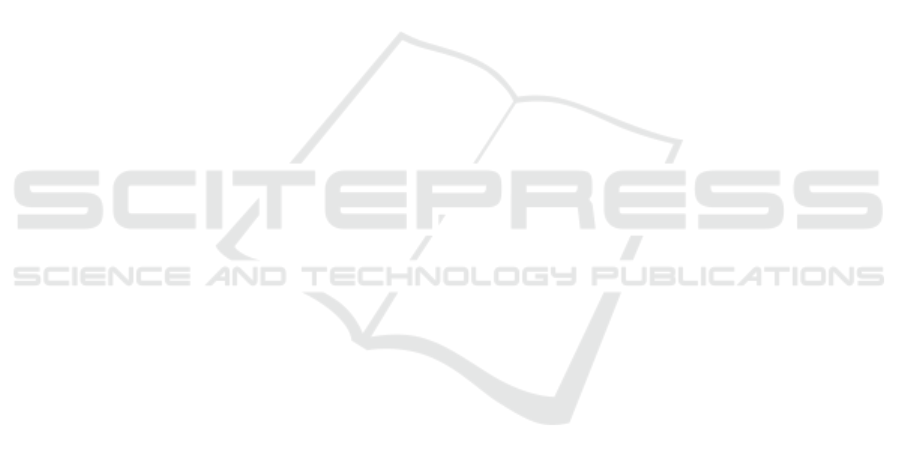
REFERENCES
Bonsu, K. O., Arunmanakul, P., and Chaiyakunapruk, N.
(2018). Pharmacological treatments for heart fail-
ure with preserved ejection fraction—a systematic re-
view and indirect comparison. Heart failure reviews,
23(2):147–156.
Breiman, L. (2001). Random forests. Machine learning,
45(1):5–32.
Bui, A. L., Horwich, T. B., and Fonarow, G. C. (2011). Epi-
demiology and risk profile of heart failure. Nature
Reviews Cardiology, 8(1):30.
Carvalho, B. S. and Irizarry, R. A. (2010). A framework
for oligonucleotide microarray preprocessing. Bioin-
formatics, 26(19):2363–2367.
Cortes, C. and Vapnik, V. (1995). Support-vector networks.
Machine learning, 20(3):273–297.
Cui, X. and Churchill, G. A. (2003). Statistical tests for dif-
ferential expression in cdna microarray experiments.
Genome biology, 4(4):210.
Dokainish, H., Teo, K., Zhu, J., Roy, A., Al-Habib, K., El-
Sayed, A., Palileo, L., Jaramillo, P. L., Karaye, K., Yu-
soff, K., et al. (2015). Heart failure in low-and middle-
income countries: background, rationale, and de-
sign of the international congestive heart failure study
(inter-chf). American heart journal, 170(4):627–634.
Dunlay, S. M., Roger, V. L., and Redfield, M. M. (2017).
Epidemiology of heart failure with preserved ejection
fraction. Nature Reviews Cardiology, 14(10):591.
Farmakis, D., Parissis, J., Lekakis, J., and Filippatos, G.
(2015). Acute heart failure: epidemiology, risk fac-
tors, and prevention. Revista Espa
˜
nola de Cardiolog
´
ıa
(English Edition), 68(3):245–248.
Ferrari, R., B
¨
ohm, M., Cleland, J. G., Paulus, W. J., Pieske,
B., Rapezzi, C., and Tavazzi, L. (2015). Heart fail-
ure with preserved ejection fraction: uncertainties
and dilemmas. European journal of heart failure,
17(7):665–671.
Gaggin, H. K. and Januzzi Jr, J. L. (2013). Biomark-
ers and diagnostics in heart failure. Biochimica et
Biophysica Acta (BBA)-Molecular Basis of Disease,
1832(12):2442–2450.
Gentleman, R. C., Carey, V. J., Bates, D. M., Bolstad, B.,
Dettling, M., Dudoit, S., Ellis, B., Gautier, L., Ge, Y.,
Gentry, J., et al. (2004). Bioconductor: open software
development for computational biology and bioinfor-
matics. Genome biology, 5(10):R80.
Haury, A.-C., Gestraud, P., and Vert, J.-P. (2011). The influ-
ence of feature selection methods on accuracy, stabil-
ity and interpretability of molecular signatures. PloS
one, 6(12):e28210.
Irizarry, R. A., Hobbs, B., Collin, F., Beazer-Barclay, Y. D.,
Antonellis, K. J., Scherf, U., and Speed, T. P. (2003).
Exploration, normalization, and summaries of high
density oligonucleotide array probe level data. Bio-
statistics, 4(2):249–264.
Jacobs, L., Thijs, L., Jin, Y., Zannad, F., Mebazaa, A.,
Rouet, P., Pinet, F., Bauters, C., Pieske, B., Tomas-
chitz, A., et al. (2014). Heart ‘omics’ in ageing
(homage): design, research objectives and character-
istics of the common database. Journal of biomedical
research, 28(5):349.
James, P. T. (2004). Obesity: the worldwide epidemic. Clin-
ics in dermatology, 22(4):276–280.
Kuhn, M. et al. (2008). Building predictive models in r us-
ing the caret package. Journal of statistical software,
28(5):1–26.
Kumar Sarmah, C. and Samarasinghe, S. (2010). Microar-
ray data integration: frameworks and a list of underly-
ing issues. Current Bioinformatics, 5(4):280–289.
Lafita, A., Bliven, S., Prli
´
c, A., Guzenko, D., Rose, P. W.,
Bradley, A., Pavan, P., Myers-Turnbull, D., Valasa-
tava, Y., Heuer, M., et al. (2019). Biojava 5: A
community driven open-source bioinformatics library.
PLoS computational biology, 15(2):e1006791.
Lazar, C., Meganck, S., Taminau, J., Steenhoff, D., Coletta,
A., Molter, C., Weiss-Sol
´
ıs, D. Y., Duque, R., Bersini,
H., and Now
´
e, A. (2012). Batch effect removal meth-
ods for microarray gene expression data integration: a
survey. Briefings in bioinformatics, 14(4):469–490.
Leek, J. T., Scharpf, R. B., Bravo, H. C., Simcha, D., Lang-
mead, B., Johnson, W. E., Geman, D., Baggerly, K.,
and Irizarry, R. A. (2010). Tackling the widespread
and critical impact of batch effects in high-throughput
data. Nature Reviews Genetics, 11(10):733.
Lourenco, A. P., Leite-Moreira, A. F., Balligand, J.-L.,
Bauersachs, J., Dawson, D., de Boer, R. A., de Windt,
L. J., Falc
˜
ao-Pires, I., Fontes-Carvalho, R., Franz, S.,
et al. (2018). An integrative translational approach to
study heart failure with preserved ejection fraction: a
position paper from the working group on myocardial
function of the european society of cardiology. Euro-
pean journal of heart failure, 20(2):216–227.
Nygaard, V., Rødland, E. A., and Hovig, E. (2016). Meth-
ods that remove batch effects while retaining group
differences may lead to exaggerated confidence in
downstream analyses. Biostatistics, 17(1):29–39.
Paulus, W. J., Tsch
¨
ope, C., Sanderson, J. E., Rusconi,
C., Flachskampf, F. A., Rademakers, F. E., Marino,
P., Smiseth, O. A., De Keulenaer, G., Leite-Moreira,
A. F., et al. (2007). How to diagnose diastolic heart
failure: a consensus statement on the diagnosis of
heart failure with normal left ventricular ejection frac-
tion by the heart failure and echocardiography asso-
ciations of the european society of cardiology. Euro-
pean heart journal, 28(20):2539–2550.
Persson, H., Lonn, E., Edner, M., Baruch, L., Lang, C. C.,
Morton, J. J.,
¨
Ostergren, J., McKelvie, R. S., et al.
(2007). Diastolic dysfunction in heart failure with pre-
served systolic function: need for objective evidence:
results from the charm echocardiographic substudy–
charmes. Journal of the American College of Cardiol-
ogy, 49(6):687–694.
Ritchie, M. E., Phipson, B., Wu, D., Hu, Y., Law, C. W., Shi,
W., and Smyth, G. K. (2015). limma powers differ-
ential expression analyses for rna-sequencing and mi-
croarray studies. Nucleic acids research, 43(7):e47–
e47.
Savarese, G. and Lund, L. H. (2017). Global public health
burden of heart failure. Cardiac failure review, 3(1):7.
A Computational Platform for Heart Failure Cases Research
607