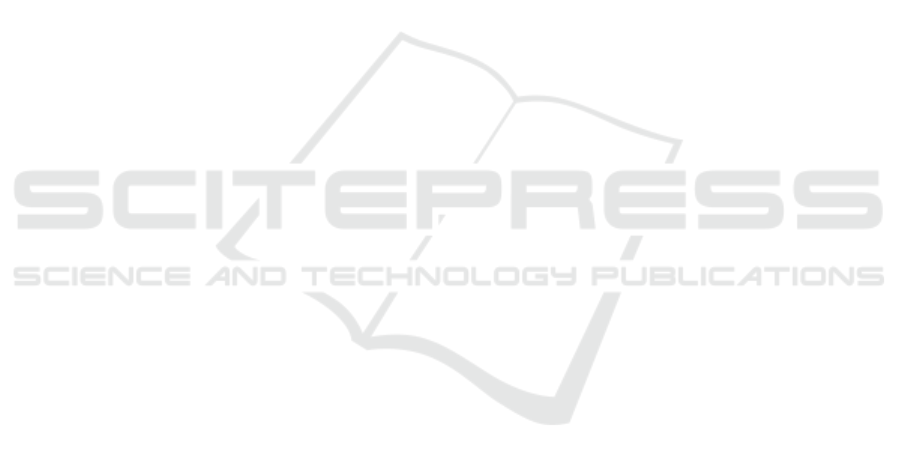
Harari, G. M., M
¨
uller, S. R., Aung, M. S., and Rentfrow,
P. J. (2017). Smartphone sensing methods for study-
ing behavior in everyday life. Current opinion in be-
havioral sciences, 18:83–90.
Hinshaw, S. P. and Stier, A. (2008). Stigma as related to
mental disorders. Annu. Rev. Clin. Psychol., 4:367–
393.
Jang, H.-J. and Kwag, Y.-K. (2015). Comparison of factors
associated with smartphone over-usage: Focusing on
self-control, mental health and interrelationship. Jour-
nal of the Korea Academia-Industrial cooperation So-
ciety, 16(1):146–154.
Jedlitschka, A. and Pfahl, D. (2005). Reporting guidelines
for controlled experiments in software engineering. In
International Symposium on Empirical Software En-
gineering, 2005., pages 10–pp. IEEE.
Kazdin, A. E. (2019). Annual research review: Expand-
ing mental health services through novel models of in-
tervention delivery. Journal of Child Psychology and
Psychiatry, 60(4):455–472.
Keele, S. (2007). Guidelines for performing systematic lit-
erature reviews in software engineering. Technical re-
port, Technical report, Ver. 2.3 EBSE Technical Re-
port. EBSE.
King, W. C., Kalarchian, M. A., Steffen, K. J., Wolfe, B. M.,
Elder, K. A., and Mitchell, J. E. (2013). Associations
between physical activity and mental health among
bariatric surgical candidates. Journal of psychoso-
matic research, 74(2):161–169.
Larson, G. E., Booth-Kewley, S., Merrill, L. L., and
Stander, V. A. (2001). Physical symptoms as indi-
cators of depression and anxiety. Military medicine,
166(9):796–799.
Lee, Y.-K., Chang, C.-T., Lin, Y., and Cheng, Z.-H. (2014).
The dark side of smartphone usage: Psychological
traits, compulsive behavior and technostress. Com-
puters in human behavior, 31:373–383.
McGinnis, R. S., McGinnis, E. W., Hruschak, J., Lopez-
Duran, N. L., Fitzgerald, K., Rosenblum, K. L., and
Muzik, M. (2018). Wearable sensors and machine
learning diagnose anxiety and depression in young
children. In IEEE EMBS International Conference on
Biomedical & Health Informatics (BHI), pages 410–
413. IEEE.
Mohr, D. C., Zhang, M., and Schueller, S. M. (2017).
Personal sensing: understanding mental health using
ubiquitous sensors and machine learning. Annual re-
view of clinical psychology, 13:23–47.
Mukhopadhyay, S. C. (2014). Wearable sensors for human
activity monitoring: A review. IEEE sensors journal,
15(3):1321–1330.
Ormel, J., VonKorff, M., Ustun, T. B., Pini, S., Korten, A.,
and Oldehinkel, T. (1994). Common mental disorders
and disability across cultures: results from the who
collaborative study on psychological problems in gen-
eral health care. JAMA, 272(22):1741–1748.
Palmius, N., Osipov, M., Bilderbeck, A., Goodwin, G.,
Saunders, K., Tsanas, A., and Clifford, G. (2014). A
multi-sensor monitoring system for objective mental
health management in resource constrained environ-
ments. In Appropriate Healthcare Technologies for
Low Resource Settings (AHT). IET.
Sahu, S. and Sharma, A. (2016). Detecting brainwaves to
evaluate mental health using labview and applications.
In International Conference on Emerging Technologi-
cal Trends (ICETT), pages 1–4. IEEE.
Sandulescu, V., Andrews, S., Ellis, D., Bellotto, N., and
Mozos, O. M. (2015). Stress detection using wear-
able physiological sensors. In International Work-
Conference on the Interplay Between Natural and Ar-
tificial Computation, pages 526–532. Springer.
Sano, A., Phillips, A. J., Amy, Z. Y., McHill, A. W., Tay-
lor, S., Jaques, N., Czeisler, C. A., Klerman, E. B.,
and Picard, R. W. (2015). Recognizing academic
performance, sleep quality, stress level, and mental
health using personality traits, wearable sensors and
mobile phones. In IEEE 12th International Confer-
ence on Wearable and Implantable Body Sensor Net-
works (BSN), pages 1–6. IEEE.
Sano, A. and Picard, R. W. (2013). Stress recognition us-
ing wearable sensors and mobile phones. In Humaine
Association Conference on Affective Computing and
Intelligent Interaction, pages 671–676. IEEE.
Stafford, M., Chandola, T., and Marmot, M. (2007). As-
sociation between fear of crime and mental health
and physical functioning. American journal of pub-
lic health, 97(11):2076–2081.
Stone, L. and Waldron, R. (2019). Great expectations and e-
mental health: The role of literacy in mediating access
to mental healthcare. Aust J Gen Pract, 48(7):474–79.
Str
¨
ohle, A., Hoefler, M., Pfister, H., Mueller, A.-G., Hoyer,
J., Wittchen, H.-U., and Lieb, R. (2007). Physical ac-
tivity and prevalence and incidence of mental disor-
ders in adolescents and young adults. Psychological
medicine, 37(11):1657–1666.
Sun, F.-T., Kuo, C., Cheng, H.-T., Buthpitiya, S., Collins, P.,
and Griss, M. (2010). Activity-aware mental stress de-
tection using physiological sensors. In International
conference on Mobile computing, applications, and
services, pages 282–301. Springer.
Wijsman, J., Grundlehner, B., Liu, H., Hermens, H., and
Penders, J. (2011). Towards mental stress detec-
tion using wearable physiological sensors. In An-
nual International Conference of the IEEE Engineer-
ing in Medicine and Biology Society, pages 1798–
1801. IEEE.
Yamaguchi, A., Ogawa, M., Tamura, T., and Togawa, T.
(1998). Monitoring behavior in the home using posi-
tioning sensors. In 20th Annual International Confer-
ence of the IEEE Engineering in Medicine and Biol-
ogy Society. Vol. 20 Biomedical Engineering Towards
the Year 2000 and Beyond (Cat. No. 98CH36286),
volume 4, pages 1977–1979. IEEE.
HEALTHINF 2020 - 13th International Conference on Health Informatics
600