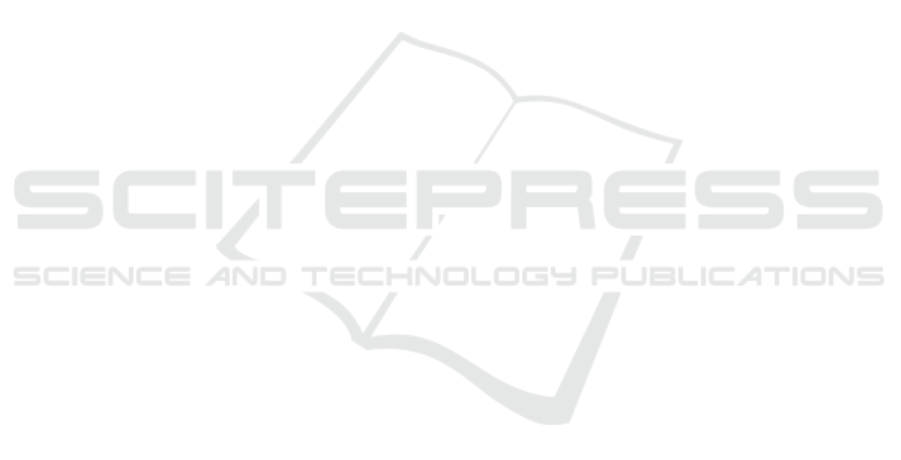
are shown in Figure 8 for each subtype. It is apparent
that both, mse and r models have failed to learn some
of the subtypes. Nevertheless, the mse model was able
to distinguish more classes in both color spaces com-
pared to the r model. The mse model also presents a
more balanced performance in terms of precision and
recall.
Low classification precision of negative samples
in r models indicates that many positive samples have
been rejected during classification and assigned to the
rejection class. This is, again, explained by the clas-
sifier bias. Furthermore, this assumption is confirmed
by the high recall score of the rejection class, mean-
ing that most of true negative samples have been clas-
sified correctly and low precision is a result of erro-
neously rejected positive samples.
Note that supertype classification still holds a high
overall performance, even when some subtypes have
not been recognized. This means that samples in
those subtypes have been assigned to a sibling sub-
type, contributing to a better supertype classification
overall. It is worth noting, that only supertypes with
just one subtype have not been recognized. Poor
classification performance also correlates with low
amount of samples available for each class.
6 CONCLUSION
It is evident that the Swiss traditional costume dataset
is desperately small, but this is also the rationale for
this work. We use poselets, similar to (Chen et al.,
2012), to define reproducible features that cannot be
located visually. We also propose to compute descrip-
tors for features by iteratively merging sample im-
ages of these features, while allowing for displace-
ment during pair-wise comparisons. We demonstrate
that the F
0.5
-score of mse-models computed with dis-
placement increases by 0.07-0.12 on the test set, com-
pared to F
0.5
-score without displacement. This model
performs best in L*a*b* color space on both, subtype
and supertype costume classification.
REFERENCES
Cao, Z., Hidalgo, G., Simon, T., Wei, S.-E., and Sheikh,
Y. (2018). OpenPose: realtime multi-person 2D pose
estimation using Part Affinity Fields. In arXiv preprint
arXiv:1812.08008.
Cao, Z., Simon, T., Wei, S.-E., and Sheikh, Y. (2017). Real-
time multi-person 2d pose estimation using part affin-
ity fields. In CVPR.
Chen, H., Gallagher, A., and Girod, B. (2012). Describ-
ing clothing by semantic attributes. In Fitzgibbon, A.,
Lazebnik, S., Perona, P., Sato, Y., and Schmid, C., ed-
itors, Computer Vision – ECCV 2012, pages 609–623,
Berlin, Heidelberg. Springer Berlin Heidelberg.
Eichner, M., Marin-Jimenez, M., Zisserman, A., and Fer-
rari, V. (2012). 2d articulated human pose estimation
and retrieval in (almost) unconstrained still images.
International journal of computer vision, 99(2):190–
214.
Kalantidis, Y., Kennedy, L., and Li, L.-J. (2013). Getting
the look: clothing recognition and segmentation for
automatic product suggestions in everyday photos. In
Proceedings of the 3rd ACM conference on Interna-
tional conference on multimedia retrieval , pages 105–
112. ACM.
Liu, Z., Luo, P., Qiu, S., Wang, X., and Tang, X. (2016).
Deepfashion: Powering robust clothes recognition and
retrieval with rich annotations. In Proceedings of
the IEEE conference on computer vision and pattern
recognition, pages 1096–1104.
Lowe, D. G. (1999). Object recognition from local scale-
invariant features. In Computer vision, 1999. The pro-
ceedings of the seventh IEEE international conference
on, volume 2, pages 1150–1157. Ieee.
Varma, M. and Zisserman, A. (2005). A statistical approach
to texture classification from single images. Interna-
tional journal of computer vision, 62(1-2):61–81.
Wei, S.-E., Ramakrishna, V., Kanade, T., and Sheikh, Y.
(2016). Convolutional pose machines. In CVPR.
Yamaguchi, K., Kiapour, M. H., Ortiz, L. E., and Berg,
T. L. (2012). Parsing clothing in fashion photographs.
In Computer Vision and Pattern Recognition (CVPR),
2012 IEEE Conference on, pages 3570–3577. IEEE.
VISAPP 2020 - 15th International Conference on Computer Vision Theory and Applications
420